A Tutorial on Fisher Information
Total Page:16
File Type:pdf, Size:1020Kb
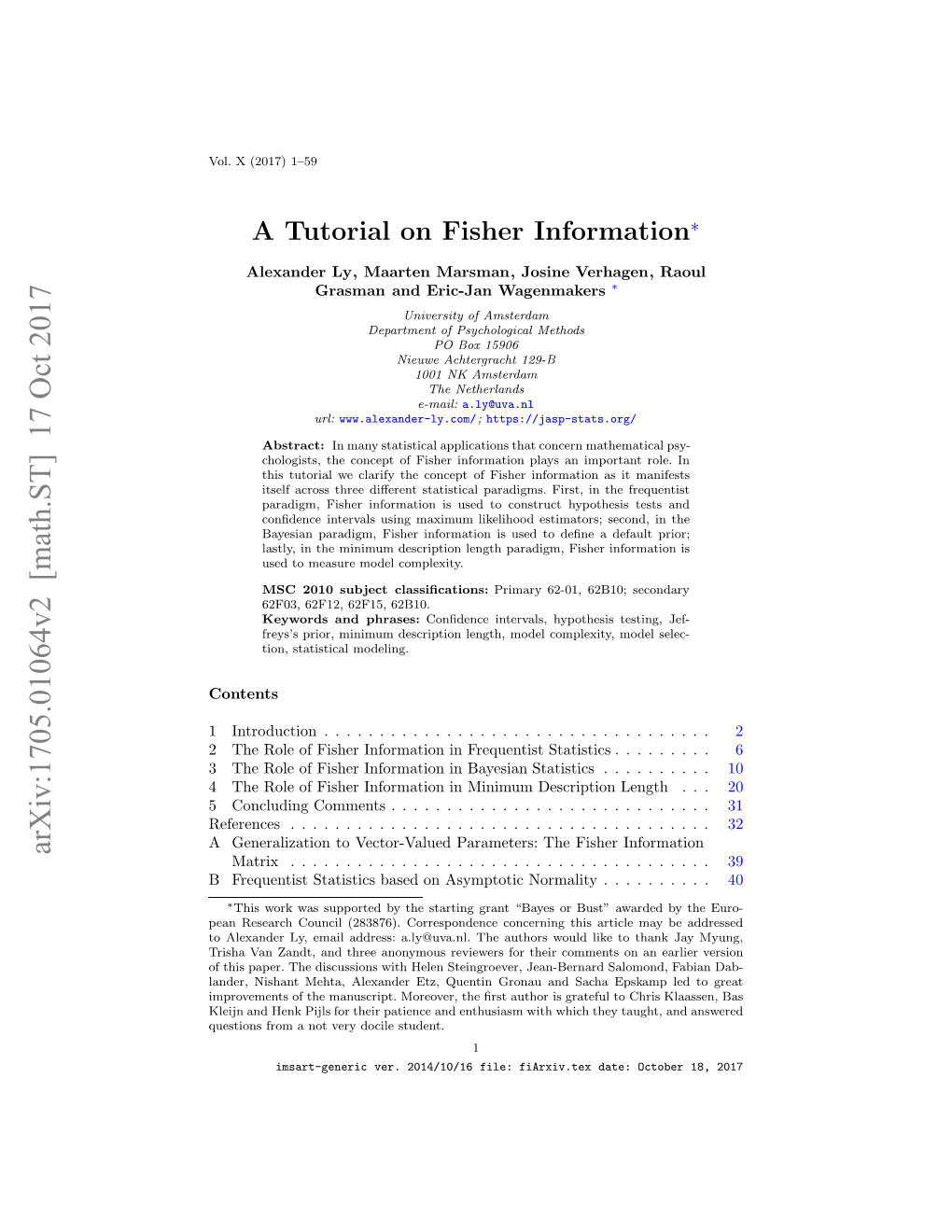
Load more
Recommended publications
-
National Academy Elects IMS Fellows Have You Voted Yet?
Volume 38 • Issue 5 IMS Bulletin June 2009 National Academy elects IMS Fellows CONTENTS The United States National Academy of Sciences has elected 72 new members and 1 National Academy elects 18 foreign associates from 15 countries in recognition of their distinguished and Raftery, Wong continuing achievements in original research. Among those elected are two IMS Adrian Raftery 2 Members’ News: Jianqing Fellows: , Blumstein-Jordan Professor of Statistics and Sociology, Center Fan; SIAM Fellows for Statistics and the Social Sciences, University of Washington, Seattle, and Wing Hung Wong, Professor 3 Laha Award recipients of Statistics and Professor of Health Research and Policy, 4 COPSS Fisher Lecturer: Department of Statistics, Stanford University, California. Noel Cressie The election was held April 28, during the business 5 Members’ Discoveries: session of the 146th annual meeting of the Academy. Nicolai Meinshausen Those elected bring the total number of active members 6 Medallion Lecture: Tony Cai to 2,150. Foreign associates are non-voting members of the Academy, with citizenship outside the United States. Meeting report: SSP Above: Adrian Raftery 7 This year’s election brings the total number of foreign 8 New IMS Fellows Below: Wing H. Wong associates to 404. The National Academy of Sciences is a private 10 Obituaries: Keith Worsley; I.J. Good organization of scientists and engineers dedicated to the furtherance of science and its use for general welfare. 12-3 JSM program highlights; It was established in 1863 by a congressional act of IMS sessions at JSM incorporation signed by Abraham Lincoln that calls on 14-5 JSM tours; More things to the Academy to act as an official adviser to the federal do in DC government, upon request, in any matter of science or 16 Accepting rejections technology. -
UC Riverside UC Riverside Previously Published Works
UC Riverside UC Riverside Previously Published Works Title Fisher information matrix: A tool for dimension reduction, projection pursuit, independent component analysis, and more Permalink https://escholarship.org/uc/item/9351z60j Authors Lindsay, Bruce G Yao, Weixin Publication Date 2012 Peer reviewed eScholarship.org Powered by the California Digital Library University of California 712 The Canadian Journal of Statistics Vol. 40, No. 4, 2012, Pages 712–730 La revue canadienne de statistique Fisher information matrix: A tool for dimension reduction, projection pursuit, independent component analysis, and more Bruce G. LINDSAY1 and Weixin YAO2* 1Department of Statistics, The Pennsylvania State University, University Park, PA 16802, USA 2Department of Statistics, Kansas State University, Manhattan, KS 66502, USA Key words and phrases: Dimension reduction; Fisher information matrix; independent component analysis; projection pursuit; white noise matrix. MSC 2010: Primary 62-07; secondary 62H99. Abstract: Hui & Lindsay (2010) proposed a new dimension reduction method for multivariate data. It was based on the so-called white noise matrices derived from the Fisher information matrix. Their theory and empirical studies demonstrated that this method can detect interesting features from high-dimensional data even with a moderate sample size. The theoretical emphasis in that paper was the detection of non-normal projections. In this paper we show how to decompose the information matrix into non-negative definite information terms in a manner akin to a matrix analysis of variance. Appropriate information matrices will be identified for diagnostics for such important modern modelling techniques as independent component models, Markov dependence models, and spherical symmetry. The Canadian Journal of Statistics 40: 712– 730; 2012 © 2012 Statistical Society of Canada Resum´ e:´ Hui et Lindsay (2010) ont propose´ une nouvelle methode´ de reduction´ de la dimension pour les donnees´ multidimensionnelles. -
Statistical Estimation in Multivariate Normal Distribution
STATISTICAL ESTIMATION IN MULTIVARIATE NORMAL DISTRIBUTION WITH A BLOCK OF MISSING OBSERVATIONS by YI LIU Presented to the Faculty of the Graduate School of The University of Texas at Arlington in Partial Fulfillment of the Requirements for the Degree of DOCTOR OF PHILOSOPHY THE UNIVERSITY OF TEXAS AT ARLINGTON DECEMBER 2017 Copyright © by YI LIU 2017 All Rights Reserved ii To Alex and My Parents iii Acknowledgements I would like to thank my supervisor, Dr. Chien-Pai Han, for his instructing, guiding and supporting me over the years. You have set an example of excellence as a researcher, mentor, instructor and role model. I would like to thank Dr. Shan Sun-Mitchell for her continuously encouraging and instructing. You are both a good teacher and helpful friend. I would like to thank my thesis committee members Dr. Suvra Pal and Dr. Jonghyun Yun for their discussion, ideas and feedback which are invaluable. I would like to thank the graduate advisor, Dr. Hristo Kojouharov, for his instructing, help and patience. I would like to thank the chairman Dr. Jianzhong Su, Dr. Minerva Cordero-Epperson, Lona Donnelly, Libby Carroll and other staffs for their help. I would like to thank my manager, Robert Schermerhorn, for his understanding, encouraging and supporting which make this happen. I would like to thank my employer Sabre and my coworkers for their support in the past two years. I would like to thank my husband Alex for his encouraging and supporting over all these years. In particularly, I would like to thank my parents -- without the inspiration, drive and support that you have given me, I might not be the person I am today. -
Memorial to Sir Harold Jeffreys 1891-1989 JOHN A
Memorial to Sir Harold Jeffreys 1891-1989 JOHN A. HUDSON and ALAN G. SMITH University of Cambridge, Cambridge, England Harold Jeffreys was one of this century’s greatest applied mathematicians, using mathematics as a means of under standing the physical world. Principally he was a geo physicist, although statisticians may feel that his greatest contribution was to the theory of probability. However, his interest in the latter subject stemmed from his realization of the need for a clear statistical method of analysis of data— at that time, travel-time readings from seismological stations across the world. He also made contributions to astronomy, fluid dynamics, meteorology, botany, psychol ogy, and photography. Perhaps one can identify Jeffreys’s principal interests from three major books that he wrote. His mathematical skills are displayed in Methods of Mathematical Physics, which he wrote with his wife Bertha Swirles Jeffreys and which was first published in 1946 and went through three editions. His Theory o f Probability, published in 1939 and also running to three editions, espoused Bayesian statistics, which were very unfashionable at the time but which have been taken up since by others and shown to be extremely powerful for the analysis of data and, in particular, image enhancement. However, the book for which he is probably best known is The Earth, Its Origin, History and Physical Consti tution, a broad-ranging account based on observations analyzed with care, using mathematics as a tool. Jeffreys’s scientific method (now known as Inverse Theory) was a logical process, clearly stated in another of his books, Scientific Inference. -
Rothamsted in the Making of Sir Ronald Fisher Scd FRS
Rothamsted in the Making of Sir Ronald Fisher ScD FRS John Aldrich University of Southampton RSS September 2019 1 Finish 1962 “the most famous statistician and mathematical biologist in the world” dies Start 1919 29 year-old Cambridge BA in maths with no prospects takes temporary job at Rothamsted Experimental Station In between 1919-33 at Rothamsted he makes a career for himself by creating a career 2 Rothamsted helped make Fisher by establishing and elevating the office of agricultural statistician—a position in which Fisher was unsurpassed by letting him do other things—including mathematical statistics, genetics and eugenics 3 Before Fisher … (9 slides) The problems were already there viz. o the relationship between harvest and weather o design of experimental trials and the analysis of their results Leading figures in agricultural science believed the problems could be treated statistically 4 Established in 1843 by Lawes and Gilbert to investigate the effective- ness of fertilisers Sir John Bennet Lawes Sir Joseph Henry Gilbert (1814-1900) land-owner, fertiliser (1817-1901) professional magnate and amateur scientist scientist (chemist) In 1902 tired Rothamsted gets make-over when Daniel Hall becomes Director— public money feeds growth 5 Crops and weather and experimental trials Crops & the weather o examined by agriculturalists—e.g. Lawes & Gilbert 1880 o subsequently treated more by meteorologists Experimental trials a hotter topic o treated in Journal of Agricultural Science o by leading figures Wood of Cambridge and Hall -
A Sufficiency Paradox: an Insufficient Statistic Preserving the Fisher
A Sufficiency Paradox: An Insufficient Statistic Preserving the Fisher Information Abram KAGAN and Lawrence A. SHEPP this it is possible to find an insufficient statistic T such that (1) holds. An example of a regular statistical experiment is constructed The following example illustrates this. Let g(x) be the density where an insufficient statistic preserves the Fisher information function of a gamma distribution Gamma(3, 1), that is, contained in the data. The data are a pair (∆,X) where ∆ is a 2 −x binary random variable and, given ∆, X has a density f(x−θ|∆) g(x)=(1/2)x e ,x≥ 0; = 0,x<0. depending on a location parameter θ. The phenomenon is based on the fact that f(x|∆) smoothly vanishes at one point; it can be Take a binary variable ∆ with eliminated by adding to the regularity of a statistical experiment P (∆=1)= w , positivity of the density function. 1 P (∆=2)= w2,w1 + w2 =1,w1 =/ w2 (2) KEY WORDS: Convexity; Regularity; Statistical experi- ment. and let Y be a continuous random variable with the conditional density f(y|∆) given by f1(y)=f(y|∆=1)=.7g(y),y≥ 0; = .3g(−y),y≤ 0, 1. INTRODUCTION (3) Let P = {p(x; θ),θ∈ Θ} be a parametric family of densities f2(y)=f(y|∆=2)=.3g(y),y≥ 0; = .7g(−y),y≤ 0. (with respect to a measure µ) of a random element X taking values in a measurable space (X , A), the parameter space Θ (4) being an interval. Following Ibragimov and Has’minskii (1981, There is nothing special in the pair (.7,.3); any pair of positive chap. -
A Parsimonious Tour of Bayesian Model Uncertainty
A Parsimonious Tour of Bayesian Model Uncertainty Pierre-Alexandre Mattei Université Côte d’Azur Inria, Maasai project-team Laboratoire J.A. Dieudonné, UMR CNRS 7351 e-mail: [email protected] Abstract: Modern statistical software and machine learning libraries are enabling semi-automated statistical inference. Within this context, it appears eas- ier and easier to try and fit many models to the data at hand, thereby reversing the Fisherian way of conducting science by collecting data after the scientific hypothesis (and hence the model) has been determined. The renewed goal of the statistician becomes to help the practitioner choose within such large and heterogeneous families of models, a task known as model selection. The Bayesian paradigm offers a systematized way of as- sessing this problem. This approach, launched by Harold Jeffreys in his 1935 book Theory of Probability, has witnessed a remarkable evolution in the last decades, that has brought about several new theoretical and methodological advances. Some of these recent developments are the focus of this survey, which tries to present a unifying perspective on work carried out by different communities. In particular, we focus on non-asymptotic out-of-sample performance of Bayesian model selection and averaging tech- niques, and draw connections with penalized maximum likelihood. We also describe recent extensions to wider classes of probabilistic frameworks in- cluding high-dimensional, unidentifiable, or likelihood-free models. Contents 1 Introduction: collecting data, fitting many models . .2 2 A brief history of Bayesian model uncertainty . .2 3 The foundations of Bayesian model uncertainty . .3 3.1 Handling model uncertainty with Bayes’s theorem . -
A Note on Inference in a Bivariate Normal Distribution Model Jaya
A Note on Inference in a Bivariate Normal Distribution Model Jaya Bishwal and Edsel A. Peña Technical Report #2009-3 December 22, 2008 This material was based upon work partially supported by the National Science Foundation under Grant DMS-0635449 to the Statistical and Applied Mathematical Sciences Institute. Any opinions, findings, and conclusions or recommendations expressed in this material are those of the author(s) and do not necessarily reflect the views of the National Science Foundation. Statistical and Applied Mathematical Sciences Institute PO Box 14006 Research Triangle Park, NC 27709-4006 www.samsi.info A Note on Inference in a Bivariate Normal Distribution Model Jaya Bishwal¤ Edsel A. Pena~ y December 22, 2008 Abstract Suppose observations are available on two variables Y and X and interest is on a parameter that is present in the marginal distribution of Y but not in the marginal distribution of X, and with X and Y dependent and possibly in the presence of other parameters which are nuisance. Could one gain more e±ciency in the point estimation (also, in hypothesis testing and interval estimation) about the parameter of interest by using the full data (both Y and X values) instead of just the Y values? Also, how should one measure the information provided by random observables or their distributions about the parameter of interest? We illustrate these issues using a simple bivariate normal distribution model. The ideas could have important implications in the context of multiple hypothesis testing or simultaneous estimation arising in the analysis of microarray data, or in the analysis of event time data especially those dealing with recurrent event data. -
Risk, Scores, Fisher Information, and Glrts (Supplementary Material for Math 494) Stanley Sawyer — Washington University Vs
Risk, Scores, Fisher Information, and GLRTs (Supplementary Material for Math 494) Stanley Sawyer | Washington University Vs. April 24, 2010 Table of Contents 1. Statistics and Esimatiors 2. Unbiased Estimators, Risk, and Relative Risk 3. Scores and Fisher Information 4. Proof of the Cram¶erRao Inequality 5. Maximum Likelihood Estimators are Asymptotically E±cient 6. The Most Powerful Hypothesis Tests are Likelihood Ratio Tests 7. Generalized Likelihood Ratio Tests 8. Fisher's Meta-Analysis Theorem 9. A Tale of Two Contingency-Table Tests 1. Statistics and Estimators. Let X1;X2;:::;Xn be an independent sample of observations from a probability density f(x; θ). Here f(x; θ) can be either discrete (like the Poisson or Bernoulli distributions) or continuous (like normal and exponential distributions). In general, a statistic is an arbitrary function T (X1;:::;Xn) of the data values X1;:::;Xn. Thus T (X) for X = (X1;X2;:::;Xn) can depend on X1;:::;Xn, but cannot depend on θ. Some typical examples of statistics are X + X + ::: + X T (X ;:::;X ) = X = 1 2 n (1:1) 1 n n = Xmax = maxf Xk : 1 · k · n g = Xmed = medianf Xk : 1 · k · n g These examples have the property that the statistic T (X) is a symmetric function of X = (X1;:::;Xn). That is, any permutation of the sample X1;:::;Xn preserves the value of the statistic. This is not true in general: For example, for n = 4 and X4 > 0, T (X1;X2;X3;X4) = X1X2 + (1=2)X3=X4 is also a statistic. A statistic T (X) is called an estimator of a parameter θ if it is a statistic that we think might give a reasonable guess for the true value of θ. -
Evaluating Fisher Information in Order Statistics Mary F
Southern Illinois University Carbondale OpenSIUC Research Papers Graduate School 2011 Evaluating Fisher Information in Order Statistics Mary F. Dorn [email protected] Follow this and additional works at: http://opensiuc.lib.siu.edu/gs_rp Recommended Citation Dorn, Mary F., "Evaluating Fisher Information in Order Statistics" (2011). Research Papers. Paper 166. http://opensiuc.lib.siu.edu/gs_rp/166 This Article is brought to you for free and open access by the Graduate School at OpenSIUC. It has been accepted for inclusion in Research Papers by an authorized administrator of OpenSIUC. For more information, please contact [email protected]. EVALUATING FISHER INFORMATION IN ORDER STATISTICS by Mary Frances Dorn B.A., University of Chicago, 2009 A Research Paper Submitted in Partial Fulfillment of the Requirements for the Master of Science Degree Department of Mathematics in the Graduate School Southern Illinois University Carbondale July 2011 RESEARCH PAPER APPROVAL EVALUATING FISHER INFORMATION IN ORDER STATISTICS By Mary Frances Dorn A Research Paper Submitted in Partial Fulfillment of the Requirements for the Degree of Master of Science in the field of Mathematics Approved by: Dr. Sakthivel Jeyaratnam, Chair Dr. David Olive Dr. Kathleen Pericak-Spector Graduate School Southern Illinois University Carbondale July 14, 2011 ACKNOWLEDGMENTS I would like to express my deepest appreciation to my advisor, Dr. Sakthivel Jeyaratnam, for his help and guidance throughout the development of this paper. Special thanks go to Dr. Olive and Dr. Pericak-Spector for serving on my committee. I would also like to thank the Department of Mathematics at SIUC for providing many wonderful learning experiences. Finally, none of this would have been possible without the continuous support and encouragement from my parents. -
Basic Statistics
Statistics Kristof Reid Assistant Professor Medical University of South Carolina Core Curriculum V5 Financial Disclosures • None Core Curriculum V5 Further Disclosures • I am not a statistician • I do like making clinical decisions based on appropriately interpreted data Core Curriculum V5 Learning Objectives • Understand why knowing statistics is important • Understand the basic principles and statistical test • Understand common statistical errors in the medical literature Core Curriculum V5 Why should I care? Indications for statistics Core Curriculum V5 47% unable to determine study design 31% did not understand p values 38% did not understand sensitivity and specificity 83% could not use odds ratios Araoye et al, JBJS 2020;102(5):e19 Core Curriculum V5 50% (or more!) of clinical research publications have at least one statistical error Thiese et al, Journal of thoracic disease, 2016-08, Vol.8 (8), p.E726-E730 Core Curriculum V5 17% of conclusions not justified by results 39% of studies used the wrong analysis Parsons et al, J Bone Joint Surg Br. 2011;93-B(9):1154-1159. Core Curriculum V5 Are these two columns different? Column A Column B You have been asked by your insurance carrier 19 10 12 11 to prove that your total hip patient outcomes 10 9 20 19 are not statistically different than your competitor 10 17 14 10 next door. 21 19 24 13 Column A is the patient reported score for you 12 18 and column B for your competitor 17 8 12 17 17 10 9 9 How would you prove you are 24 10 21 9 18 18 different or better? 13 4 18 8 12 14 15 15 14 17 Core Curriculum V5 Are they still the same? Column A: Mean=15 Column B: Mean=12 SD=4 Core Curriculum V5 We want to know if we're making a difference Core Curriculum V5 What is statistics? a. -
Information-Geometric Optimization Algorithms: a Unifying Picture Via Invariance Principles
Information-Geometric Optimization Algorithms: A Unifying Picture via Invariance Principles Ludovic Arnold, Anne Auger, Nikolaus Hansen, Yann Ollivier Abstract We present a canonical way to turn any smooth parametric family of probability distributions on an arbitrary search space 푋 into a continuous-time black-box optimization method on 푋, the information- geometric optimization (IGO) method. Invariance as a major design principle keeps the number of arbitrary choices to a minimum. The resulting method conducts a natural gradient ascent using an adaptive, time-dependent transformation of the objective function, and makes no particular assumptions on the objective function to be optimized. The IGO method produces explicit IGO algorithms through time discretization. The cross-entropy method is recovered in a particular case with a large time step, and can be extended into a smoothed, parametrization-independent maximum likelihood update. When applied to specific families of distributions on discrete or con- tinuous spaces, the IGO framework allows to naturally recover versions of known algorithms. From the family of Gaussian distributions on R푑, we arrive at a version of the well-known CMA-ES algorithm. From the family of Bernoulli distributions on {0, 1}푑, we recover the seminal PBIL algorithm. From the distributions of restricted Boltzmann ma- chines, we naturally obtain a novel algorithm for discrete optimization on {0, 1}푑. The IGO method achieves, thanks to its intrinsic formulation, max- imal invariance properties: invariance under reparametrization of the search space 푋, under a change of parameters of the probability dis- tribution, and under increasing transformation of the function to be optimized. The latter is achieved thanks to an adaptive formulation of the objective.