Application of Genomic Big Data in Plant Breeding: Past, Present, and Future
Total Page:16
File Type:pdf, Size:1020Kb
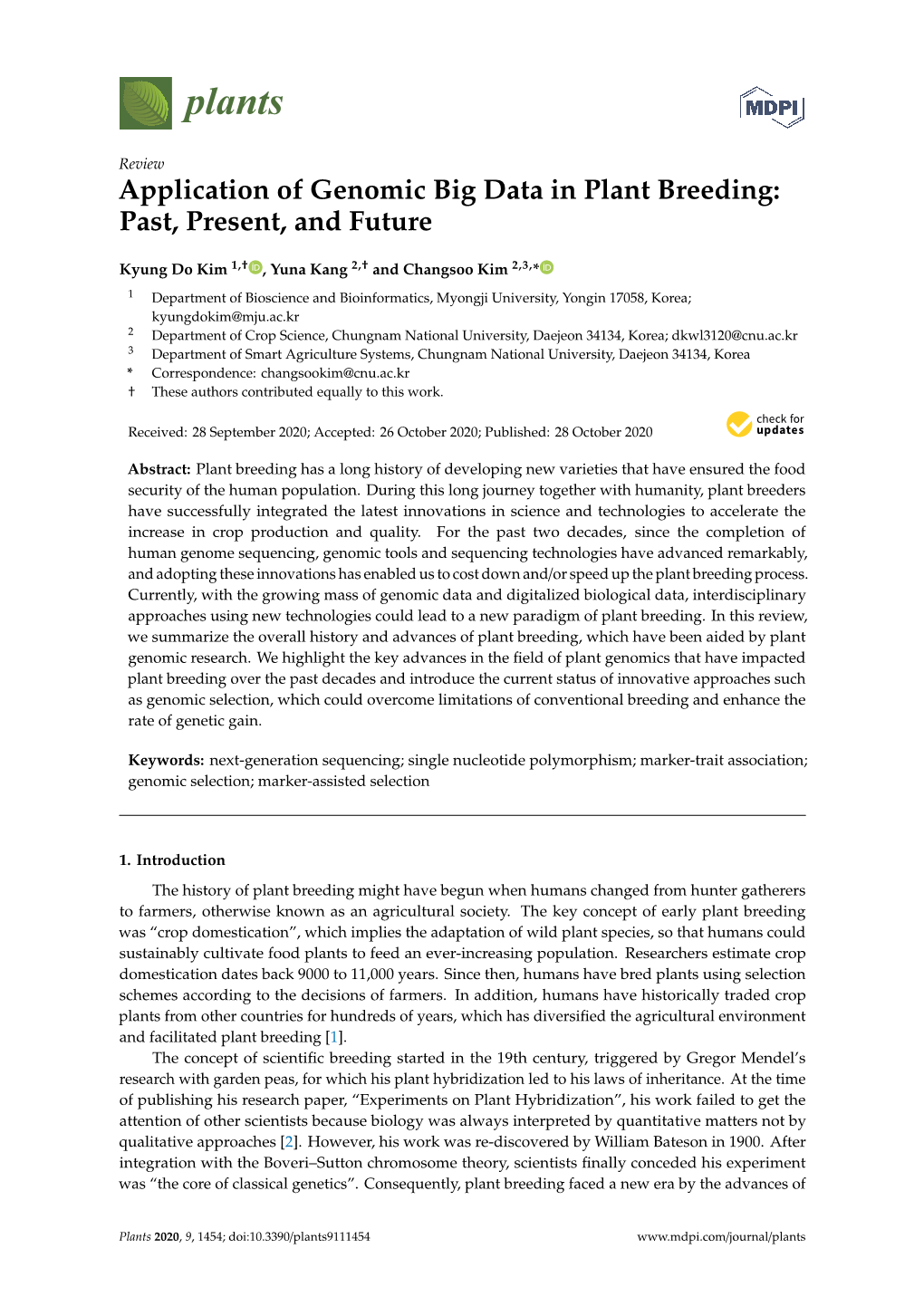
Load more
Recommended publications
-
Reference Genome Sequence of the Model Plant Setaria
UC Davis UC Davis Previously Published Works Title Reference genome sequence of the model plant Setaria Permalink https://escholarship.org/uc/item/2rv1r405 Journal Nature Biotechnology, 30(6) ISSN 1087-0156 1546-1696 Authors Bennetzen, Jeffrey L Schmutz, Jeremy Wang, Hao et al. Publication Date 2012-05-13 DOI 10.1038/nbt.2196 Peer reviewed eScholarship.org Powered by the California Digital Library University of California ARTICLES Reference genome sequence of the model plant Setaria Jeffrey L Bennetzen1,13, Jeremy Schmutz2,3,13, Hao Wang1, Ryan Percifield1,12, Jennifer Hawkins1,12, Ana C Pontaroli1,12, Matt Estep1,4, Liang Feng1, Justin N Vaughn1, Jane Grimwood2,3, Jerry Jenkins2,3, Kerrie Barry3, Erika Lindquist3, Uffe Hellsten3, Shweta Deshpande3, Xuewen Wang5, Xiaomei Wu5,12, Therese Mitros6, Jimmy Triplett4,12, Xiaohan Yang7, Chu-Yu Ye7, Margarita Mauro-Herrera8, Lin Wang9, Pinghua Li9, Manoj Sharma10, Rita Sharma10, Pamela C Ronald10, Olivier Panaud11, Elizabeth A Kellogg4, Thomas P Brutnell9,12, Andrew N Doust8, Gerald A Tuskan7, Daniel Rokhsar3 & Katrien M Devos5 We generated a high-quality reference genome sequence for foxtail millet (Setaria italica). The ~400-Mb assembly covers ~80% of the genome and >95% of the gene space. The assembly was anchored to a 992-locus genetic map and was annotated by comparison with >1.3 million expressed sequence tag reads. We produced more than 580 million RNA-Seq reads to facilitate expression analyses. We also sequenced Setaria viridis, the ancestral wild relative of S. italica, and identified regions of differential single-nucleotide polymorphism density, distribution of transposable elements, small RNA content, chromosomal rearrangement and segregation distortion. -
Y Chromosome Dynamics in Drosophila
Y chromosome dynamics in Drosophila Amanda Larracuente Department of Biology Sex chromosomes X X X Y J. Graves Sex chromosome evolution Proto-sex Autosomes chromosomes Sex Suppressed determining recombination Differentiation X Y Reviewed in Rice 1996, Charlesworth 1996 Y chromosomes • Male-restricted • Non-recombining • Degenerate • Heterochromatic Image from Willard 2003 Drosophila Y chromosome D. melanogaster Cen Hoskins et al. 2015 ~40 Mb • ~20 genes • Acquired from autosomes • Heterochromatic: Ø 80% is simple satellite DNA Photo: A. Karwath Lohe et al. 1993 Satellite DNA • Tandem repeats • Heterochromatin • Centromeres, telomeres, Y chromosomes Yunis and Yasmineh 1970 http://www.chrombios.com Y chromosome assembly challenges • Repeats are difficult to sequence • Underrepresented • Difficult to assemble Genome Sequence read: Short read lengths cannot span repeats Single molecule real-time sequencing • Pacific Biosciences • Average read length ~15 kb • Long reads span repeats • Better genome assemblies Zero mode waveguide Eid et al. 2009 Comparative Y chromosome evolution in Drosophila I. Y chromosome assemblies II. Evolution of Y-linked genes Drosophila genomes 2 Mya 0.24 Mya Photo: A. Karwath P6C4 ~115X ~120X ~85X ~95X De novo genome assembly • Assemble genome Iterative assembly: Canu, Hybrid, Quickmerge • Polish reference Quiver x 2; Pilon 2L 2R 3L 3R 4 X Y Assembled genome Mahul Chakraborty, Ching-Ho Chang 2L 2R 3L 3R 4 X Y Y X/A heterochromatin De novo genome assembly species Total bp # contigs NG50 D. simulans 154,317,203 161 21,495729 -
The Bacteria Genome Pipeline (BAGEP): an Automated, Scalable Workflow for Bacteria Genomes with Snakemake
The Bacteria Genome Pipeline (BAGEP): an automated, scalable workflow for bacteria genomes with Snakemake Idowu B. Olawoye1,2, Simon D.W. Frost3,4 and Christian T. Happi1,2 1 Department of Biological Sciences, Faculty of Natural Sciences, Redeemer's University, Ede, Osun State, Nigeria 2 African Centre of Excellence for Genomics of Infectious Diseases (ACEGID), Redeemer's University, Ede, Osun State, Nigeria 3 Microsoft Research, Redmond, WA, USA 4 London School of Hygiene & Tropical Medicine, University of London, London, United Kingdom ABSTRACT Next generation sequencing technologies are becoming more accessible and affordable over the years, with entire genome sequences of several pathogens being deciphered in few hours. However, there is the need to analyze multiple genomes within a short time, in order to provide critical information about a pathogen of interest such as drug resistance, mutations and genetic relationship of isolates in an outbreak setting. Many pipelines that currently do this are stand-alone workflows and require huge computational requirements to analyze multiple genomes. We present an automated and scalable pipeline called BAGEP for monomorphic bacteria that performs quality control on FASTQ paired end files, scan reads for contaminants using a taxonomic classifier, maps reads to a reference genome of choice for variant detection, detects antimicrobial resistant (AMR) genes, constructs a phylogenetic tree from core genome alignments and provide interactive short nucleotide polymorphism (SNP) visualization across -
Lawrence Berkeley National Laboratory Recent Work
Lawrence Berkeley National Laboratory Recent Work Title 1,003 reference genomes of bacterial and archaeal isolates expand coverage of the tree of life. Permalink https://escholarship.org/uc/item/7cx5710p Journal Nature biotechnology, 35(7) ISSN 1087-0156 Authors Mukherjee, Supratim Seshadri, Rekha Varghese, Neha J et al. Publication Date 2017-07-01 DOI 10.1038/nbt.3886 Peer reviewed eScholarship.org Powered by the California Digital Library University of California RESOU r CE OPEN 1,003 reference genomes of bacterial and archaeal isolates expand coverage of the tree of life Supratim Mukherjee1,10, Rekha Seshadri1,10, Neha J Varghese1, Emiley A Eloe-Fadrosh1, Jan P Meier-Kolthoff2 , Markus Göker2 , R Cameron Coates1,9, Michalis Hadjithomas1, Georgios A Pavlopoulos1 , David Paez-Espino1 , Yasuo Yoshikuni1, Axel Visel1 , William B Whitman3, George M Garrity4,5, Jonathan A Eisen6, Philip Hugenholtz7 , Amrita Pati1,9, Natalia N Ivanova1, Tanja Woyke1, Hans-Peter Klenk8 & Nikos C Kyrpides1 We present 1,003 reference genomes that were sequenced as part of the Genomic Encyclopedia of Bacteria and Archaea (GEBA) initiative, selected to maximize sequence coverage of phylogenetic space. These genomes double the number of existing type strains and expand their overall phylogenetic diversity by 25%. Comparative analyses with previously available finished and draft genomes reveal a 10.5% increase in novel protein families as a function of phylogenetic diversity. The GEBA genomes recruit 25 million previously unassigned metagenomic proteins from 4,650 samples, improving their phylogenetic and functional interpretation. We identify numerous biosynthetic clusters and experimentally validate a divergent phenazine cluster with potential new chemical structure and antimicrobial activity. -
Telomere-To-Telomere Assembly of a Complete Human X Chromosome W
https://doi.org/10.1038/s41586-020-2547-7 Accelerated Article Preview Telomere-to-telomere assembly of a W complete human X chromosome E VI Received: 30 July 2019 Karen H. Miga, Sergey Koren, Arang Rhie, Mitchell R. Vollger, Ariel Gershman, Andrey Bzikadze, Shelise Brooks, Edmund Howe, David Porubsky, GlennisE A. Logsdon, Accepted: 29 May 2020 Valerie A. Schneider, Tamara Potapova, Jonathan Wood, William Chow, Joel Armstrong, Accelerated Article Preview Published Jeanne Fredrickson, Evgenia Pak, Kristof Tigyi, Milinn Kremitzki,R Christopher Markovic, online 14 July 2020 Valerie Maduro, Amalia Dutra, Gerard G. Bouffard, Alexander M. Chang, Nancy F. Hansen, Amy B. Wilfert, Françoise Thibaud-Nissen, Anthony D. Schmitt,P Jon-Matthew Belton, Cite this article as: Miga, K. H. et al. Siddarth Selvaraj, Megan Y. Dennis, Daniela C. Soto, Ruta Sahasrabudhe, Gulhan Kaya, Telomere-to-telomere assembly of a com- Josh Quick, Nicholas J. Loman, Nadine Holmes, Matthew Loose, Urvashi Surti, plete human X chromosome. Nature Rosa ana Risques, Tina A. Graves Lindsay, RobertE Fulton, Ira Hall, Benedict Paten, https://doi.org/10.1038/s41586-020-2547-7 Kerstin Howe, Winston Timp, Alice Young, James C. Mullikin, Pavel A. Pevzner, (2020). Jennifer L. Gerton, Beth A. Sullivan, EvanL E. Eichler & Adam M. Phillippy C This is a PDF fle of a peer-reviewedI paper that has been accepted for publication. Although unedited, the Tcontent has been subjected to preliminary formatting. Nature is providing this early version of the typeset paper as a service to our authors and readers. The text andR fgures will undergo copyediting and a proof review before the paper is published in its fnal form. -
Human Genome Reference Program (HGRP)
Frequently Asked Questions – Human Genome Reference Program (HGRP) Funding Announcements • RFA-HG-19-002: High Quality Human Reference Genomes (HQRG) • RFA-HG-19-003: Research and Development for Genome Reference Representations (GRR) • RFA-HG-19-004: Human Genome Reference Center (HGRC) • NOT-HG-19-011: Emphasizing Opportunity for Developing Comprehensive Human Genome Sequencing Methodologies Concept Clearance Slides https://www.genome.gov/pages/about/nachgr/september2018agendadocuments/sept2018council_hg _reference_program.pdf. *Note that this presentation includes a Concept for a fifth HGRP component seeking development of informatics tools for the pan-genome. Eligibility Questions 1. Are for-profit entities eligible to apply? a. Only higher education institutes, governments, and non-profits are eligible to apply for the HGRC (RFA-HG-19-004). b. For-profit entities are eligible to apply for HQRG and GRR (RFA-HG-19-002 and 003), and for the Notice for Comprehensive Human Genome Sequencing Methodologies (NOT-HG- 19-011). The Notice also allows SBIR applications. 2. Can foreign institutions apply or receive subcontracts? a. Foreign institutions are eligible to apply to the HQRG and GRR announcements, and Developing Comprehensive Sequencing Methodologies Notice. b. Foreign institutions, including non-domestic (U.S.) components of U.S. organizations, are not eligible for HGRC. However, the FOA does allow foreign components. c. For more information, please see the NIH Grants Policy Statement. 3. Will applications with multiple sites be considered? Yes, applications with multiple sites, providing they are eligible institutions, will be considered. Application Questions 1. How much funding is available for this program? NHGRI has set aside ~$10M total costs per year for the Human Genome Reference Program. -
De Novo Genome Assembly Versus Mapping to a Reference Genome
De novo genome assembly versus mapping to a reference genome Beat Wolf PhD. Student in Computer Science University of Würzburg, Germany University of Applied Sciences Western Switzerland [email protected] 1 Outline ● Genetic variations ● De novo sequence assembly ● Reference based mapping/alignment ● Variant calling ● Comparison ● Conclusion 2 What are variants? ● Difference between a sample (patient) DNA and a reference (another sample or a population consensus) ● Sum of all variations in a patient determine his genotype and phenotype 3 Variation types ● Small variations ( < 50bp) – SNV (Single nucleotide variation) – Indel (insertion/deletion) 4 Structural variations 5 Sequencing technologies ● Sequencing produces small overlapping sequences 6 Sequencing technologies ● Difference read lengths, 36 – 10'000bp (150-500bp is typical) ● Different sequencing technologies produce different data And different kinds of errors – Substitutions (Base replaced by other) – Homopolymers (3 or more repeated bases) ● AAAAA might be read as AAAA or AAAAAA – Insertion (Non existent base has been read) – Deletion (Base has been skipped) – Duplication (cloned sequences during PCR) – Somatic cells sequenced 7 Sequencing technologies ● Standardized output format: FASTQ – Contains the read sequence and a quality for every base http://en.wikipedia.org/wiki/FASTQ_format 8 Recreating the genome ● The problem: – Recreate the original patient genome from the sequenced reads ● For which we dont know where they came from and are noisy ● Solution: – Recreate the genome -
Practical Guideline for Whole Genome Sequencing Disclosure
Practical Guideline for Whole Genome Sequencing Disclosure Kwangsik Nho Assistant Professor Center for Neuroimaging Department of Radiology and Imaging Sciences Center for Computational Biology and Bioinformatics Indiana University School of Medicine • Kwangsik Nho discloses that he has no relationships with commercial interests. What You Will Learn Today • Basic File Formats in WGS • Practical WGS Analysis Pipeline • WGS Association Analysis Methods Whole Genome Sequencing File Formats WGS Sequencer Base calling FASTQ: raw NGS reads Aligning SAM: aligned NGS reads BAM Variant Calling VCF: Genomic Variation How have BIG data problems been solved in next generation sequencing? gkno.me Whole Genome Sequencing File Formats • FASTQ: text-based format for storing both a DNA sequence and its corresponding quality scores (File sizes are huge (raw text) ~300GB per sample) @HS2000-306_201:6:1204:19922:79127/1 ACGTCTGGCCTAAAGCACTTTTTCTGAATTCCACCCCAGTCTGCCCTTCCTGAGTGCCTGGGCAGGGCCCTTGGGGAGCTGCTGGTGGGGCTCTGAATGT + BC@DFDFFHHHHHJJJIJJJJJJJJJJJJJJJJJJJJJHGGIGHJJJJJIJEGJHGIIJJIGGIIJHEHFBECEC@@D@BDDDDD<<?DB@BDDCDCDDC @HS2000-306_201:6:1204:19922:79127/2 GGGTAAAAGGTGTCCTCAGCTAATTCTCATTTCCTGGCTCTTGGCTAATTCTCATTCCCTGGGGGCTGGCAGAAGCCCCTCAAGGAAGATGGCTGGGGTC + BCCDFDFFHGHGHIJJJJJJJJJJJGJJJIIJCHIIJJIJIJJJJIJCHEHHIJJJJJJIHGBGIIHHHFFFDEEDED?B>BCCDDCBBDACDD8<?B09 @HS2000-306_201:4:1106:6297:92330/1 CACCATCCCGCGGAGCTGCCAGATTCTCGAGGTCACGGCTTACACTGCGGAGGGCCGGCAACCCCGCCTTTAATCTGCCTACCCAGGGAAGGAAAGCCTC + CCCFFFFFHGHHHJIJJJJJJJJIJJIIIGIJHIHHIJIGHHHHGEFFFDDDDDDDDDDD@ADBDDDDDDDDDDDDEDDDDDABCDDB?BDDBDCDDDDD -
Y Chromosome
G C A T T A C G G C A T genes Article An 8.22 Mb Assembly and Annotation of the Alpaca (Vicugna pacos) Y Chromosome Matthew J. Jevit 1 , Brian W. Davis 1 , Caitlin Castaneda 1 , Andrew Hillhouse 2 , Rytis Juras 1 , Vladimir A. Trifonov 3 , Ahmed Tibary 4, Jorge C. Pereira 5, Malcolm A. Ferguson-Smith 5 and Terje Raudsepp 1,* 1 Department of Veterinary Integrative Biosciences, College of Veterinary Medicine and Biomedical Sciences, Texas A&M University, College Station, TX 77843-4458, USA; [email protected] (M.J.J.); [email protected] (B.W.D.); [email protected] (C.C.); [email protected] (R.J.) 2 Molecular Genomics Workplace, Institute for Genome Sciences and Society, Texas A&M University, College Station, TX 77843-4458, USA; [email protected] 3 Laboratory of Comparative Genomics, Institute of Molecular and Cellular Biology, 630090 Novosibirsk, Russia; [email protected] 4 Department of Veterinary Clinical Sciences, College of Veterinary Medicine, Washington State University, Pullman, WA 99164-6610, USA; [email protected] 5 Department of Veterinary Medicine, University of Cambridge, Cambridge CB3 0ES, UK; [email protected] (J.C.P.); [email protected] (M.A.F.-S.) * Correspondence: [email protected] Abstract: The unique evolutionary dynamics and complex structure make the Y chromosome the most diverse and least understood region in the mammalian genome, despite its undisputable role in sex determination, development, and male fertility. Here we present the first contig-level annotated draft assembly for the alpaca (Vicugna pacos) Y chromosome based on hybrid assembly of short- and long-read sequence data of flow-sorted Y. -
Reference Genomes and Common File Formats Overview
Reference genomes and common file formats Overview ● Reference genomes and GRC ● Fasta and FastQ (unaligned sequences) ● SAM/BAM (aligned sequences) ● Summarized genomic features ○ BED (genomic intervals) ○ GFF/GTF (gene annotation) ○ Wiggle files, BEDgraphs, BigWigs (genomic scores) Why do we need to know about reference genomes? ● Allows for genes and genomic features to be evaluated in their genomic context. ○ Gene A is close to gene B ○ Gene A and gene B are within feature C ● Can be used to align shallow targeted high-throughput sequencing to a pre-built map of an organism Genome Reference Consortium (GRC) ● Most model organism reference genomes are being regularly updated ● Reference genomes consist of a mixture of known chromosomes and unplaced contigs called as Genome Reference Assembly ● Genome Reference Consortium: ○ A collaboration of institutes which curate and maintain the reference genomes of 4 model organisms: ■ Human - GRCh38.p9 (26 Sept 2016) ■ Mouse - GRCm38.p5 (29 June 2016) ■ Zebrafish - GRCz10 (12 Sept 2014) ■ Chicken - Gallus_gallus-5.0 (16 Dec 2015) ○ Latest human assembly is GRCh38, patches add information to the assembly without disrupting the chromosome coordinates ● Other model organisms are maintained separately, like: ○ Drosophila - Berkeley Drosophila Genome Project Overview ● Reference genomes and GRC ● Fasta and FastQ (unaligned sequences) ● SAM/BAM (aligned sequences) ● Summarized genomic features ○ BED (genomic intervals) ○ GFF/GTF (gene annotation) ○ Wiggle files, BEDgraphs, BigWigs (genomic scores) The -
Informatics and Clinical Genome Sequencing: Opening the Black Box
©American College of Medical Genetics and Genomics REVIEW Informatics and clinical genome sequencing: opening the black box Sowmiya Moorthie, PhD1, Alison Hall, MA 1 and Caroline F. Wright, PhD1,2 Adoption of whole-genome sequencing as a routine biomedical tool present an overview of the data analysis and interpretation pipeline, is dependent not only on the availability of new high-throughput se- the wider informatics needs, and some of the relevant ethical and quencing technologies, but also on the concomitant development of legal issues. methods and tools for data collection, analysis, and interpretation. Genet Med 2013:15(3):165–171 It would also be enormously facilitated by the development of deci- sion support systems for clinicians and consideration of how such Key Words: bioinformatics; data analysis; massively parallel; information can best be incorporated into care pathways. Here we next-generation sequencing INTRODUCTION Each of these steps requires purpose-built databases, algo- Technological advances have resulted in a dramatic fall in the rithms, software, and expertise to perform. By and large, issues cost of human genome sequencing. However, the sequencing related to primary analysis have been solved and are becom- assay is only the beginning of the process of converting a sample ing increasingly automated, and are therefore not discussed of DNA into meaningful genetic information. The next step of further here. Secondary analysis is also becoming increasingly data collection and analysis involves extensive use of various automated for human genome resequencing, and methods of computational methods for converting raw data into sequence mapping reads to the most recent human genome reference information, and the application of bioinformatics techniques sequence (GRCh37), and calling variants from it, are becom- for the interpretation of that sequence. -
Using DECIPHER V2.0 to Analyze Big Biological Sequence Data in R by Erik S
CONTRIBUTED RESEARCH ARTICLES 352 Using DECIPHER v2.0 to Analyze Big Biological Sequence Data in R by Erik S. Wright Abstract In recent years, the cost of DNA sequencing has decreased at a rate that has outpaced improvements in memory capacity. It is now common to collect or have access to many gigabytes of biological sequences. This has created an urgent need for approaches that analyze sequences in subsets without requiring all of the sequences to be loaded into memory at one time. It has also opened opportunities to improve the organization and accessibility of information acquired in sequencing projects. The DECIPHER package offers solutions to these problems by assisting in the curation of large sets of biological sequences stored in compressed format inside a database. This approach has many practical advantages over standard bioinformatics workflows, and enables large analyses that would otherwise be prohibitively time consuming. Introduction With the advent of next-generation sequencing technologies, the cost of sequencing DNA has plum- meted, facilitating a deluge of biological sequences (Hayden, 2014). Since the cost of computer storage space has not kept pace with biologists’ ability to generate data, multiple compression methods have been developed for compactly storing nucleotide sequences (Deorowicz and Grabowski, 2013). These methods are critical for efficiently transmitting and preserving the outputs of next-generation sequencing machines. However, far less emphasis has been placed on the organization and usability of the massive amounts of biological sequences that are now routine in bioinformatics work. It is still commonplace to decompress large files and load them entirely into memory before analyses are performed.