Parallel and External High Quality Graph Partitioning
Total Page:16
File Type:pdf, Size:1020Kb
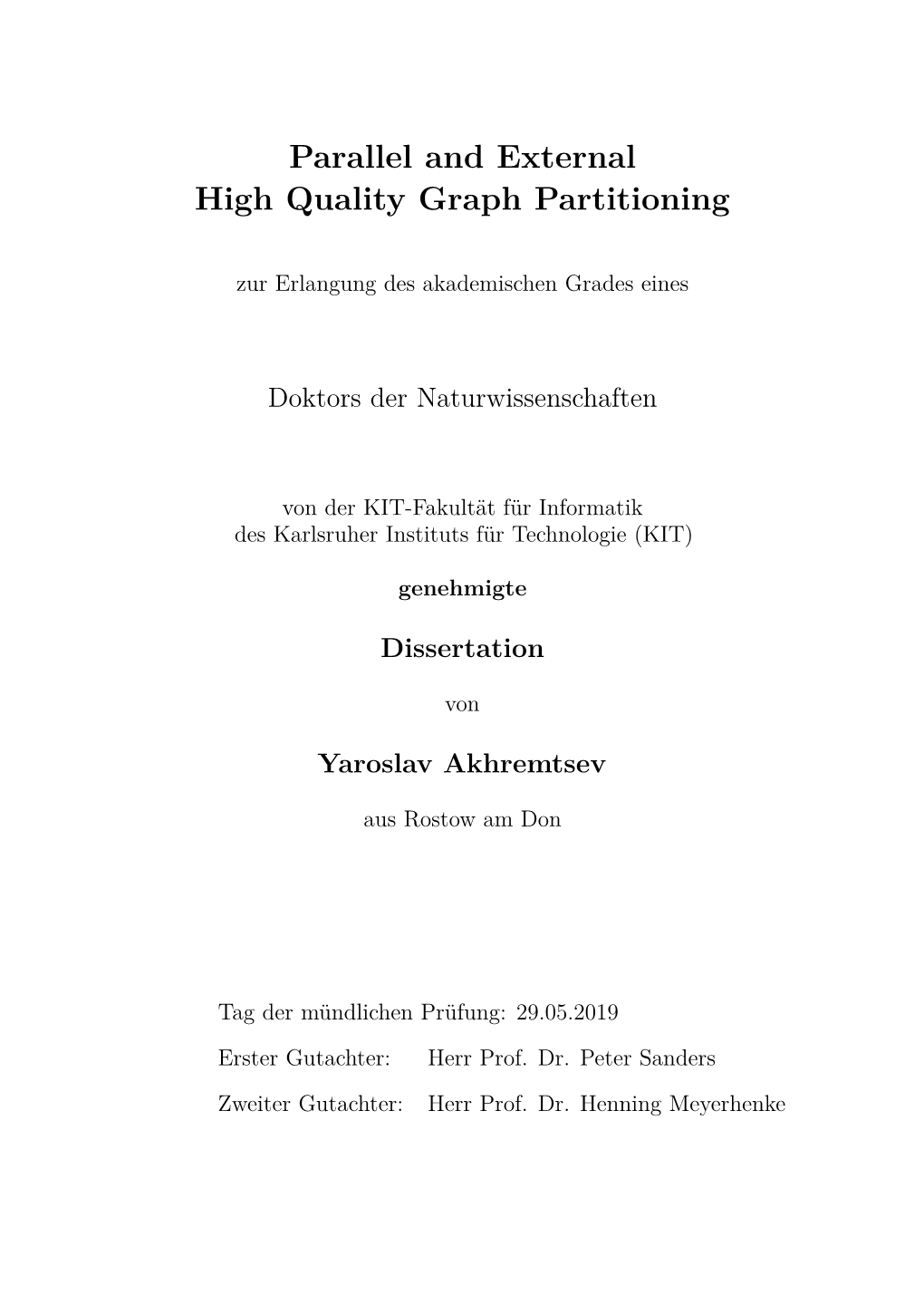
Load more
Recommended publications
-
16.6 Graph Partition 541
16.6 GRAPH PARTITION 541 INPUT OUTPUT 16.6 Graph Partition Input description: A (weighted) graph G =(V,E) and integers k and m. Problem description: Partition the vertices into m roughly equal-sized subsets such that the total edge cost spanning the subsets is at most k. Discussion: Graph partitioning arises in many divide-and-conquer algorithms, which gain their efficiency by breaking problems into equal-sized pieces such that the respective solutions can easily be reassembled. Minimizing the number of edges cut in the partition usually simplifies the task of merging. Graph partition also arises when we need to cluster the vertices into logical components. If edges link “similar” pairs of objects, the clusters remaining after partition should reflect coherent groupings. Large graphs are often partitioned into reasonable-sized pieces to improve data locality or make less cluttered drawings. Finally, graph partition is a critical step in many parallel algorithms. Consider the finite element method, which is used to compute the physical properties (such as stress and heat transfer) of geometric models. Parallelizing such calculations requires partitioning the models into equal-sized pieces whose interface is small. This is a graph-partitioning problem, since the topology of a geometric model is usually represented using a graph. Several different flavors of graph partitioning arise depending on the desired objective function: 542 16. GRAPH PROBLEMS: HARD PROBLEMS Figure 16.1: The maximum cut of a graph • Minimum cut set –Thesmallest set of edges to cut that will disconnect a graph can be efficiently found using network flow or randomized algorithms. -
Tensor Spectral Clustering for Partitioning Higher-Order Network Structures
Tensor Spectral Clustering for Partitioning Higher-order Network Structures Austin R. Benson∗ David F. Gleichy Jure Leskovecz Abstract normalized) number of first-order structures (i.e., edges) that Spectral graph theory-based methods represent an important need to be cut in order to split the graph into two parts. In a class of tools for studying the structure of networks. Spec- similar spirit, a higher-order generalization of spectral clus- tral methods are based on a first-order Markov chain de- tering would try to minimize cutting higher-order structures rived from a random walk on the graph and thus they cannot that involve multiple nodes (e.g., triangles). take advantage of important higher-order network substruc- Incorporating higher-order graph information (that is, tures such as triangles, cycles, and feed-forward loops. Here network motifs/graphlets) into the partitioning process can we propose a Tensor Spectral Clustering (TSC) algorithm significantly improve our understanding of the underlying that allows for modeling higher-order network structures in a network. For example, triangles (three-dimensional network graph partitioning framework. Our TSC algorithm allows the structures involving three nodes) have proven fundamental to user to specify which higher-order network structures (cy- understanding social networks [14, 21] and their community cles, feed-forward loops, etc.) should be preserved by the structure [10, 26, 29]. Most importantly, higher-order spec- network clustering. Higher-order network structures of in- tral clustering would allow for greater modeling flexibility terest are represented using a tensor, which we then partition as the application would drive which higher-order network by developing a multilinear spectral method. -
Preconditioned Spectral Clustering for Stochastic Block Partition
Preconditioned Spectral Clustering for Stochastic Block Partition Streaming Graph Challenge (Preliminary version at arXiv.) David Zhuzhunashvili Andrew Knyazev University of Colorado Mitsubishi Electric Research Laboratories (MERL) Boulder, Colorado 201 Broadway, 8th Floor, Cambridge, MA 02139-1955 Email: [email protected] Email: [email protected], WWW: http://www.merl.com/people/knyazev Abstract—Locally Optimal Block Preconditioned Conjugate The graph partitioning problem can be formulated in terms Gradient (LOBPCG) is demonstrated to efficiently solve eigen- of spectral graph theory, e.g., using a spectral decomposition value problems for graph Laplacians that appear in spectral of a graph Laplacian matrix, obtained from a graph adjacency clustering. For static graph partitioning, 10–20 iterations of LOBPCG without preconditioning result in ˜10x error reduction, matrix with non-negative entries that represent positive graph enough to achieve 100% correctness for all Challenge datasets edge weights describing similarities of graph vertices. Most with known truth partitions, e.g., for graphs with 5K/.1M commonly, a multi-way graph partitioning is obtained from (50K/1M) Vertices/Edges in 2 (7) seconds, compared to over approximated “low frequency eigenmodes,” i.e. eigenvectors 5,000 (30,000) seconds needed by the baseline Python code. Our corresponding to the smallest eigenvalues, of the graph Lapla- Python code 100% correctly determines 98 (160) clusters from the Challenge static graphs with 0.5M (2M) vertices in 270 (1,700) cian matrix. Alternatively and, in some cases, e.g., normalized seconds using 10GB (50GB) of memory. Our single-precision cuts, equivalently, one can operate with a properly scaled graph MATLAB code calculates the same clusters at half time and adjacency matrix, turning it into a row-stochastic matrix that memory. -
Big Graph Processing: Partitioning and Aggregated Querying
Big Graph Processing : Partitioning and Aggregated Querying Ghizlane Echbarthi To cite this version: Ghizlane Echbarthi. Big Graph Processing : Partitioning and Aggregated Querying. Databases [cs.DB]. Université de Lyon, 2017. English. NNT : 2017LYSE1225. tel-01707153 HAL Id: tel-01707153 https://tel.archives-ouvertes.fr/tel-01707153 Submitted on 12 Feb 2018 HAL is a multi-disciplinary open access L’archive ouverte pluridisciplinaire HAL, est archive for the deposit and dissemination of sci- destinée au dépôt et à la diffusion de documents entific research documents, whether they are pub- scientifiques de niveau recherche, publiés ou non, lished or not. The documents may come from émanant des établissements d’enseignement et de teaching and research institutions in France or recherche français ou étrangers, des laboratoires abroad, or from public or private research centers. publics ou privés. No d’ordre NNT : 2017LYSE1225 THESEDEDOCTORATDEL’UNIVERSIT` EDELYON´ op´er´ee au sein de l’Universit´e Claude Bernard Lyon 1 Ecole´ Doctorale ED512 Infomath Sp´ecialit´e de doctorat : Informatique Soutenue publiquement le 23/10/2017, par : Ghizlane ECHBARTHI Big Graph Processing: Partitioning and Aggregated Querying Devant le jury compos´ede: Nom Pr´enom, grade/qualit´e, ´etablissement/entreprise Pr´esident(e) Karine Zeitouni, Professeur, Universit´e de Versaille Rapporteure Raphael Couturier, Professeur, Universit´e de Franche-Comt´e Rapporteur Angela Bonifati, Professeur, Universit´e Lyon 1 Examinatrice Mohand Boughanem, Professeur, Universit´e Paul Sabatier Examinateur Hamamache Kheddouci, Professeur, Universit´e Lyon 1 Directeur de th`ese Remerciements Tout d’abord je tiens `a remercier mon directeur de th`ese Hamamache KHEDDOUCI, de m’avoir acceuilli au sein de l’´equipe GOAL, d’avoir constamment veill´e sur la qualit´e de mon travail et de m’avoir apport´e un soutien scientifique et morale d’une grande importance. -
Recent Advances in Graph Partitioning
Recent Advances in Graph Partitioning Aydın Buluç1, Henning Meyerhenke2, Ilya Safro3, Peter Sanders2, and Christian Schulz2 1 Computational Research Division, Lawrence Berkeley National Laboratory, Berkeley, USA 2 Institute of Theoretical Informatics, Karlsruhe Institute of Technology (KIT), Karlsruhe, Germany 3 School of Computing, Clemson University, Clemson SC, USA Abstract. We survey recent trends in practical algorithms for balanced graph partitioning, point to applications and discuss future research directions. 1 Introduction Graphs are frequently used by computer scientists as abstractions when modeling an appli- cation problem. Cutting a graph into smaller pieces is one of the fundamental algorithmic operations. Even if the final application concerns a different problem (such as traversal, find- ing paths, trees, and flows), partitioning large graphs is often an important subproblem for complexity reduction or parallelization. With the advent of ever larger instances in applica- tions such as scientific simulation, social networks, or road networks, graph partitioning (GP) therefore becomes more and more important, multifaceted, and challenging. The purpose of this paper is to give a structured overview of the rich literature, with a clear emphasis on explaining key ideas and discussing recent work that is missing in other overviews. For a more detailed picture on how the field has evolved previously, we refer the interested reader to a number of surveys. Bichot and Siarry [BS11] cover studies on GP within the area of numerical analysis. This includes techniques for GP, hypergraph partitioning and parallel methods. The book discusses studies from a combinatorial viewpoint as well as several ap- plications of GP such as the air traffic control problem. -
Solving the Max-Cut Problem Using Eigenvalues
DISCRETE APPLIED MATHEMATICS Discrete Applied Mathematics 62 (1995) 2499278 Solving the max-cut problem using eigenvalues Svatopluk Poljak”, Franz Rendlbq* “FakultlitJiir Mathematik und Informatik, Universitiit Passau, 94030 Passau, Germany bTechnische Universitiit Graz, Institut fir Mathematik, Kopernikusgasse 24, A-8010 Gras Austria Received 2 April 1992; revised 10 April 1994 Abstract We present computational experiments for solving the max-cut problem using an eigenvalue relaxation. Our motivation is twofold - we are interested both in the quality of the bound, and in developing an efficient code to compute it. We describe the theoretical background of the method, an implementation of the algorithm, and its practical performance. The experiments have been done for various data sets, including random graphs of different densities, clustering problems, problems arising from quadratic O-l optimization, and some graphs taken from the literature. The basic algorithm is used to compute an upper and lower bound on the max-cut. The relative gap between these bounds is typically much less than 10%. We also present results where the basic algorithm is used in a “branch and bound” setting to find the exact value of the max-cut. The largest problems solved to optimality are dense geometric graphs with up to 100 nodes. Keywords: The max-cut problem; Eigenvalues; Subdifferential optimization 1. Introduction The max-cut problem consists of finding a decomposition of vertices of a weighted undirected graph into two parts (of not necessarily equal size) such that the sum of the weights on the edges between the parts is maximum. We denote by mc(G,w) the max-cut of a graph G with weights w. -
Some Applications of Eigenvalues of Graphs
Chapter 14 Some Applications of Eigenvalues of Graphs Sebastian M. Cioaba˘ Abstract The main goal of spectral graph theory is to relate important structural properties of a graph to its eigenvalues. In this chapter, we survey some old and new applications of spectral methods in graph partitioning, ranking, epidemic spreading in networks and clustering. Keywords Eigenvalues Graph Partition Laplacian MSC2000: Primary 15A18; Secondary 68R10, 05C99 14.1 Introduction The study of eigenvalues of graphs is an important part of combinatorics. His- torically, the first relation between the spectrum and the structure of a graph was discovered in 1876 by Kirchhoff when he proved his famous matrix-tree theorem. The key principle dominating spectral graph theory is to relate important invariants of a graph to its spectrum. Often, such invariants such as chromatic number or in- dependence number, for example, are difficult to compute so comparing them with expressions involving eigenvalues is very useful. In this chapter, we present some connections between the spectrum of a graph and its structure and some applications of these connections in fields such as graph partitioning, ranking, epidemic spread- ing in networks, and clustering. For other applications of eigenvalues of graphs we recommend the surveys [44] (expander graphs), [51] (pseudorandom graphs), or [61, 62] (spectral characterization of graphs). To an undirected graph G of order n, one can associate the following matrices: The adjacency matrix A D A.G/. S.M. Cioab˘a() Department of Mathematical Sciences, University of Delaware, 501 Ewing Hall, Newark, DE 19716-2553, USA e-mail: [email protected] M. -
Sphynx: a Parallel Multi-GPU Graph Partitioner for Distributed-Memory Systems
Sphynx: a parallel multi-GPU graph partitioner for distributed-memory systems Seher Acer1,∗, Erik G. Boman1,∗, Christian A. Glusa1, Sivasankaran Rajamanickam1 Center for Computing Research, Sandia National Laboratories, Albuquerque, NM, U.S.A. Abstract Graph partitioning has been an important tool to partition the work among several processors to minimize the communication cost and balance the workload. While accelerator-based supercomputers are emerging to be the standard, the use of graph partitioning becomes even more important as applications are rapidly moving to these architectures. However, there is no distributed-memory- parallel, multi-GPU graph partitioner available for applications. We developed a spectral graph partitioner, Sphynx, using the portable, accelerator-friendly stack of the Trilinos framework. In Sphynx, we allow using different preconditioners and exploit their unique advantages. We use Sphynx to systematically evaluate the various algorithmic choices in spectral partitioning with a focus on the GPU performance. We perform those evaluations on two distinct classes of graphs: regular (such as meshes, matrices from finite element methods) and irregular (such as social networks and web graphs), and show that different settings and preconditioners are needed for these graph classes. The experimental results on the Summit supercomputer show that Sphynx is the fastest alternative on irregular graphs in an application-friendly setting and obtains a partitioning quality close to ParMETIS on regular graphs. When compared to nvGRAPH on a single GPU, Sphynx is faster and obtains better balance and better quality partitions. Sphynx provides a good and robust partitioning method across a wide range of graphs for applications looking for a GPU-based partitioner. -
Incremental Streaming Graph Partitioning
Incremental Streaming Graph Partitioning Lisa Durbeck Peter Athanas Virginia Tech Virginia Tech Dept. of ECE Dept. of ECE ldurbeck @ vt.edu athanas @ vt.edu Abstract—Graph partitioning is an NP-hard problem its contributions is a set of graphs along with vertex whose efficient approximation has long been a subject group labels with which researchers can develop of interest. The I/O bounds of contemporary computing new algorithms and validate results. This also fa- environments favor incremental or streaming graph parti- cilitates comparisons among competing approaches tioning methods. Methods have sought a balance between latency, simplicity, accuracy, and memory size. In this in terms of their computational and memory cost paper, we apply an incremental approach to streaming versus their accuracy of results. Facilitating this partitioning that tracks changes with a lightweight proxy further is a graph generator, and a baseline algorithm to trigger partitioning as the clustering error increases. and scoring functions against which researchers can We evaluate its performance on the DARPA/MIT Graph benchmark their progress, and a code base in C++ Challenge streaming stochastic block partition dataset, and or Python with which to begin. Within the larger find that it can dramatically reduce the invocation of partitioning, which can provide an order of magnitude DGC, which contains several challenges since 2017, speedup. this work focuses on the Streaming Graph Chal- Index Terms—Computation (stat.CO); Graph partition; lenge (SGC), a challenge for graph partitioning that graph sparsification; spectral graph theory; LOBPCG; includes both static and streaming datasets of syn- spectral graph partitioning; stochastic blockmodels; incre- thetic stochastic blockmodel-based (SBM) graphs. -
Graph Partitioning
Technische Universitt Chemnitz Ulrich Elsner Graph Partitioning A survey MASSIV PARALLEL M SIMULATION S SFB 393 Sonderforschungsbereich Numerische Simulation auf massiv parallelen Rechnern Preprint SFB Dec Contents Contents i Notation iii The problem Introduction Graph Partitioning Examples Partial Dierential Equations Sparse MatrixVector Multiplication Other Applications Time vs Quality Miscellaneous Algorithms Introduction Recursive Bisection Partitioning with geometric information Co ordinate Bisection Inertial Bisection Geometric Partitioning Partitioning without geometric information Introduction Graph Growing and Greedy Algorithms KerniganLin Algorithm Sp ectral Bisection Sp ectral Bisection for weighted graphs Sp ectral Quadri and Octasection Multilevel Sp ectral Bisection Algebraic Multilevel Multilevel Partitioning Coarsening the graph i Contents Partitioning the smallest graph Pro jecting up and -
Preconditioned Spectral Clustering for Stochastic Block Partition Streaming Graph Challenge
MITSUBISHI ELECTRIC RESEARCH LABORATORIES http://www.merl.com Preconditioned Spectral Clustering for Stochastic Block Partition Streaming Graph Challenge Zhuzhunashvili, D.; Knyazev, A. TR2017-131 September 25, 2017 Abstract Locally Optimal Block Preconditioned Conjugate Gradient (LOBPCG) is demonstrated to efficiently solve eigenvalue problems for graph Laplacians that appear in spectral clustering. For static graph partitioning, 10-20 iterations of LOBPCG without preconditioning result in - 10x error reduction, enough to achieve 100% correctness for all Challenge datasets with known truth partitions, e.g., for graphs with 5K/.1M (50K/1M Vertices/Edges in 2 (7) seconds, compared to over 5,000 (30,000) seconds needed by the baseline Python code. Our Python code 100% correctly determines 98 (160) clusters from the Challenge static graphs with 0.5M (2M) vertices in 270 (1,700) seconds using 10GB (50GB) of memory. Our single-precision MATLAB code calculates the same clusters at half time and memory. For streaming graph partitioning, LOBPCG is initiated with approximate eigenvectors of the graph Laplacian already computed for the previous graph, in many cases reducing 2-3 times the number of required LOBPCG iterations, compared to the static case. Our spectral clustering is generic, i.e. assuming nothing specific of the block model or streaming, used to generate the graphs for the Challenge, in contrast to the base code. Nevertheless, in 10-stage streaming comparison with the base code for the 5K graph, the quality of our clusters is similar or better starting at stage 4 (7) for emerging edging (snowballing) streaming, while the computing time is 100-1000 smaller. -
Multi-Level Spectral Graph Partitioning Method Community Detection Jianjun Cheng, Longjie Li, Mingwei Leng Et Al
Journal of Statistical Mechanics: Theory and Experiment PAPER: INTERDISCIPLINARY STATISTICAL MECHANICS Related content - A divisive spectral method for network Multi-level spectral graph partitioning method community detection Jianjun Cheng, Longjie Li, Mingwei Leng et al. To cite this article: Muhammed Fatih Talu J. Stat. Mech. (2017) 093406 - Motif-based embedding for graph clustering Sungsu Lim and Jae-Gil Lee - Complex eigenvectors of network matrices View the article online for updates and enhancements. give better insight into the communitystructure Mina Zarei, Keivan Aghababaei Samani and Gholam Reza Omidi This content was downloaded from IP address 193.140.142.195 on 20/08/2019 at 11:59 IOP Journal of Statistical Mechanics: Theory and Experiment J. Stat. Mech. 2017 ournal of Statistical Mechanics: Theory and Experiment 2017 J © 2017 IOP Publishing Ltd and SISSA Medialab srl PAPER: Interdisciplinary statistical mechanics JSMTC6 Multi-level spectral graph partitioning 093406 method M F Talu J. Stat. Mech. Mech. Stat. J. Muhammed Fatih Talu Multi-level spectral graph partitioning method Computer Science Department, Inonu University, Malatya, Turkey Printed in the UK E-mail: [email protected] Received 29 March 2017 JSTAT Accepted for publication 31 July 2017 Published 27 September 2017 10.1088/1742-5468/aa85ba Online at stacks.iop.org/JSTAT/2017/093406 https://doi.org/10.1088/1742-5468/aa85ba Abstract. In this article, a new method for multi-level and balanced division of non-directional graphs (MSGP) is introduced. Using the eigenvectors of 1742-5468 the Laplacian matrix of graphs, the method has a spectral approach which ( has superiority over local methods (Kernighan–Lin and Fiduccia-Mattheyses) 2017 with a global division ability.