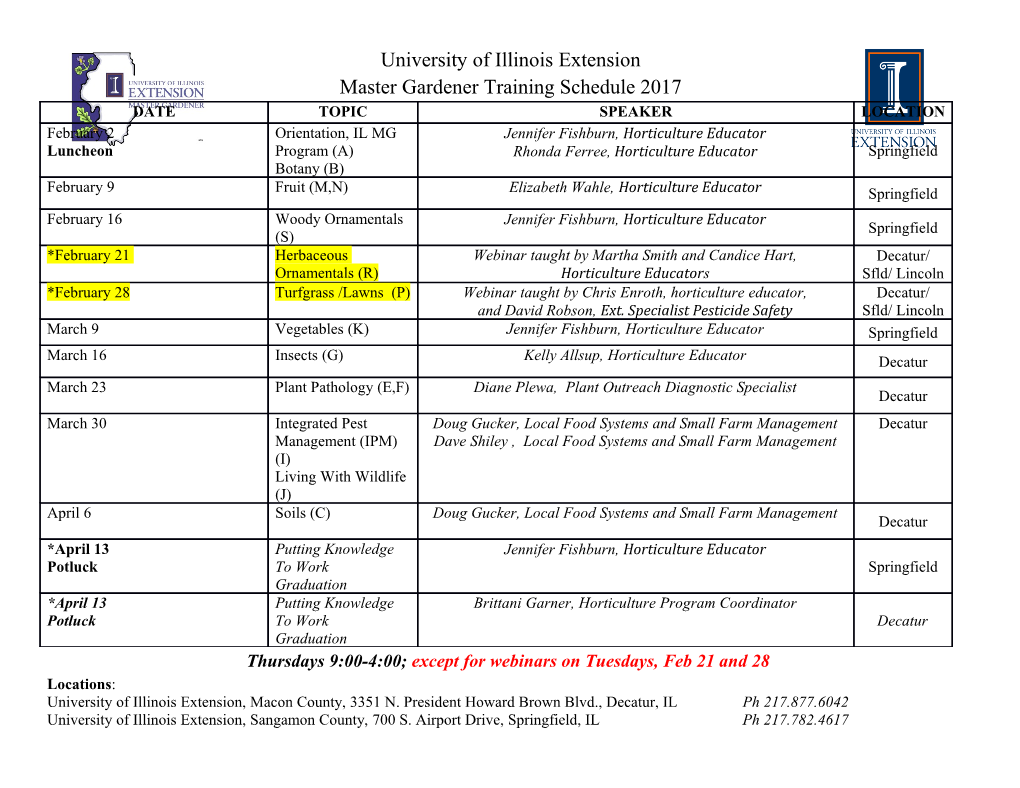
Maximum Margin Reward Networks for Learning from Explicit and Implicit Supervision Haoruo Peng1 Ming-Wei Chang2 Wen-tau Yih2 1University of Illinois, Urbana-Champaign 2Microsoft Research, Redmond [email protected] 2 minchang,scottyih @microsoft.com { } Abstract Q: Who played Meg in Season 1 of Family Guy? 휆푥. ∃푦. 푐푎푠푡 FamilyGuySeason1, 푦 ∧ 푎푐푡표푟 푦, 푥 Neural networks have achieved state-of- the-art performance on several structured- KB Lacey Chabert, Seth MacFarlane, Alex Borstein, output prediction tasks, trained in a fully Seth Green, John Viener, Alec Sulkin supervised fashion. However, annotated examples in structured domains are of- ten costly to obtain, which thus limits A: Lacey Chabert the applications of neural networks. In Figure 1: Learning a semantic parser using im- this work, we propose Maximum Mar- plicit supervision signals (labeled answers). Since gin Reward Networks, a neural network- there are no gold parses, a model needs to explore based framework that aims to learn from different parses, where their quality can only be both explicit (full structures) and implicit indirectly verified by comparing retrieved answers supervision signals (delayed feedback on and the labeled answers. the correctness of the predicted structure). On named entity recognition and seman- tic parsing, our model outperforms previ- comparing the derived answers from the knowl- ous systems on the benchmark datasets, edge base and the provided labeled answers. CoNLL-2003 and WebQuestionsSP. This setting of implicit supervision increases the difficulty of learning a neural model, not only 1 Introduction because the signals are vague and noisy, but also Structured-output prediction problems, where the delayed. For instance, among different semantic goal is to determine values of a set of inter- parses that result in the same answers, typically dependent variables, are ubiquitous in NLP. Struc- only few of them correctly represent the meaning tures of such problems can range from simple se- of the question. Moreover, the correctness of an- quences like part-of-speech tagging (Ling et al., swers corresponding to a parse can only be eval- 2015) and named entity recognition (Lample et al., uated through an external oracle (e.g., executing 2016), to complex syntactic or semantic analysis the query on the knowledge base) after the parse such as dependency parsing (Dyer et al., 2015) and is fully constructed. Early model update before the semantic parsing (Dong and Lapata, 2016). State- search of a full semantic parse is complete is gen- of-the-art methods of these tasks are often neu- erally infeasible.1 It is also not clear how to lever- ral network models trained using fully annotated age implicit and explicit signals integrally during structures, which can be costly or time-consuming learning when both kinds of labels are present. to obtain. Weakly supervised learning settings, In this work, we propose Maximum Margin Re- where the algorithm assumes only the existence of ward Networks (MMRN), which is a general neu- implicit signals on whether a prediction is correct, ral network-based framework that is able to learn are thus more appealing in many scenarios. from both implicit and explicit supervision sig- For example, Figure 1 shows a weakly super- nals. By casting structured-output learning as a vised setting of learning semantic parsers using search problem, the key insight in MMRN is the only question–answer pairs. When the system 1Existing weakly supervised methods (Clarke et al., 2010; generates a candidate semantic parse during train- Artzi and Zettlemoyer, 2013) often leverage domain-specific ing, the quality needs to be indirectly measured by heuristics, which are not always available. 2368 Proceedings of the 2017 Conference on Empirical Methods in Natural Language Processing, pages 2368–2378 Copenhagen, Denmark, September 7–11, 2017. c 2017 Association for Computational Linguistics special mechanism of rewards. Rewards can be posed (Daume´ and Marcu, 2005; Daume´ et al., viewed as the training signals that drive the model 2009), which casts the structured prediction task to explore the search space and to find the cor- as a general search problem. Most recently, rect structure. The explicit supervision signals can recurrent neural networks such as LSTM mod- be viewed as a source of immediate rewards, as els (Hochreiter and Schmidhuber, 1997) have been we can often instantly know the correctness of the used as a general tool for structured output mod- current action. On the other hand, the implicit su- els (Vinyals et al., 2015). pervision can be viewed as a source of delayed re- Latent structured learning algorithms address wards, where the reward of the actions can only be the problem of learning from incomplete labeled revealed later. We unify these two types of reward data (Yu and Joachims, 2009; Quattoni et al., signals by using a maximum margin update, in- 2007). The main difference compared to our spired by structured SVM (Joachims et al., 2009). framework is the existence of the external envi- The effectiveness of MMRN is demonstrated on ronment when learning from implicit signals. three NLP tasks: named entity recognition, entity Upadhyay et al. (2016) first proposed the idea of linking and semantic parsing. MMRN outperforms learning from implicit supervision, and is the most the current best results on CoNLL-2003 named related paper to our work. Compared to their lin- entity recognition dataset (Tjong Kim Sang and ear algorithm, our framework is more principled De Meulder, 2003), reaching 91.4% F1, in the and general as we integrate the concept of margin close setting where no gazetteer is allowed. It also in our method. Furthermore, we also extend the performs comparably to the existing state-of-the- framework using neural models. art systems on entity linking. Models for these two tasks are trained using explicit supervision. 3 Search-based Inference For semantic parsing, where only implicit super- vision signals are provided, MMRN is able to learn In our framework, predicting the best structured from delayed rewards, improving the entity link- output, inference, is formulated as a state/action ing component and the overall semantic parsing search problem. Our search space can be de- framework jointly, and outperforms the best pub- scribed as follows. The initial state, s0, is the lished system by 1.4% absolute on the WebQSP starting point of the search process. We define dataset (Yih et al., 2016). γ(s) as the set of all feasible actions that can In the rest of the paper, we survey the most be taken at s, and denote s0 = τ(s, a) as the related work in Sec. 2 and give an in-depth dis- transition function, where s0 is the new state af- cussion on comparing MMRN and other learning ter taking action a from s. A path h is a se- frameworks in Sec. 7. We start the description of quence of state–action pairs, starting with the ini- tial state: h = (s , a ),..., (s , a ) , where our method from the search formulation and the { 0 0 k k } state–action spaces in our targeted tasks in Sec. 3, si = τ(si 1, ai 1), i = 1, . , k. We denote ; − − ∀ followed by the reward and learning algorithm in h sˆ, if sˆ = τ(sk, ak), the final state which the Sec. 4 and the detailed neural model design in path h leads to. A path essentially is a partial or Sec. 5. Sec. 6 reports the experimental results and complete structured prediction. For each input x, we define (x) to be the set of all possible paths Sec. 8 concludes the paper. H for the input. We also define (x) = h h E { | ∈ (x), h ; s,ˆ γ(ˆs) = , which is all possible 2 Related Work H ∅} paths that lead to terminal states. Structured output prediction tasks have been stud- Given a state s and an action a, the scoring func- ied extensively in the field of natural language pro- tion fθ(s, a) measures the quality of an immediate cessing (NLP). Many supervised structured learn- action with respect to the current state, where θ is ing algorithms has been proposed for capturing the model parameters. The score of a path h is the relationships between output variables. These defined as the sum of the scores for state-action k models include structured perceptron (Collins, pairs in h: fθ(h) = i=0 fθ(si, ai). During test 2002; Collins and Roark, 2004), conditional ran- time, inference is to find the best path in (x): P E dom fields (Lafferty et al., 2001), and structured arg maxh (x) fθ(h; x). In practice, inference is SVM (Taskar et al., 2004; Joachims et al., 2009). often approximated∈E by beam search when no effi- Later, the learning to search framework is pro- cient algorithm exists. 2369 In the remaining of this section, we describe 휆푥. ∃푦. 푐푎푠푡 FamilyGuySeason1, 푦 ∧ 푎푐푡표푟 푦, 푥 the states and actions in the targeted tasks in this ∧ 푐ℎ푎푟푎푐푡푒푟(푦,MegGriffin) work: named entity recognition, entity linking and Meg Griffin semantic parsing. The the model and learning al- gorithm will be discussed in Sec. 4 and Sec. 5. Family Guy Season 1 cast y actor x 3.1 Named entity recognition Figure 2: Semantic parses in λ-calculus (top) and The task of named entity recognition (NER) is to query graph (bottom) of the question “who played identify entity mentions in a sentence, as well as meg in season 1 of family guy?” to assign their types, such as Person or Location. Following the conventional setting, we treat it as a sequence labeling problem using the standard 3.3 Semantic parsing BIOES encoding. For instance, a “B-LOC” tag Our third targeted task is semantic parsing (SP), on a word means that the word is the beginning of which is a task of mapping a text utterance to a for- a multi-word location entity.
Details
-
File Typepdf
-
Upload Time-
-
Content LanguagesEnglish
-
Upload UserAnonymous/Not logged-in
-
File Pages11 Page
-
File Size-