Parallel Exact Linear Algebra Ingredients for the Parallelization Parallel Dense Linear Algebra Mod P
Total Page:16
File Type:pdf, Size:1020Kb
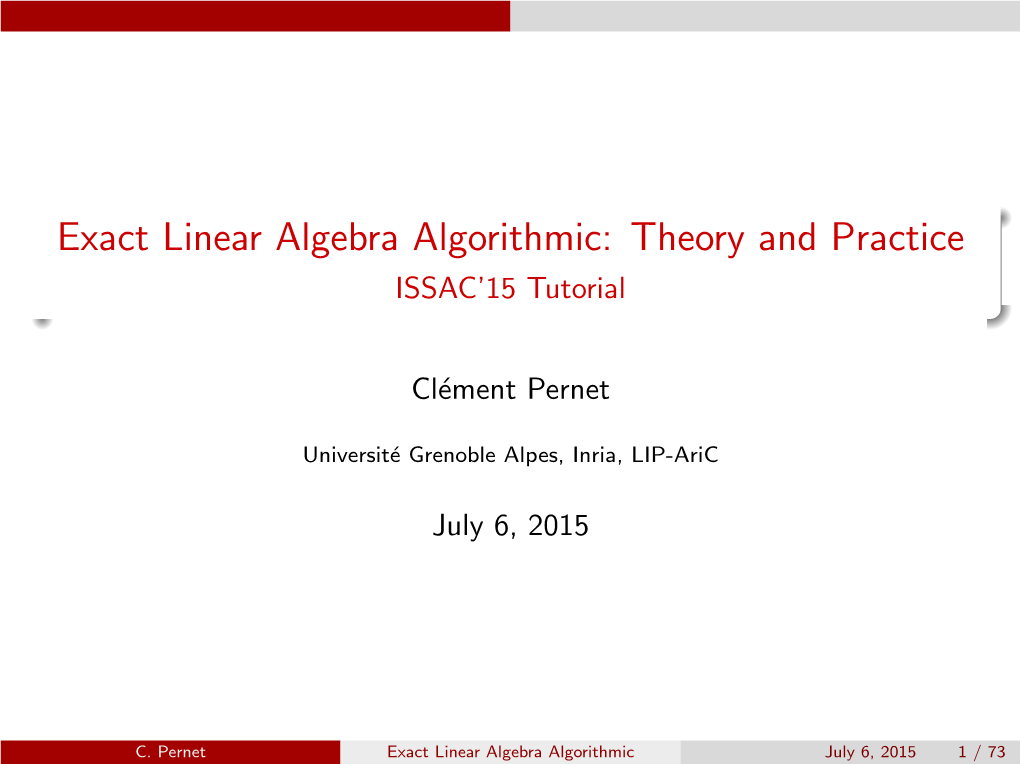
Load more
Recommended publications
-
Recursive Approach in Sparse Matrix LU Factorization
51 Recursive approach in sparse matrix LU factorization Jack Dongarra, Victor Eijkhout and the resulting matrix is often guaranteed to be positive Piotr Łuszczek∗ definite or close to it. However, when the linear sys- University of Tennessee, Department of Computer tem matrix is strongly unsymmetric or indefinite, as Science, Knoxville, TN 37996-3450, USA is the case with matrices originating from systems of Tel.: +865 974 8295; Fax: +865 974 8296 ordinary differential equations or the indefinite matri- ces arising from shift-invert techniques in eigenvalue methods, one has to revert to direct methods which are This paper describes a recursive method for the LU factoriza- the focus of this paper. tion of sparse matrices. The recursive formulation of com- In direct methods, Gaussian elimination with partial mon linear algebra codes has been proven very successful in pivoting is performed to find a solution of Eq. (1). Most dense matrix computations. An extension of the recursive commonly, the factored form of A is given by means technique for sparse matrices is presented. Performance re- L U P Q sults given here show that the recursive approach may per- of matrices , , and such that: form comparable to leading software packages for sparse ma- LU = PAQ, (2) trix factorization in terms of execution time, memory usage, and error estimates of the solution. where: – L is a lower triangular matrix with unitary diago- nal, 1. Introduction – U is an upper triangular matrix with arbitrary di- agonal, Typically, a system of linear equations has the form: – P and Q are row and column permutation matri- Ax = b, (1) ces, respectively (each row and column of these matrices contains single a non-zero entry which is A n n A ∈ n×n x where is by real matrix ( R ), and 1, and the following holds: PPT = QQT = I, b n b, x ∈ n and are -dimensional real vectors ( R ). -
Lecture 5 - Triangular Factorizations & Operation Counts
Lecture 5 - Triangular Factorizations & Operation Counts LU Factorization We have seen that the process of GE essentially factors a matrix A into LU. Now we want to see how this factorization allows us to solve linear systems and why in many cases it is the preferred algorithm compared with GE. Remember on paper, these methods are the same but computationally they can be different. First, suppose we want to solve A~x = ~b and we are given the factorization A = LU. It turns out that the system LU~x = ~b is \easy" to solve because we do a forward solve L~y = ~b and then back solve U~x = ~y. We have seen that we can easily implement the equations for the back solve and for homework you will write out the equations for the forward solve. Example If 0 2 −1 2 1 0 1 0 0 1 0 2 −1 2 1 A = @ 4 1 9 A = LU = @ 2 1 0 A @ 0 3 5 A 8 5 24 4 3 1 0 0 1 solve the linear system A~x = ~b where ~b = (0; −5; −16)T . ~ We first solve L~y = b to get y1 = 0, 2y1 +y2 = −5 implies y2 = −5 and 4y1 +3y2 +y3 = −16 T implies y3 = −1. Now we solve U~x = ~y = (0; −5; −1) . Back solving yields x3 = −1, 3x2 + 5x3 = −5 implies x2 = 0 and finally 2x1 − x2 + 2x3 = 0 implies x1 = 1 giving the solution (1; 0; −1)T . If GE and LU factorization are equivalent on paper, why would one be computationally advantageous in some settings? Recall that when we solve A~x = ~b by GE we must also multiply the right hand side by the Gauss transformation matrices. -
LU Factorization LU Decomposition LU Decomposition: Motivation LU Decomposition
LU Factorization LU Decomposition To further improve the efficiency of solving linear systems Factorizations of matrix A : LU and QR A matrix A can be decomposed into a lower triangular matrix L and upper triangular matrix U so that LU Factorization Methods: Using basic Gaussian Elimination (GE) A = LU ◦ Factorization of Tridiagonal Matrix ◦ LU decomposition is performed once; can be used to solve multiple Using Gaussian Elimination with pivoting ◦ right hand sides. Direct LU Factorization ◦ Similar to Gaussian elimination, care must be taken to avoid roundoff Factorizing Symmetrix Matrices (Cholesky Decomposition) ◦ errors (partial or full pivoting) Applications Special Cases: Banded matrices, Symmetric matrices. Analysis ITCS 4133/5133: Intro. to Numerical Methods 1 LU/QR Factorization ITCS 4133/5133: Intro. to Numerical Methods 2 LU/QR Factorization LU Decomposition: Motivation LU Decomposition Forward pass of Gaussian elimination results in the upper triangular ma- trix 1 u12 u13 u1n 0 1 u ··· u 23 ··· 2n U = 0 0 1 u3n . ···. 0 0 0 1 ··· We can determine a matrix L such that LU = A , and l11 0 0 0 l l 0 ··· 0 21 22 ··· L = l31 l32 l33 0 . ···. . l l l 1 n1 n2 n3 ··· ITCS 4133/5133: Intro. to Numerical Methods 3 LU/QR Factorization ITCS 4133/5133: Intro. to Numerical Methods 4 LU/QR Factorization LU Decomposition via Basic Gaussian Elimination LU Decomposition via Basic Gaussian Elimination: Algorithm ITCS 4133/5133: Intro. to Numerical Methods 5 LU/QR Factorization ITCS 4133/5133: Intro. to Numerical Methods 6 LU/QR Factorization LU Decomposition of Tridiagonal Matrix Banded Matrices Matrices that have non-zero elements close to the main diagonal Example: matrix with a bandwidth of 3 (or half band of 1) a11 a12 0 0 0 a21 a22 a23 0 0 0 a32 a33 a34 0 0 0 a43 a44 a45 0 0 0 a a 54 55 Efficiency: Reduced pivoting needed, as elements below bands are zero. -
LU-Factorization 1 Introduction 2 Upper and Lower Triangular Matrices
MAT067 University of California, Davis Winter 2007 LU-Factorization Isaiah Lankham, Bruno Nachtergaele, Anne Schilling (March 12, 2007) 1 Introduction Given a system of linear equations, a complete reduction of the coefficient matrix to Reduced Row Echelon (RRE) form is far from the most efficient algorithm if one is only interested in finding a solution to the system. However, the Elementary Row Operations (EROs) that constitute such a reduction are themselves at the heart of many frequently used numerical (i.e., computer-calculated) applications of Linear Algebra. In the Sections that follow, we will see how EROs can be used to produce a so-called LU-factorization of a matrix into a product of two significantly simpler matrices. Unlike Diagonalization and the Polar Decomposition for Matrices that we’ve already encountered in this course, these LU Decompositions can be computed reasonably quickly for many matrices. LU-factorizations are also an important tool for solving linear systems of equations. You should note that the factorization of complicated objects into simpler components is an extremely common problem solving technique in mathematics. E.g., we will often factor a polynomial into several polynomials of lower degree, and one can similarly use the prime factorization for an integer in order to simply certain numerical computations. 2 Upper and Lower Triangular Matrices We begin by recalling the terminology for several special forms of matrices. n×n A square matrix A =(aij) ∈ F is called upper triangular if aij = 0 for each pair of integers i, j ∈{1,...,n} such that i>j. In other words, A has the form a11 a12 a13 ··· a1n 0 a22 a23 ··· a2n A = 00a33 ··· a3n . -
On Finding Multiplicities of Characteristic Polynomial Factors Of
On finding multiplicities of characteristic polynomial factors of black-box matrices∗. Jean-Guillaume Dumas† Cl´ement Pernet‡ B. David Saunders§ November 2, 2018 Abstract We present algorithms and heuristics to compute the characteristic polynomial of a matrix given its minimal polynomial. The matrix is rep- resented as a black-box, i.e., by a function to compute its matrix-vector product. The methods apply to matrices either over the integers or over a large enough finite field. Experiments show that these methods perform efficiently in practice. Combined in an adaptive strategy, these algorithms reach significant speedups in practice for some integer matrices arising in an application from graph theory. Keywords: Characteristic polynomial ; black-box matrix ; finite field. 1 Introduction Computing the characteristic polynomial of an integer matrix is a classical math- ematical problem. It is closely related to the computation of the Frobenius nor- mal form which can be used to test two matrices for similarity, or computing invariant subspaces under the action of the matrix. Although the Frobenius normal form contains more information on the matrix than the characteristic arXiv:0901.4747v2 [cs.SC] 18 May 2009 polynomial, most algorithms to compute it are based on computations of char- acteristic polynomials (see for example [25, 9.7]). Several matrix representations are used in§ computational linear algebra. In the dense representation, a m n matrix is considered as the array of all the m n coefficients. The sparse representation× only considers non-zero coefficients using× different possible data structures. In the black-box representation, the matrix ∗Saunders supported by National Science Foundation Grants CCF-0515197, CCF-0830130. -
LU, QR and Cholesky Factorizations Using Vector Capabilities of Gpus LAPACK Working Note 202 Vasily Volkov James W
LU, QR and Cholesky Factorizations using Vector Capabilities of GPUs LAPACK Working Note 202 Vasily Volkov James W. Demmel Computer Science Division Computer Science Division and Department of Mathematics University of California at Berkeley University of California at Berkeley Abstract GeForce Quadro GeForce GeForce GPU name We present performance results for dense linear algebra using 8800GTX FX5600 8800GTS 8600GTS the 8-series NVIDIA GPUs. Our matrix-matrix multiply routine # of SIMD cores 16 16 12 4 (GEMM) runs 60% faster than the vendor implementation in CUBLAS 1.1 and approaches the peak of hardware capabilities. core clock, GHz 1.35 1.35 1.188 1.458 Our LU, QR and Cholesky factorizations achieve up to 80–90% peak Gflop/s 346 346 228 93.3 of the peak GEMM rate. Our parallel LU running on two GPUs peak Gflop/s/core 21.6 21.6 19.0 23.3 achieves up to ~300 Gflop/s. These results are accomplished by memory bus, MHz 900 800 800 1000 challenging the accepted view of the GPU architecture and memory bus, pins 384 384 320 128 programming guidelines. We argue that modern GPUs should be viewed as multithreaded multicore vector units. We exploit bandwidth, GB/s 86 77 64 32 blocking similarly to vector computers and heterogeneity of the memory size, MB 768 1535 640 256 system by computing both on GPU and CPU. This study flops:word 16 18 14 12 includes detailed benchmarking of the GPU memory system that Table 1: The list of the GPUs used in this study. Flops:word is the reveals sizes and latencies of caches and TLB. -
LU and Cholesky Decompositions. J. Demmel, Chapter 2.7
LU and Cholesky decompositions. J. Demmel, Chapter 2.7 1/14 Gaussian Elimination The Algorithm — Overview Solving Ax = b using Gaussian elimination. 1 Factorize A into A = PLU Permutation Unit lower triangular Non-singular upper triangular 2/14 Gaussian Elimination The Algorithm — Overview Solving Ax = b using Gaussian elimination. 1 Factorize A into A = PLU Permutation Unit lower triangular Non-singular upper triangular 2 Solve PLUx = b (for LUx) : − LUx = P 1b 3/14 Gaussian Elimination The Algorithm — Overview Solving Ax = b using Gaussian elimination. 1 Factorize A into A = PLU Permutation Unit lower triangular Non-singular upper triangular 2 Solve PLUx = b (for LUx) : − LUx = P 1b − 3 Solve LUx = P 1b (for Ux) by forward substitution: − − Ux = L 1(P 1b). 4/14 Gaussian Elimination The Algorithm — Overview Solving Ax = b using Gaussian elimination. 1 Factorize A into A = PLU Permutation Unit lower triangular Non-singular upper triangular 2 Solve PLUx = b (for LUx) : − LUx = P 1b − 3 Solve LUx = P 1b (for Ux) by forward substitution: − − Ux = L 1(P 1b). − − 4 Solve Ux = L 1(P 1b) by backward substitution: − − − x = U 1(L 1P 1b). 5/14 Example of LU factorization We factorize the following 2-by-2 matrix: 4 3 l 0 u u = 11 11 12 . (1) 6 3 l21 l22 0 u22 One way to find the LU decomposition of this simple matrix would be to simply solve the linear equations by inspection. Expanding the matrix multiplication gives l11 · u11 + 0 · 0 = 4, l11 · u12 + 0 · u22 = 3, (2) l21 · u11 + l22 · 0 = 6, l21 · u12 + l22 · u22 = 3. -
Math 2270 - Lecture 27 : Calculating Determinants
Math 2270 - Lecture 27 : Calculating Determinants Dylan Zwick Fall 2012 This lecture covers section 5.2 from the textbook. In the last lecture we stated and discovered a number of properties about determinants. However, we didn’t talk much about how to calculate them. In fact, the only general formula was the nasty formula mentioned at the beginning, namely n det(A)= sgn(σ) a . X Y iσ(i) σ∈Sn i=1 We also learned a formula for calculating the determinant in a very special case. Namely, if we have a triangular matrix, the determinant is just the product of the diagonals. Today, we’re going to discuss how that special triangular case can be used to calculate determinants in a very efficient manner, and we’ll derive the nasty formula. We’ll also go over one other way of calculating a deter- minnat, the “cofactor expansion”, that, to be honest, is not all that useful computationally, but can be very useful when you need to prove things. The assigned problems for this section are: Section 5.2 - 1, 3, 11, 15, 16 1 1 Calculating the Determinant from the Pivots In practice, the easiest way to calculate the determinant of a general matrix is to use elimination to get an upper-triangular matrix with the same de- terminant, and then just calculate the determinant of the upper-triangular matrix by taking the product of the diagonal terms, a.k.a. the pivots. If there are no row exchanges required for the LU decomposition of A, then A = LU, and det(A)= det(L)det(U). -
LU Decomposition S = LU, Then We Can 1 0 0 2 4 −2 2 4 −2 Use It to Solve Sx = F
LU-Decomposition Example 1 Compare with Example 1 in gaussian elimination.pdf. Consider I The Gaussian elimination for the solution of the linear system Sx = f transforms the augmented matrix (Sjf) into (Ujc), where U 0 2 4 −21 is upper triangular. The transformations are determined by the S = 4 9 −3 : (1) matrix S only. @ A If we have to solve a system Sx = f~ with a different right hand side −2 −3 7 f~, then we start over. Most of the computations that lead us from We express Gaussian Elimination using Matrix-Matrix-multiplications (Sjf~) into (Ujc~), depend only on S and are identical to the steps that we executed when we applied Gaussian elimination to Sx = f. 0 1 0 0 1 0 2 4 −2 1 0 2 4 −2 1 We now express Gaussian elimination as a sequence of matrix-matrix I @ −2 1 0 A @ 4 9 −3 A = @ 0 1 1 A multiplications. This representation leads to the decomposition of S 1 0 1 −2 −3 7 0 1 5 into a product of a lower triangular matrix L and an upper triangular | {z } | {z } | {z } matrix U, S = LU. This is known as the LU-Decomposition of S. =E1 =S =E1S I If we have computed the LU decomposition S = LU, then we can 0 1 0 0 1 0 2 4 −2 1 0 2 4 −2 1 use it to solve Sx = f. @ 0 1 0 A @ 0 1 1 A = @ 0 1 1 A I We replace S by LU, 0 −1 1 0 1 5 0 0 4 LUx = f; | {z } | {z } | {z } and introduce y = Ux. -
Scalable Sparse Symbolic LU Factorization on Gpus
JOURNAL OF LATEX CLASS FILES, VOL. 14, NO. 8, AUGUST 2015 1 GSOFA: Scalable Sparse Symbolic LU Factorization on GPUs Anil Gaihre, Xiaoye Sherry Li and Hang Liu Abstract—Decomposing a matrix A into a lower matrix L and an upper matrix U, which is also known as LU decomposition, is an essential operation in numerical linear algebra. For a sparse matrix, LU decomposition often introduces more nonzero entries in the L and U factors than in the original matrix. A symbolic factorization step is needed to identify the nonzero structures of L and U matrices. Attracted by the enormous potentials of the Graphics Processing Units (GPUs), an array of efforts have surged to deploy various LU factorization steps except for the symbolic factorization, to the best of our knowledge, on GPUs. This paper introduces GSOFA, the first GPU-based symbolic factorization design with the following three optimizations to enable scalable LU symbolic factorization for nonsymmetric pattern sparse matrices on GPUs. First, we introduce a novel fine-grained parallel symbolic factorization algorithm that is well suited for the Single Instruction Multiple Thread (SIMT) architecture of GPUs. Second, we tailor supernode detection into a SIMT friendly process and strive to balance the workload, minimize the communication and saturate the GPU computing resources during supernode detection. Third, we introduce a three-pronged optimization to reduce the excessive space consumption problem faced by multi-source concurrent symbolic factorization. Taken together, GSOFA achieves up to 31× speedup from 1 to 44 Summit nodes (6 to 264 GPUs) and outperforms the state-of-the-art CPU project, on average, by 5×. -
LU, QR and Cholesky Factorizations Using Vector Capabilities of Gpus LAPACK Working Note 202 Vasily Volkov James W
LU, QR and Cholesky Factorizations using Vector Capabilities of GPUs LAPACK Working Note 202 Vasily Volkov James W. Demmel Computer Science Division Computer Science Division and Department of Mathematics University of California at Berkeley University of California at Berkeley Abstract GeForce Quadro GeForce GeForce GPU name We present performance results for dense linear algebra using 8800GTX FX5600 8800GTS 8600GTS the 8-series NVIDIA GPUs. Our matrix-matrix multiply routine # of SIMD cores 16 16 12 4 (GEMM) runs 60% faster than the vendor implementation in CUBLAS 1.1 and approaches the peak of hardware capabilities. core clock, GHz 1.35 1.35 1.188 1.458 Our LU, QR and Cholesky factorizations achieve up to 80–90% peak Gflop/s 346 346 228 93.3 of the peak GEMM rate. Our parallel LU running on two GPUs peak Gflop/s/core 21.6 21.6 19.0 23.3 achieves up to ~300 Gflop/s. These results are accomplished by memory bus, MHz 900 800 800 1000 challenging the accepted view of the GPU architecture and memory bus, pins 384 384 320 128 programming guidelines. We argue that modern GPUs should be viewed as multithreaded multicore vector units. We exploit bandwidth, GB/s 86 77 64 32 blocking similarly to vector computers and heterogeneity of the memory size, MB 768 1535 640 256 system by computing both on GPU and CPU. This study flops:word 16 18 14 12 includes detailed benchmarking of the GPU memory system that Table 1: The list of the GPUs used in this study. Flops:word is the reveals sizes and latencies of caches and TLB. -
Observavility Brunovsky Normal Form: Multi-Output Linear Dynamical Systems Driss Boutat, Frédéric Kratz, Jean-Pierre Barbot
Observavility Brunovsky Normal Form: Multi-Output Linear Dynamical Systems Driss Boutat, Frédéric Kratz, Jean-Pierre Barbot To cite this version: Driss Boutat, Frédéric Kratz, Jean-Pierre Barbot. Observavility Brunovsky Normal Form: Multi- Output Linear Dynamical Systems. American Control Conference, ACC09, IEEE, Jun 2009, St. Louis, MO, United States. hal-00772281 HAL Id: hal-00772281 https://hal.inria.fr/hal-00772281 Submitted on 10 Jan 2013 HAL is a multi-disciplinary open access L’archive ouverte pluridisciplinaire HAL, est archive for the deposit and dissemination of sci- destinée au dépôt et à la diffusion de documents entific research documents, whether they are pub- scientifiques de niveau recherche, publiés ou non, lished or not. The documents may come from émanant des établissements d’enseignement et de teaching and research institutions in France or recherche français ou étrangers, des laboratoires abroad, or from public or private research centers. publics ou privés. Observavility Brunovsky Normal Form: Multi-Output Linear Dynamical Systems Driss Boutat, Fred´ eric´ Kratz and Jean-Pierre Barbot Abstract— This paper gives the sufficient and necessary II. NOTATION AND PROBLEM STATEMENT conditions to guarantee the existence of a linear change of co- ordinates to transform a multi-output linear dynamical system (modulo a nonlinear term depending on inputs and outputs) in the observability Brunovsky canonical form. Consider the following multi-output dynamical system: I. INTRODUCTION x˙ = Ax + γ(y, u) (1) y = Cx (2) For a single output dynamical linear system the observability rank condition is a necessary and sufficient condition to transform it into the Brunovsky observability where: normal form. In this last form, it is possible to use classical observer such that [8] observer and, [5] observer.