Clustering Data Streams
Total Page:16
File Type:pdf, Size:1020Kb
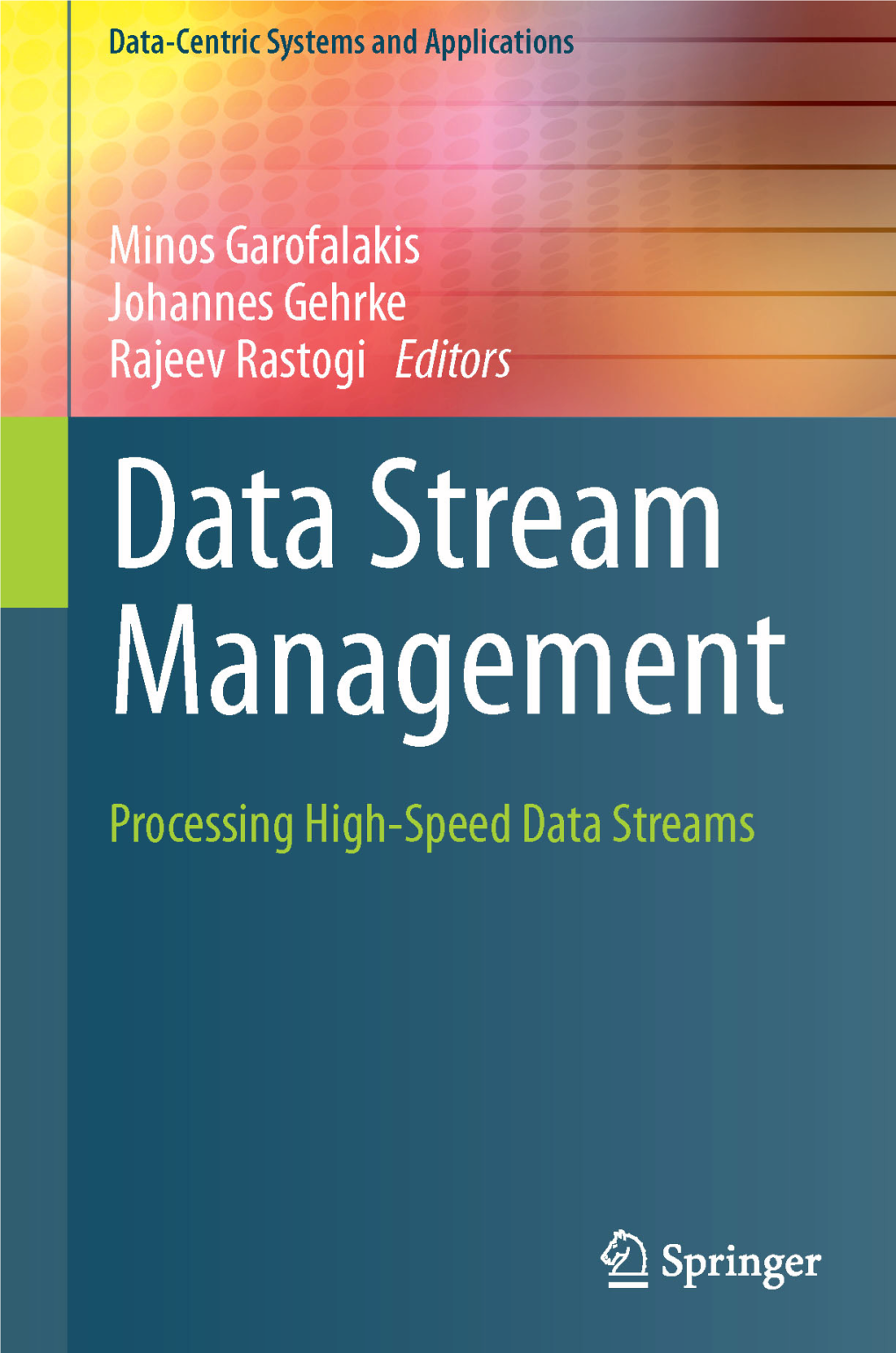
Load more
Recommended publications
-
FOCS 2005 Program SUNDAY October 23, 2005
FOCS 2005 Program SUNDAY October 23, 2005 Talks in Grand Ballroom, 17th floor Session 1: 8:50am – 10:10am Chair: Eva´ Tardos 8:50 Agnostically Learning Halfspaces Adam Kalai, Adam Klivans, Yishay Mansour and Rocco Servedio 9:10 Noise stability of functions with low influences: invari- ance and optimality The 46th Annual IEEE Symposium on Elchanan Mossel, Ryan O’Donnell and Krzysztof Foundations of Computer Science Oleszkiewicz October 22-25, 2005 Omni William Penn Hotel, 9:30 Every decision tree has an influential variable Pittsburgh, PA Ryan O’Donnell, Michael Saks, Oded Schramm and Rocco Servedio Sponsored by the IEEE Computer Society Technical Committee on Mathematical Foundations of Computing 9:50 Lower Bounds for the Noisy Broadcast Problem In cooperation with ACM SIGACT Navin Goyal, Guy Kindler and Michael Saks Break 10:10am – 10:30am FOCS ’05 gratefully acknowledges financial support from Microsoft Research, Yahoo! Research, and the CMU Aladdin center Session 2: 10:30am – 12:10pm Chair: Satish Rao SATURDAY October 22, 2005 10:30 The Unique Games Conjecture, Integrality Gap for Cut Problems and Embeddability of Negative Type Metrics Tutorials held at CMU University Center into `1 [Best paper award] Reception at Omni William Penn Hotel, Monongahela Room, Subhash Khot and Nisheeth Vishnoi 17th floor 10:50 The Closest Substring problem with small distances Tutorial 1: 1:30pm – 3:30pm Daniel Marx (McConomy Auditorium) Chair: Irit Dinur 11:10 Fitting tree metrics: Hierarchical clustering and Phy- logeny Subhash Khot Nir Ailon and Moses Charikar On the Unique Games Conjecture 11:30 Metric Embeddings with Relaxed Guarantees Break 3:30pm – 4:00pm Ittai Abraham, Yair Bartal, T-H. -
Hierarchical Clustering with Global Objectives: Approximation Algorithms and Hardness Results
HIERARCHICAL CLUSTERING WITH GLOBAL OBJECTIVES: APPROXIMATION ALGORITHMS AND HARDNESS RESULTS ADISSERTATION SUBMITTED TO THE DEPARTMENT OF COMPUTER SCIENCE AND THE COMMITTEE ON GRADUATE STUDIES OF STANFORD UNIVERSITY IN PARTIAL FULFILLMENT OF THE REQUIREMENTS FOR THE DEGREE OF DOCTOR OF PHILOSOPHY Evangelos Chatziafratis June 2020 © 2020 by Evangelos Chatziafratis. All Rights Reserved. Re-distributed by Stanford University under license with the author. This work is licensed under a Creative Commons Attribution- Noncommercial 3.0 United States License. http://creativecommons.org/licenses/by-nc/3.0/us/ This dissertation is online at: http://purl.stanford.edu/bb164pj1759 ii I certify that I have read this dissertation and that, in my opinion, it is fully adequate in scope and quality as a dissertation for the degree of Doctor of Philosophy. Tim Roughgarden, Primary Adviser I certify that I have read this dissertation and that, in my opinion, it is fully adequate in scope and quality as a dissertation for the degree of Doctor of Philosophy. Moses Charikar, Co-Adviser I certify that I have read this dissertation and that, in my opinion, it is fully adequate in scope and quality as a dissertation for the degree of Doctor of Philosophy. Li-Yang Tan I certify that I have read this dissertation and that, in my opinion, it is fully adequate in scope and quality as a dissertation for the degree of Doctor of Philosophy. Gregory Valiant Approved for the Stanford University Committee on Graduate Studies. Stacey F. Bent, Vice Provost for Graduate Education This signature page was generated electronically upon submission of this dissertation in electronic format. -
Constraint Clustering and Parity Games
Constrained Clustering Problems and Parity Games Clemens Rösner geboren in Ulm Dissertation zur Erlangung des Doktorgrades (Dr. rer. nat.) der Mathematisch-Naturwissenschaftlichen Fakultät der Rheinischen Friedrich-Wilhelms-Universität Bonn Bonn 2019 1. Gutachter: Prof. Dr. Heiko Röglin 2. Gutachterin: Prof. Dr. Anne Driemel Tag der mündlichen Prüfung: 05. September 2019 Erscheinungsjahr: 2019 Angefertigt mit Genehmigung der Mathematisch-Naturwissenschaftlichen Fakultät der Rheinischen Friedrich-Wilhelms-Universität Bonn Abstract Clustering is a fundamental tool in data mining. It partitions points into groups (clusters) and may be used to make decisions for each point based on its group. We study several clustering objectives. We begin with studying the Euclidean k-center problem. The k-center problem is a classical combinatorial optimization problem which asks to select k centers and assign each input point in a set P to one of the centers, such that the maximum distance of any input point to its assigned center is minimized. The Euclidean k-center problem assumes that the input set P is a subset of a Euclidean space and that each location in the Euclidean space can be chosen as a center. We focus on the special case with k = 1, the smallest enclosing ball problem: given a set of points in m-dimensional Euclidean space, find the smallest sphere enclosing all the points. We combine known results about convex optimization with structural properties of the smallest enclosing ball to create a new algorithm. We show that on instances with rational coefficients our new algorithm computes the exact center of the optimal solutions and has a worst-case run time that is polynomial in the size of the input. -
Implementation of Locality Sensitive Hashing Techniques
Implementation of Locality Sensitive Hashing Techniques Project Report submitted in partial fulfillment of the requirement for the degree of Bachelor of Technology. in Computer Science & Engineering under the Supervision of Dr. Nitin Chanderwal By Srishti Tomar(111210) to Jaypee University of Information and TechnologyWaknaghat, Solan – 173234, Himachal Pradesh i Certificate This is to certify that project report entitled “Implementaion of Locality Sensitive Hashing Techniques”, submitted by Srishti Tomar in partial fulfillment for the award of degree of Bachelor of Technology in Computer Science & Engineering to Jaypee University of Information Technology, Waknaghat, Solan has been carried out under my supervision. This work has not been submitted partially or fully to any other University or Institute for the award of this or any other degree or diploma. Date: Supervisor’s Name: Dr. Nitin Chanderwal Designation : Associate Professor ii Acknowledgement I am highly indebted to Jaypee University of Information Technology for their guidance and constant supervision as well as for providing necessary information regarding the project & also for their support in completing the project. I would like to express my gratitude towards my parents & Project Guide for their kind co-operation and encouragement which help me in completion of this project. I would like to express my special gratitude and thanks to industry persons for giving me such attention and time. My thanks and appreciations also go to my colleague in developing the project and people who have willingly helped me out with their abilities Date: Name of the student: Srishti Tomar iii Table of Content S. No. Topic Page No. 1. Abstract 1 2. -
Model Checking Large Design Spaces: Theory, Tools, and Experiments
Iowa State University Capstones, Theses and Graduate Theses and Dissertations Dissertations 2020 Model checking large design spaces: Theory, tools, and experiments Rohit Dureja Iowa State University Follow this and additional works at: https://lib.dr.iastate.edu/etd Recommended Citation Dureja, Rohit, "Model checking large design spaces: Theory, tools, and experiments" (2020). Graduate Theses and Dissertations. 18304. https://lib.dr.iastate.edu/etd/18304 This Thesis is brought to you for free and open access by the Iowa State University Capstones, Theses and Dissertations at Iowa State University Digital Repository. It has been accepted for inclusion in Graduate Theses and Dissertations by an authorized administrator of Iowa State University Digital Repository. For more information, please contact [email protected]. Model checking large design spaces: Theory, tools, and experiments by Rohit Dureja A dissertation submitted to the graduate faculty in partial fulfillment of the requirements for the degree of DOCTOR OF PHILOSOPHY Major: Computer Science Program of Study Committee: Kristin Y. Rozier, Co-major Professor Gianfranco Ciardo, Co-major Professor Samik Basu Robyn Lutz Hridesh Rajan The student author, whose presentation of the scholarship herein was approved by the program of study committee, is solely responsible for the content of this dissertation. The Graduate College will ensure this dissertation is globally accessible and will not permit alterations after a degree is conferred. Iowa State University Ames, Iowa 2020 Copyright © Rohit Dureja, 2020. All rights reserved. ii DEDICATION To my family. iii TABLE OF CONTENTS LIST OF FIGURES . vi LIST OF TABLES . .x ABSTRACT . xi CHAPTER 1. INTRODUCTION . .1 1.1 Motivation . -
Detecting Near-Duplicates for Web Crawling
WWW 2007 / Track: Data Mining Session: Similarity Search Detecting Near-Duplicates for Web Crawling ∗ Gurmeet Singh Manku Arvind Jain Anish Das Sarma Google Inc. Google Inc. Stanford University [email protected] [email protected] [email protected] ABSTRACT Documents that are exact duplicates of each other (due to Near-duplicate web documents are abundant. Two such mirroring and plagiarism) are easy to identify by standard documents differ from each other in a very small portion checksumming techniques. A more difficult problem is the that displays advertisements, for example. Such differences identification of near-duplicate documents. Two such docu- are irrelevant for web search. So the quality of a web crawler ments are identical in terms of content but differ in a small increases if it can assess whether a newly crawled web page portion of the document such as advertisements, counters is a near-duplicate of a previously crawled web page or not. and timestamps. These differences are irrelevant for web In the course of developing a near-duplicate detection sys- search. So if a newly-crawled page Pduplicate is deemed a tem for a multi-billion page repository, we make two research near-duplicate of an already-crawled page P , the crawl en- contributions. First, we demonstrate that Charikar's finger- gine should ignore Pduplicate and all its out-going links (in- printing technique is appropriate for this goal. Second, we tuition suggests that these are probably near-duplicates of P 1 present an algorithmic technique for identifying existing f- pages reachable from ). Elimination of near-duplicates bit fingerprints that differ from a given fingerprint in at most saves network bandwidth, reduces storage costs and im- k bit-positions, for small k. -
Time-Release Cryptography from Minimal Circuit Assumptions
Time-release Cryptography from Minimal Circuit Assumptions Samuel Jaques∗ Hart Montgomeryy Arnab Royz Abstract Time-release cryptography requires problems that take a long time to solve and take just as long even with significant computational resources. While time-release cryptography originated with the seminal paper of Rivest, Shamir and Wagner (’96), it has gained special visibility recently due to new time-release primitives, like verifiable delay functions (VDFs) and sequential proofs of work, and their novel blockchain applications. In spite of this recent progress, security definitions remain inconsistent and fragile, and foundational treatment of these primitives is scarce. Relationships between the various time-release primitives are elusive, with few connections to standard cryptographic assumptions. We systematically address these drawbacks. We define formal notions of sequential functions, the building blocks of time-release cryptography. The new definitions are robust against change of machine models, making them more amenable to complexity theoretic treatment. We demonstrate the equivalence of various types of sequential functions under standard cryptographic assumptions. The time-release primitives in the literature (such as those defined by Bitansky et al. (ITCS ’16)) imply that these primitives exist, as well as the converse. However, showing that a given construction is a sequential function is a hard circuit lower bound problem. To our knowledge, no results show that standard cryptographic assumptions imply any sequen- tiality. For example, repeated squaring over RSA groups is assumed to be sequential, but nothing connects this conjecture to standard hardness assumptions. To circumvent this, we construct a function that we prove is sequential if there exists any sequential function, without needing any specific knowledge of this hypothetical function. -
Moses Charikar Stanford University
Moses Charikar Stanford University Friday, March 22, 2019 3:00 pm Luddy Hall, Rm. 1106 Importance Sampling in High Dimensions via Hashing Abstract: Locality sensitive hashing (LSH) is a popular technique for nearest neighbor search in high dimensional data sets. Recently, a new view at LSH as a biased sampling technique has been fruitful for density estimation problems in high dimensions. Given a set of points and a query point, the goal (roughly) is to estimate the density of the data set around the query. One way to formalize this is by kernel density estimation: Given a function that decays with distance and represents the "influence" of a data point at the query, sum up this influence function over the data set. Yet another way to formalize this problem is by counting the number of data points within a certain radius of the query. While these problems can easily be solved by making a linear pass over the data, this can be prohibitive for large data sets and multiple queries. Can we preprocess the data so as to answer queries efficiently? This talk will survey several recent papers that use locality sensitive hashing to design unbiased estimators for such density estimation problems and their extensions. This talk will survey joint works with Arturs Backurs, Piotr Indyk, Vishnu Natchu, Paris Syminelakis and Xian (Carrie) Wu. Biography: Moses Charikar is the Donald E. Knuth professor of Computer Science at Stanford University. He obtained his PhD from Stanford in 2000, spent a year in the research group at Google, and was on the faculty at Princeton from 2001-2015. -
Approximation Algorithms for Grammar-Based
Approximation Algorithms for Grammar-Based Data Compression by Eric Lehman Submitted to the Department of Electrical Engineering and Computer Science in partial fulfillment of the requirements for the degree of Doctor of Philosophy at the MASSACHUSETTS INSTITUTE OF TECHNOLOGY February 2002 © Eric Lehman, MMII. All rights reserved. The author hereby grants to MIT permission to reproduce and E'AKER distribute publicly paper and electronic copies of this thesis dm STSITTUCE in whole or in part. ArPR 1 6 2002 LIBRARIES Author ............... Department of Electrical Engineering and Computer Science February 1, 2002 Certified by ....................... Madhu Sudan Associate Professor of Electrical Enginjering and Computer Science Thesjs Supevisor Accepted by ............. ...... Arthur C. Smith Chairman, Department Committee on Graduate Students 2 Approximation Algorithms for Grammar-Based Data Compression by Eric Lehman Submitted to the Department of Electrical Engineering and Computer Science on February 1, 2002, in partial fulfillment of the requirements for the degree of Doctor of Philosophy Abstract This thesis considers the smallest grammar problem: find the smallest context-free grammar that generates exactly one given string. We show that this problem is intractable, and so our objective is to find approximation algorithms. This simple question is connected to many areas of research. Most importantly, there is a link to data compression; instead of storing a long string, one can store a small grammar that generates it. A small grammar for a string also naturally brings out under- lying patterns, a fact that is useful, for example, in DNA analysis. Moreover, the size of the smallest context-free grammar generating a string can be regarded as a computable relaxation of Kolmogorov complexity. -
Rajeev Motwani
THEORY OF COMPUTING, Volume 8 (2012), pp. 55–68 www.theoryofcomputing.org SPECIAL ISSUE IN HONOR OF RAJEEV MOTWANI Rajeev Motwani (1962-2009) Prabhakar Raghavan∗ Received: September 13, 2010; published: March 31, 2012. Abstract: Rajeev Motwani was a pre-eminent theoretical computer scientist of his genera- tion, a technology thought leader, an insightful venture capitalist, and a mentor to some of the most influential entrepreneurs in Silicon Valley in the first decade of the 21st century. This article presents an overview of Rajeev’s research, and provides a window to his early life and the various influences that shaped his research and professional career—it is a small celebration of his wonderful life and many achievements. ACM Classification: K.2/People, F.2.2 AMS Classification: 01A70, 68-00 Key words and phrases: biography 1 Early Life and Education Rajeev Motwani was born on March 24, 1962 in the Indian city of Jammu to Lieutenant Colonel Hotchand Motwani, an officer in the Indian Army, and Namita Motwani (nee´ Sushila). His family included brothers Sanjeev and Suneev. Given his father’s army career, Rajeev’s family moved often and lived in various parts of India before settling down in New Delhi. Those who knew Rajeev as a young boy remember him as an avid reader. When Rajeev was seven, his father was stationed in the scenic town of Devlali near Mumbai, India. His family would walk a kilometer to the local library to get books, and the seven-year-old Rajeev would be seen reading the books ∗Based on contributions by Sanjeev Arora, Gautam Bhargava, Piotr Indyk, Asha Jadeja, Sanjeev Motwani, Prabhakar Raghavan, and G. -
Practical Hash Functions for Similarity Estimation and Dimensionality Reduction
Practical Hash Functions for Similarity Estimation and Dimensionality Reduction Søren Dahlgaard Mathias Bæk Tejs Knudsen University of Copenhagen / SupWiz University of Copenhagen / SupWiz [email protected] [email protected] Mikkel Thorup University of Copenhagen [email protected] Abstract Hashing is a basic tool for dimensionality reduction employed in several aspects of machine learning. However, the perfomance analysis is often carried out under the abstract assumption that a truly random unit cost hash function is used, without concern for which concrete hash function is employed. The concrete hash function may work fine on sufficiently random input. The question is if they can be trusted in the real world where they may be faced with more structured input. In this paper we focus on two prominent applications of hashing, namely similarity estimation with the one permutation hashing (OPH) scheme of Li et al. [NIPS’12] and feature hashing (FH) of Weinberger et al. [ICML’09], both of which have found numerous applications, i.e. in approximate near-neighbour search with LSH and large-scale classification with SVM. We consider the recent mixed tabulation hash function of Dahlgaard et al. [FOCS’15] which was proved theoretically to perform like a truly random hash function in many applications, including the above OPH. Here we first show im- proved concentration bounds for FH with truly random hashing and then argue that mixed tabulation performs similar when the input vectors are not too dense. Our main contribution, however, is an experimental comparison of different hashing schemes when used inside FH, OPH, and LSH. -
STOC ’98 to Qualify for the Early Registration Fee, Your Registra- Tion Application Must Be Postmarked by Thursday, April 30, 1998
Registration for STOC '98 To qualify for the early registration fee, your registra- tion application must be postmarked by Thursday, April 30, 1998. The non-student registration fee in- cludes the Saturday night reception, the Sunday night STOC '98 business meeting, the Monday night banquet, coffee breaks, lunches, and a copy of the proceedings. The student fee includes all of the above except the banquet. Mail the form below, along with credit card informa- tion or a check or money order in U.S. currency drawn through a U.S. bank, made payable to ACM/STOC'98. STOC '98 Computer Science Program University of Texas at Dallas P.O. Box 830688, EC 31 Richardson, TX 75083{0688 Name Address Email Phone FAX Special Needs (Please specify) Affiliation (as you wish it to appear on your name tag): The 30th Annual Please circle one amount below and fill in your member- ACM Symposium on ship number if appropriate: # . Theory of Computing Registrants in the \Other" category will automatically become SIGACT members for a period of one year. Fee (US$) after April 30 ACM or SIGACT Member 315 390 Student 125 190 Dallas, Texas Other 390 465 May 24 { May 26, 1998 Check your dietary preference: Kosher 2 Vegetarian 2 No Restriction 2 Number of additional proceedings $54 each: Number of additional Banquet tickets $55 each: Total included: $ Sponsored by ACM SIGACT Credit Card Information (if applicable): VISA / MasterCard / American Express (circle) with support from Microsoft one Card # Exp. Date: Signature Hotel Reservations Deadline: April 30, 1998 The conference will be held at the Fairmont Hotel, Dal- las, Texas.