Ffmpeg Documentation Table of Contents
Total Page:16
File Type:pdf, Size:1020Kb
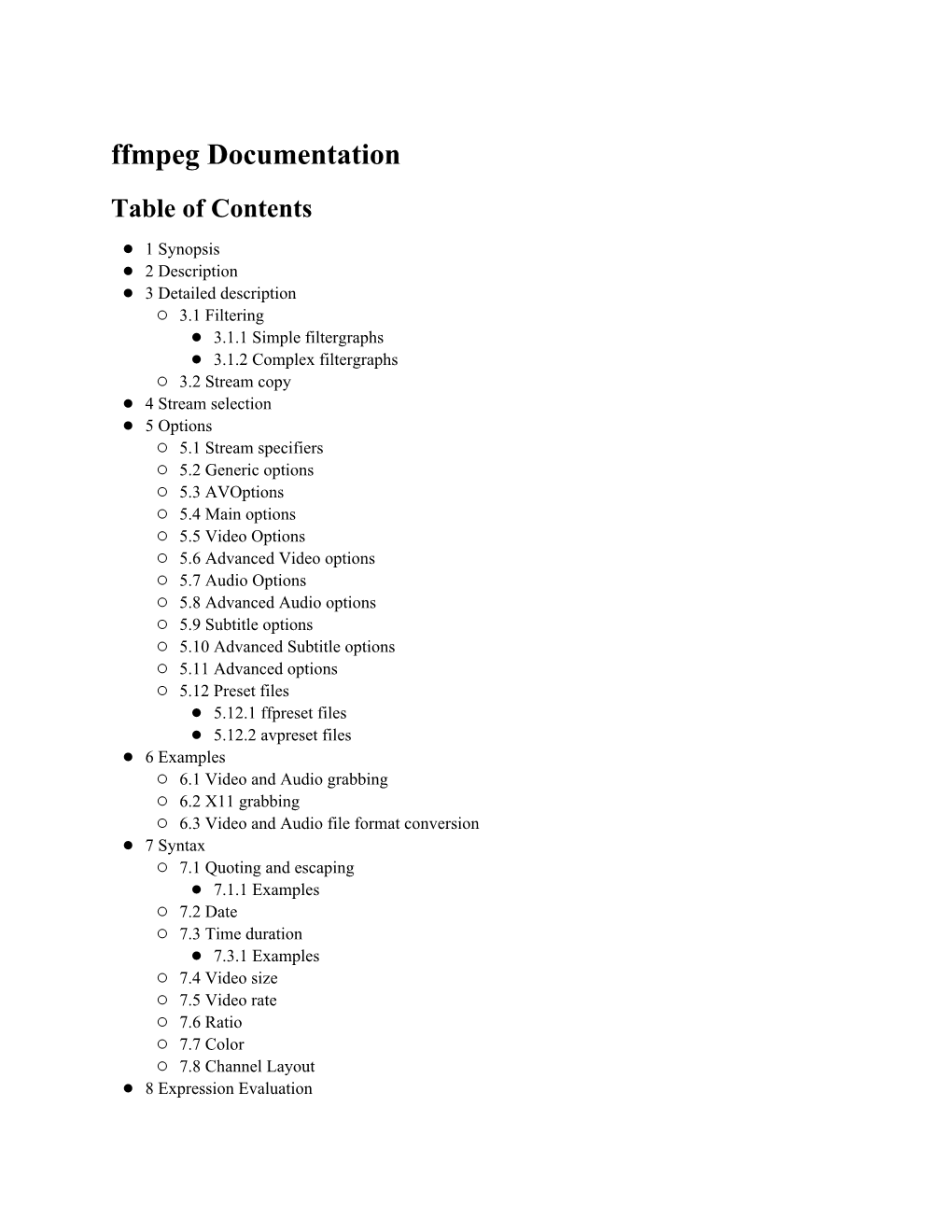
Load more
Recommended publications
-
EECS 442 Computer Vision: Homework 2
EECS 442 Computer Vision: Homework 2 Instructions • This homework is due at 11:59:59 p.m. on Friday February 26th, 2021. • The submission includes two parts: 1. To Canvas: submit a zip file of all of your code. We have indicated questions where you have to do something in code in red. Your zip file should contain a single directory which has the same name as your uniqname. If I (David, uniqname fouhey) were submitting my code, the zip file should contain a single folder fouhey/ containing all required files. What should I submit? At the end of the homework, there is a canvas submission checklist provided. We provide a script that validates the submission format here. If we don’t ask you for it, you don’t need to submit it; while you should clean up the directory, don’t panic about having an extra file or two. 2. To Gradescope: submit a pdf file as your write-up, including your answers to all the questions and key choices you made. We have indicated questions where you have to do something in the report in blue. You might like to combine several files to make a submission. Here is an example online link for combining multiple PDF files: https://combinepdf.com/. The write-up must be an electronic version. No handwriting, including plotting questions. LATEX is recommended but not mandatory. Python Environment We are using Python 3.7 for this course. You can find references for the Python standard library here: https://docs.python.org/3.7/library/index.html. -
Image Segmentation Based on Sobel Edge Detection Yuqin Yao1,A
5th International Conference on Advanced Materials and Computer Science (ICAMCS 2016) Image Segmentation Based on Sobel Edge Detection Yuqin Yao 1,a 1 Chengdu University of Information Technology, Chengdu, 610225, China a email: [email protected] Keywords: MM-sobel, edge detection, mathematical morphology, image segmentation Abstract. This paper aiming at the digital image processing, the system research to add salt and pepper noise, digital morphological preprocessing, image filtering noise reduction based on the MM-sobel edge detection and region growing for edge detection. System in this paper, the related knowledge, and application in various fields and studied and fully unifies in together, the four finished a pair of gray image edge detection is relatively complete algorithm, through the simulation experiment shows that the algorithm for edge detection effect is remarkable, in the case of almost can keep more edge details. Research overview The edge of the image is the most important visual information in an image. Image edge detection is the base of image analysis, image processing, computer vision, pattern recognition and human visual [1]. The ultimate goal is image segmentation; the largest premise is image edge detection. Image edge extraction plays an important role in image processing and machine vision. Proper image detection method is always the research hotspots in digital image processing, there are many methods to achieve edge detection, we expect to find an accurate positioning, strong anti-noise, not false, not missing detection algorithm [2]. The edge is an important feature of an image. Typically, when we take the digital image as input, the image edge is that the gray value of the image is changing radically and discontinuous, in mathematics the point is known as the break point of signal or singular point. -
Study and Comparison of Different Edge Detectors for Image
Global Journal of Computer Science and Technology Graphics & Vision Volume 12 Issue 13 Version 1.0 Year 2012 Type: Double Blind Peer Reviewed International Research Journal Publisher: Global Journals Inc. (USA) Online ISSN: 0975-4172 & Print ISSN: 0975-4350 Study and Comparison of Different Edge Detectors for Image Segmentation By Pinaki Pratim Acharjya, Ritaban Das & Dibyendu Ghoshal Bengal Institute of Technology and Management Santiniketan, West Bengal, India Abstract - Edge detection is very important terminology in image processing and for computer vision. Edge detection is in the forefront of image processing for object detection, so it is crucial to have a good understanding of edge detection operators. In the present study, comparative analyses of different edge detection operators in image processing are presented. It has been observed from the present study that the performance of canny edge detection operator is much better then Sobel, Roberts, Prewitt, Zero crossing and LoG (Laplacian of Gaussian) in respect to the image appearance and object boundary localization. The software tool that has been used is MATLAB. Keywords : Edge Detection, Digital Image Processing, Image segmentation. GJCST-F Classification : I.4.6 Study and Comparison of Different Edge Detectors for Image Segmentation Strictly as per the compliance and regulations of: © 2012. Pinaki Pratim Acharjya, Ritaban Das & Dibyendu Ghoshal. This is a research/review paper, distributed under the terms of the Creative Commons Attribution-Noncommercial 3.0 Unported License http://creativecommons.org/licenses/by-nc/3.0/), permitting all non-commercial use, distribution, and reproduction inany medium, provided the original work is properly cited. Study and Comparison of Different Edge Detectors for Image Segmentation Pinaki Pratim Acharjya α, Ritaban Das σ & Dibyendu Ghoshal ρ Abstract - Edge detection is very important terminology in noise the Canny edge detection [12-14] operator has image processing and for computer vision. -
Computer Vision: Edge Detection
Edge Detection Edge detection Convert a 2D image into a set of curves • Extracts salient features of the scene • More compact than pixels Origin of Edges surface normal discontinuity depth discontinuity surface color discontinuity illumination discontinuity Edges are caused by a variety of factors Edge detection How can you tell that a pixel is on an edge? Profiles of image intensity edges Edge detection 1. Detection of short linear edge segments (edgels) 2. Aggregation of edgels into extended edges (maybe parametric description) Edgel detection • Difference operators • Parametric-model matchers Edge is Where Change Occurs Change is measured by derivative in 1D Biggest change, derivative has maximum magnitude Or 2nd derivative is zero. Image gradient The gradient of an image: The gradient points in the direction of most rapid change in intensity The gradient direction is given by: • how does this relate to the direction of the edge? The edge strength is given by the gradient magnitude The discrete gradient How can we differentiate a digital image f[x,y]? • Option 1: reconstruct a continuous image, then take gradient • Option 2: take discrete derivative (finite difference) How would you implement this as a cross-correlation? The Sobel operator Better approximations of the derivatives exist • The Sobel operators below are very commonly used -1 0 1 1 2 1 -2 0 2 0 0 0 -1 0 1 -1 -2 -1 • The standard defn. of the Sobel operator omits the 1/8 term – doesn’t make a difference for edge detection – the 1/8 term is needed to get the right gradient -
Vision Review: Image Processing
Vision Review: Image Processing Course web page: www.cis.udel.edu/~cer/arv September 17, 2002 Announcements • Homework and paper presentation guidelines are up on web page • Readings for next Tuesday: Chapters 6, 11.1, and 18 • For next Thursday: “Stochastic Road Shape Estimation” Computer Vision Review Outline • Image formation • Image processing • Motion & Estimation • Classification Outline •Images • Binary operators •Filtering – Smoothing – Edge, corner detection • Modeling, matching • Scale space Images • An image is a matrix of pixels Note: Matlab uses • Resolution – Digital cameras: 1600 X 1200 at a minimum – Video cameras: ~640 X 480 • Grayscale: generally 8 bits per pixel → Intensities in range [0…255] • RGB color: 3 8-bit color planes Image Conversion •RGB → Grayscale: Mean color value, or weight by perceptual importance (Matlab: rgb2gray) •Grayscale → Binary: Choose threshold based on histogram of image intensities (Matlab: imhist) Color Representation • RGB, HSV (hue, saturation, value), YUV, etc. • Luminance: Perceived intensity • Chrominance: Perceived color – HS(V), (Y)UV, etc. – Normalized RGB removes some illumination dependence: Binary Operations • Dilation, erosion (Matlab: imdilate, imerode) – Dilation: All 0’s next to a 1 → 1 (Enlarge foreground) – Erosion: All 1’s next to a 0 → 0 (Enlarge background) • Connected components – Uniquely label each n-connected region in binary image – 4- and 8-connectedness –Matlab: bwfill, bwselect • Moments: Region statistics – Zeroth-order: Size – First-order: Position (centroid) -
Computer Vision Based Human Detection Md Ashikur
Computer Vision Based Human Detection Md Ashikur. Rahman To cite this version: Md Ashikur. Rahman. Computer Vision Based Human Detection. International Journal of Engineer- ing and Information Systems (IJEAIS), 2017, 1 (5), pp.62 - 85. hal-01571292 HAL Id: hal-01571292 https://hal.archives-ouvertes.fr/hal-01571292 Submitted on 2 Aug 2017 HAL is a multi-disciplinary open access L’archive ouverte pluridisciplinaire HAL, est archive for the deposit and dissemination of sci- destinée au dépôt et à la diffusion de documents entific research documents, whether they are pub- scientifiques de niveau recherche, publiés ou non, lished or not. The documents may come from émanant des établissements d’enseignement et de teaching and research institutions in France or recherche français ou étrangers, des laboratoires abroad, or from public or private research centers. publics ou privés. International Journal of Engineering and Information Systems (IJEAIS) ISSN: 2000-000X Vol. 1 Issue 5, July– 2017, Pages: 62-85 Computer Vision Based Human Detection Md. Ashikur Rahman Dept. of Computer Science and Engineering Shaikh Burhanuddin Post Graduate College Under National University, Dhaka, Bangladesh [email protected] Abstract: From still images human detection is challenging and important task for computer vision-based researchers. By detecting Human intelligence vehicles can control itself or can inform the driver using some alarming techniques. Human detection is one of the most important parts in image processing. A computer system is trained by various images and after making comparison with the input image and the database previously stored a machine can identify the human to be tested. This paper describes an approach to detect different shape of human using image processing. -
An Analysis and Implementation of the Harris Corner Detector
Published in Image Processing On Line on 2018–10–03. Submitted on 2018–06–04, accepted on 2018–09–18. ISSN 2105–1232 c 2018 IPOL & the authors CC–BY–NC–SA This article is available online with supplementary materials, software, datasets and online demo at https://doi.org/10.5201/ipol.2018.229 2015/06/16 v0.5.1 IPOL article class An Analysis and Implementation of the Harris Corner Detector Javier S´anchez1, Nelson Monz´on2, Agust´ın Salgado1 1 CTIM, Department of Computer Science, University of Las Palmas de Gran Canaria, Spain ({jsanchez, agustin.salgado}@ulpgc.es) 2 CMLA, Ecole´ Normale Sup´erieure , Universit´eParis-Saclay, France ([email protected]) Abstract In this work, we present an implementation and thorough study of the Harris corner detector. This feature detector relies on the analysis of the eigenvalues of the autocorrelation matrix. The algorithm comprises seven steps, including several measures for the classification of corners, a generic non-maximum suppression method for selecting interest points, and the possibility to obtain the corners position with subpixel accuracy. We study each step in detail and pro- pose several alternatives for improving the precision and speed. The experiments analyze the repeatability rate of the detector using different types of transformations. Source Code The reviewed source code and documentation for this algorithm are available from the web page of this article1. Compilation and usage instruction are included in the README.txt file of the archive. Keywords: Harris corner; feature detector; interest point; autocorrelation matrix; non-maximum suppression 1 Introduction The Harris corner detector [9] is a standard technique for locating interest points on an image. -
Comparison of Canny and Sobel Edge Detection in MRI Images
Comparison of Canny and Sobel Edge Detection in MRI Images Zolqernine Othman Habibollah Haron Mohammed Rafiq Abdul Kadir Faculty of Computer Science Faculty of Computer Science Biomechanics & Tissue Engineering Group, and Information System, and Information System, Faculty of Biomedical Engineering Universiti Teknologi Malaysia, Universiti Teknologi Malaysia, and Health Science, 81310 UTM Skudai, Malaysia. 81310 UTM Skudai, Malaysia. Universiti Teknologi Malaysia, [email protected] [email protected] 81310 UTM Skudai, Malaysia. [email protected] ABSTRACT Feature extraction approach in medical magnetic resonance detection method of MRI image of knee, the most widely imaging (MRI) is very important in order to perform used edge detection algorithms [5]. The Sobel operator diagnostic image analysis [1]. Edge detection is one of the performs a 2-D spatial gradient measurement on an image way to extract more information from magnetic resonance and so emphasizes regions of high spatial frequency that images. Edge detection reduces the amount of data and correspond to edges. Typically it is used to find the filters out useless information, while protecting the approximate absolute gradient magnitude at each point in an important structural properties in an image [2]. In this paper, input grayscale image. Canny edge detector uses a filter we compare Sobel and Canny edge detection method. In based on the first derivative of a Gaussian, it is susceptible order to compare between them, one slice of MRI image to noise present on raw unprocessed image data, so to begin tested with both method. Both method of the edge detection with, the raw image is convolved with a Gaussian filter. -
DENSE GRADIENT-BASED FEATURES (DEGRAF) for COMPUTATIONALLY EFFICIENT and INVARIANT FEATURE EXTRACTION in REAL-TIME APPLICATIONS Ioannis Katramados?, Toby P
DENSE GRADIENT-BASED FEATURES (DEGRAF) FOR COMPUTATIONALLY EFFICIENT AND INVARIANT FEATURE EXTRACTION IN REAL-TIME APPLICATIONS Ioannis Katramados?, Toby P. Breckony ?fCranfield University | Cosmonio Ltd}, Bedfordshire, UK yDurham University, Durham, UK ABSTRACT We propose a computationally efficient approach for the ex- traction of dense gradient-based features based on the use of localized intensity-weighted centroids within the image. Fig. 1: Dense DeGraF-β features (right) from vehicle sensing (left) Whilst prior work concentrates on sparse feature deriva- using dense feature grids (e.g. dense SIFT, [24]) found itself tions or computationally expensive dense scene sensing, we falling foul of the recent trend in feature point optimization show that Dense Gradient-based Features (DeGraF) can be - improved feature stability at the expense of computational derived based on initial multi-scale division of Gaussian cost Vs. reduced computational cost at the expense of feature preprocessing, weighted centroid gradient calculation and stability. Such applications found themselves instead requir- either local saliency (DeGraF-α) or signal-to-noise inspired ing real-time high density features that were in themselves (DeGraF-β) final stage filtering. We present two variants stable to the narrower subset of metric conditions typical of (DeGraF-α / DeGraF-β) of which the signal-to-noise based the automotive-type application genre (e.g. lesser camera ro- approach is shown to perform admirably against the state of tation, high image noise, extreme illumination changes). the art in terms of feature density, computational efficiency Whilst contemporary work aimed to address this issue via and feature stability. Our approach is evaluated under a range dense scene mapping [23, 22] (computationally enabled by of environmental conditions typical of automotive sensing GPU) or a move to scene understanding via 3D stereo sens- applications with strong feature density requirements. -
Non-Native Contents Dectection and Localization for Online Fashion Images
https://doi.org/10.2352/ISSN.2470-1173.2019.8.IMAWM-415 © 2019, Society for Imaging Science and Technology Non-native Contents Detection and Localization for Online Fashion Images∗ Litao Hu, Karthick Shankar, Zhi Li, Zhenxun Yuan, Jan Allebach; Purdue University; West Lafayette, IN Gautam Glowala, Sathya Sundaram, Perry Lee; Poshmark Inc.; Redwood City, CA Abstract Figure 1. Non-native content detection is about detecting regions of contents in an image that do not belong to the original or natu- ral contents of the image. In the online fashion market, sellers often add non-native contents to their product images in order to emphasize the features of their products and get more views. However, from the buyer’s point of view, these excessive contents are often redundant and may interfere with the evaluation of the major contents or products in the image. In this paper, we pro- pose two methods for detecting non-native content in online fash- ion images. The first one utilizes the special properties of image mosaicing and de-mosaicing where there are local correlations Figure 1: Pipeline of the First Method between pixels of an image. The second method is based on the In the second method, an gray-scale input image be used to periodic properties of interpolations which is a common process generate multiple smaller patches, with a chosen step size. Then involved in the creation of forged images. Performance of the two a feature vector will be extracted for each patch. After that, we methods are compared by testing on a dataset consisting of real use a trained Siamese classifier to classify each pair of patches images from an online fashion marketplace. -
Edge Detection 1-D &
Edge Detection CS485/685 Computer Vision Dr. George Bebis Modified by Guido Gerig for CS/BIOEN 6640 U of Utah www.cse.unr.edu/~bebis/CS485/Lectures/ EdgeDetection.ppt Edge detection is part of segmentation image human segmentation gradient magnitude • Berkeley segmentation database: http://www.eecs.berkeley.edu/Research/Projects/CS/vision/grouping/segbench/ Goal of Edge Detection • Produce a line “drawing” of a scene from an image of that scene. Why is Edge Detection Useful? • Important features can be extracted from the edges of an image (e.g., corners, lines, curves). • These features are used by higher-level computer vision algorithms (e.g., recognition). Modeling Intensity Changes • Step edge: the image intensity abruptly changes from one value on one side of the discontinuity to a different value on the opposite side. Modeling Intensity Changes (cont’d) • Ramp edge: a step edge where the intensity change is not instantaneous but occur over a finite distance. Modeling Intensity Changes (cont’d) • Ridge edge: the image intensity abruptly changes value but then returns to the starting value within some short distance (i.e., usually generated by lines). Modeling Intensity Changes (cont’d) • Roof edge: a ridge edge where the intensity change is not instantaneous but occur over a finite distance (i.e., usually generated by the intersection of two surfaces). Edge Detection Using Derivatives • Often, points that lie on an edge are detected by: (1) Detecting the local maxima or minima of the first derivative. 1st derivative (2) Detecting the zero-crossings of the second derivative. 2nd derivative Image Derivatives • How can we differentiate a digital image? – Option 1: reconstruct a continuous image, f(x,y), then compute the derivative. -
Edge Detection (Trucco, Chapt 4 and Jain Et Al., Chapt 5)
Edge detection (Trucco, Chapt 4 AND Jain et al., Chapt 5) • Definition of edges -Edges are significant local changes of intensity in an image. -Edges typically occur on the boundary between twodifferent regions in an image. • Goal of edge detection -Produce a line drawing of a scene from an image of that scene. -Important features can be extracted from the edges of an image (e.g., corners, lines, curves). -These features are used by higher-levelcomputer vision algorithms (e.g., recogni- tion). -2- • What causes intensity changes? -Various physical events cause intensity changes. -Geometric events *object boundary (discontinuity in depth and/or surface color and texture) *surface boundary (discontinuity in surface orientation and/or surface color and texture) -Non-geometric events *specularity (direct reflection of light, such as a mirror) *shadows (from other objects or from the same object) *inter-reflections • Edge descriptors Edge normal: unit vector in the direction of maximum intensity change. Edge direction: unit vector to perpendicular to the edge normal. Edge position or center: the image position at which the edge is located. Edge strength: related to the local image contrast along the normal. -3- • Modeling intensity changes -Edges can be modeled according to their intensity profiles. Step edge: the image intensity abruptly changes from one value to one side of the discontinuity to a different value on the opposite side. Ramp edge: astep edge where the intensity change is not instantaneous but occur overafinite distance. Ridge edge: the image intensity abruptly changes value but then returns to the starting value within some short distance (generated usually by lines).