About the Size of Google Scholar: Playing the Numbers
Total Page:16
File Type:pdf, Size:1020Kb
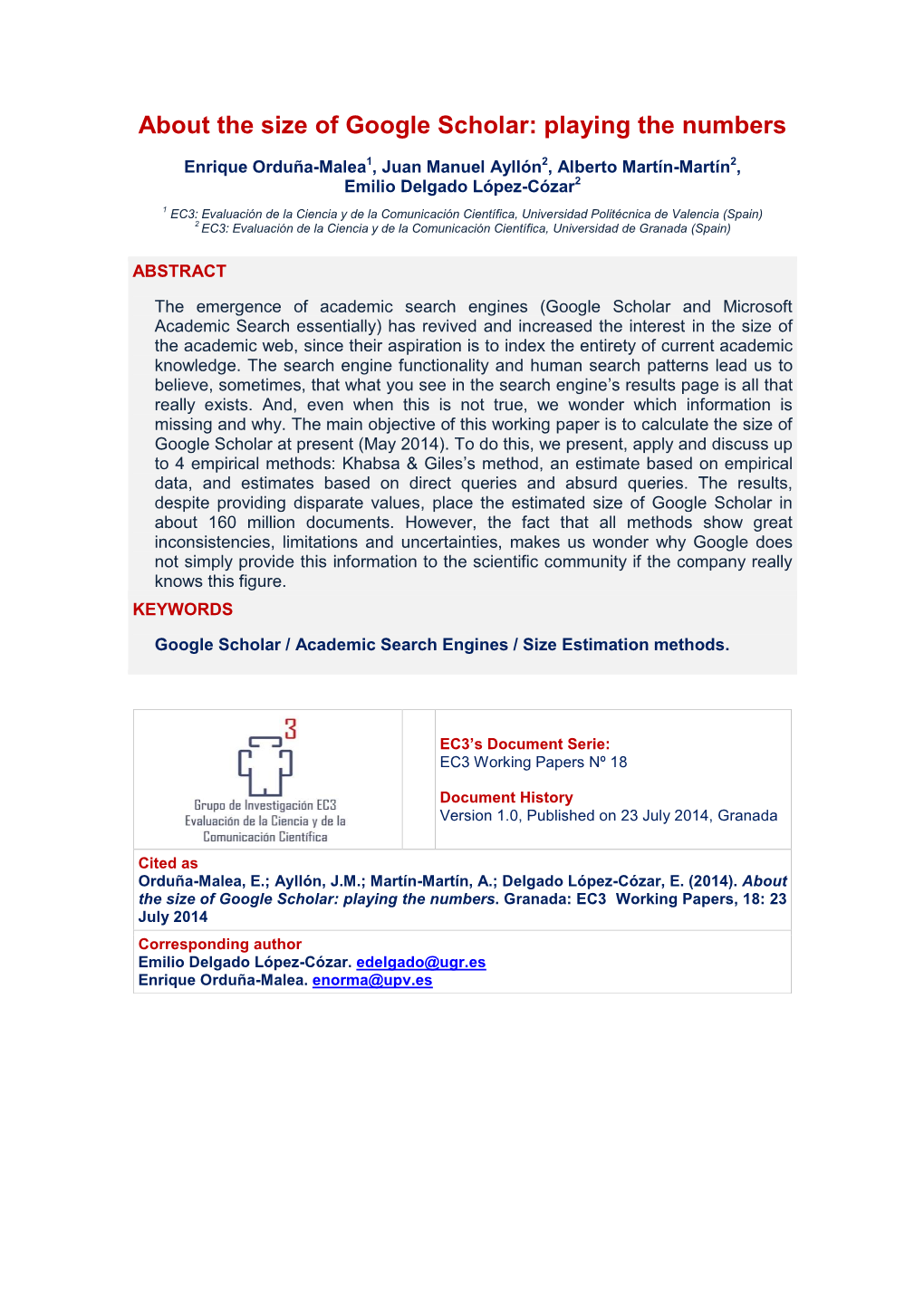
Load more
Recommended publications
-
Google Apps: an Introduction to Docs, Scholar, and Maps
[Not for Circulation] Google Apps: An Introduction to Docs, Scholar, and Maps This document provides an introduction to using three Google Applications: Google Docs, Google Scholar, and Google Maps. Each application is free to use, some just simply require the creation of a Google Account, also at no charge. Creating a Google Account To create a Google Account, 1. Go to http://www.google.com/. 2. At the top of the screen, select “Gmail”. 3. On the Gmail homepage, click on the right of the screen on the button that is labeled “Create an account”. 4. In order to create an account, you will be asked to fill out information, including choosing a Login name which will serve as your [email protected], as well as a password. After completing all the information, click “I accept. Create my account.” at the bottom of the page. 5. After you successfully fill out all required information, your account will be created. Click on the “Show me my account” button which will direct you to your Gmail homepage. Google Docs Now that you have an account created with Google, you are able to begin working with Google Docs. Google Docs is an application that allows the creation and sharing of documents, spreadsheets, presentations, forms, and drawings online. Users can collaborate with others to make changes to the same document. 1. Type in the address www.docs.google.com, or go to Google’s homepage, http://www. google.com, click the more drop down tab at the top, and then click Documents. Information Technology Services, UIS 1 [Not for Circulation] 2. -
The Informal Sector and Economic Growth of South Africa and Nigeria: a Comparative Systematic Review
Journal of Open Innovation: Technology, Market, and Complexity Review The Informal Sector and Economic Growth of South Africa and Nigeria: A Comparative Systematic Review Ernest Etim and Olawande Daramola * Department of Information Technology, Cape Peninsula University of Technology, P.O. Box 652, South Africa; [email protected] * Correspondence: [email protected] Received: 17 August 2020; Accepted: 10 October 2020; Published: 6 November 2020 Abstract: The informal sector is an integral part of several sub-Saharan African (SSA) countries and plays a key role in the economic growth of these countries. This article used a comparative systematic review to explore the factors that act as drivers to informality in South Africa (SA) and Nigeria, the challenges that impede the growth dynamics of the informal sector, the dominant subsectors, and policy initiatives targeting informal sector providers. A systematic search of Google Scholar, Scopus, ResearchGate was performed together with secondary data collated from grey literature. Using Boolean string search protocols facilitated the elucidation of research questions (RQs) raised in this study. An inclusion and exclusion criteria became necessary for rigour, comprehensiveness and limitation of publication bias. The data collated from thirty-one (31) primary studies (17 for SA and 14 for Nigeria) revealed that unemployment, income disparity among citizens, excessive tax burdens, excessive bureaucratic hurdles from government, inflationary tendencies, poor corruption control, GDP per capita, and lack of social protection survival tendencies all act as drivers to the informal sector in SA and Nigeria. Several challenges are given for both economies and policy incentives that might help sustain and improve the informal sector in these two countries. -
4 Google Scholar-Books
Scholarship Tools: Google Scholar Eccles Health Sciences Library NANOS 2010 What is Google Scholar? Google Scholar provides a simple way to broadly search for scholarly literature. From one place, you can search across many disciplines and sources: articles, theses, books, abstracts and court opinions, from academic publishers, professional societies, online repositories, universities and other web sites. Google Scholar helps you find relevant work across the world of scholarly research. Features of Google Scholar • Search diverse sources from one convenient place • Find articles, theses, books, abstracts or court opinions • Locate the complete document through your library or on the web • Cited by links you to abstracts of papers where the article has been cited • Related Articles links you to articles on similar topics Searching Google Scholar You can do a simple keyword search as is done in Google, or you can refine your search using the Advanced Search option. Advanced Search allows you t o limit by author, journal, date and collections. Results are ranked by weighing the full text of the article, where it is published, who it is written by and how often it has been n cited in other scholarly journals. Google Scholar Library Links Find an interesting abstract or citation that you wish you could read? In many cases you may have access to the complet e document through your library. You can set your preferences so that Google Scholar knows your primary library. Once this is set, it will determine whether your library can supply the article in Google Scholar preferences. You may need to be on campus or logged into a VPN or a Proxy server to get the full text of the article, even if it is in your library. -
Social Factors Associated with Chronic Non-Communicable
BMJ Open: first published as 10.1136/bmjopen-2019-035590 on 28 June 2020. Downloaded from PEER REVIEW HISTORY BMJ Open publishes all reviews undertaken for accepted manuscripts. Reviewers are asked to complete a checklist review form (http://bmjopen.bmj.com/site/about/resources/checklist.pdf) and are provided with free text boxes to elaborate on their assessment. These free text comments are reproduced below. ARTICLE DETAILS TITLE (PROVISIONAL) Social factors associated with chronic non-communicable disease and comorbidity with mental health problems in India: a scoping review AUTHORS M D, Saju; Benny, Anuja; Scaria, Lorane; Anjana, Nannatt; Fendt- Newlin, Meredith; Joubert, Jacques; Joubert, Lynette; Webber, Martin VERSION 1 – REVIEW REVIEWER Hoan Linh Banh University of Alberta, Canada REVIEW RETURNED 28-Nov-2019 GENERAL COMMENTS There is a major flaw with the search strategy. The authors did not include important databases such as PsycInfo and the Educational Resources Information Centre. Also, pubmed should have been used rather than medline. Finally, the authors did not even attempt to search google scholar for grey literature. The the study only included 10 papers and 6 were on multiple countries which was one of the exclusion critera. http://bmjopen.bmj.com/ REVIEWER Graham Thornicroft KCL, UK REVIEW RETURNED 06-Dec-2019 GENERAL COMMENTS MJ Open: Social factors associated with chronic non-communicable disease and comorbidity with mental health problems in India: a scoping review on October 2, 2021 by guest. Protected copyright. The aim of this paper is to examine the existing literature of the major social risk factors which are associated with diabetes, hypertension and the co- morbid conditions of depression and anxiety in India. -
Assessment of the Registration of Bibliographical Data in the ESS Bibliography Database
Assessment of the registration of bibliographical data in the ESS Bibliography database Professor Birger Larsen Aalborg University [email protected] August 2018 1 Table of Contents 1 Introduction ............................................................................................................................................... 4 2 Methodology ............................................................................................................................................. 4 3 Background - ESS and the ESSB ................................................................................................................. 4 4 Analysis ...................................................................................................................................................... 5 4.1 Current procedures of registering data to the ESSB ......................................................................... 5 4.1.1 Self-reporting by ESS users ........................................................................................................ 7 4.1.2 Identification of Google Scholar publications at the University of Ljubljana .......................... 11 4.1.3 Bibliographic control ............................................................................................................... 13 4.2 Current presentation of ESS bibliographical data and proposed improvements ........................... 14 4.3 Possibilities for bibliometric analyses ............................................................................................. -
A Content Analysis in the Studies of Youtube in Selected Journals
Available online at www.sciencedirect.com ScienceDirect Procedia - Social and Behavioral Sciences 103 ( 2013 ) 10 – 18 13th International Educational Technology Conference A content analysis in the studies of YouTube in selected journals Norlidah Alias*, Siti Hajar Abd Razak, Ghada elHadad, Nurul Rabihah Mat Noh Kokila Kunjambu, Parimaladevi Muniandy Department of Curriculum & Instructional Technology, Faculty of Education, University of Malaya, 50603 Kuala Lumpur, Malaysia [email protected], [email protected], [email protected], [email protected], [email protected], [email protected] Abstract This paper provides a review of research trends and content analysis of studies in the field of YouTube that were published in seven major journals: Turkish Online Journal of Educational Technology (TOJET), Educational Technology & Society (ET&S), Educational Technology Research & Development (ETR&D), Computers & Education (C&E), Learning and Instruction (L&I), Australasian Journal of Educational Technology (AJET) and British Journal of Educational Technology (BJET). These articles were cross analyzed by published years. Content analysis was implemented for further analysis based on their research topics, issues category, research settings and sampling, research design, research method and data analysis. The results of the analysis also provide insights for educators and researchers into research trends and issues related to YouTube. © 20132013 The The Authors. Authors. Published Published by byElsevier Elsevier Ltd. Ltd. Selection and and peer-review peer-review under under responsibility responsibility of The of AssociationThe Association of Science, of Science, Education Education and Technology-TASET, and Technology-TASET, Sakarya Sakarya Universitesi, Turkey. Turkey. Keywords: YouTube; trends; content analysis. * Corresponding author. -
AN INTRODUCTION to GOOGLE SCHOLAR Randy L
AN INTRODUCTION TO GOOGLE SCHOLAR Randy L. Miller Graduate Research Assistance Librarian September 2013 434-592-3096 [email protected] What does Google Scholar include? Google Scholar www.scholar.google.com provides a simple way to broadly search for scholarly literature. From one place, you can search across many disciplines and sources: articles, theses, books, abstracts and court opinions, from academic publishers, professional societies, online repositories, universities and other web sites. Google Scholar helps you find relevant work across the world of scholarly research. (Important note: If you are doing a comprehensive review of the literature, you should always check other sources such as Summon and discipline-specific databases rather than relying solely on Google Scholar.) Google Scholar only contains citation references to books, journal articles, and other resources, not general websites like the Google Search engine. It is more complete in the STEM (science, technology, engineering, and medicine) literature. It is also fairly comprehensive in the Social Sciences (such as Education and Counseling). Has the fewest indexed articles in the Humanities, including Religion and Biblical Studies. (See the “Metrics” link at the top to show the major disciplines and the most highly indexed journals in each discipline.) It also tends to include more recent literature rather than pre-1990 literature because this older literature has often never been digitized and put on the web. Although it contains patent records, court cases, and legal documents, we will not be discussing those in this handout. What are some advantages of using Google Scholar? 1. In addition to showing resources like journal articles in our subscription databases, it also shows free “open access” and gray literature items (like conference proceedings, organization white papers, etc.) found on the web. -
Decrease in Free Computer Science Papers Found Through Google Scholar Lee A
Virginia Commonwealth University VCU Scholars Compass VCU Libraries Faculty and Staff ubP lications VCU Libraries 2014 Decrease in Free Computer Science Papers Found through Google Scholar Lee A. Pedersen Brown University, [email protected] Julie Arendt Virginia Commonwealth University, [email protected] Follow this and additional works at: http://scholarscompass.vcu.edu/libraries_pubs Part of the Databases and Information Systems Commons, and the Library and Information Science Commons Copyright Emerald Group Publishing Recommended Citation Lee A. Pedersen, Julie Arendt, (2014) "Decrease in free computer science papers found through Google Scholar", Online Information Review, Vol. 38 Iss: 3, pp.348 - 361. This Article is brought to you for free and open access by the VCU Libraries at VCU Scholars Compass. It has been accepted for inclusion in VCU Libraries Faculty and Staff ubP lications by an authorized administrator of VCU Scholars Compass. For more information, please contact [email protected]. Decrease in free computer science papers found through Google Scholar Abstract: Purpose - Google Scholar was used to locate free full-text versions of computer science research papers to determine what proportion could be freely accessed. Design/methodology/approach - A sample of 1967 conference papers and periodical articles from 2003-2010, indexed in the ACM Guide to Computing Literature, was searched for manually in Google Scholar, using the paper or article title and the first author’s surname and supplementary searches as needed. Findings - Free full-text versions were found for 52% of the conference papers and 55% of the periodical articles. Documents with older publication dates were more likely to be freely accessible than newer documents, with free full-text versions found for 71% of items published in 2003 and 43% of items published 2010. -
Ten-Year Analysis of Hepatitis-Related Papers in the Middle East
Turk J Gastroenterol 2017; 28: 20-5 Liver Ten-year analysis of hepatitis-related papers in the Middle East: a web of science-based scientometric study Mohammad Saeid Rezaee-Zavareh1,2,3, Seyed Moayed Alavian2,3 1Student Research Committee, Baqiyatallah University of Medical Sciences, Tehran, Iran 2Baqiyatallah Research Center for Gastroenterology and Liver Diseases (BRCGL), Baqiyatallah University of Medical Sciences, Tehran, Iran 3Scientometrics Institute of Middle East Liver Diseases (MELD) Center, Tehran, IR Iran ABSTRACT Background/Aims: In the Middle East (ME), the proper understanding of hepatitis, especially viral hepatitis, is considered to be extremely important. However, no published paper has investigated the status of hepatitis- related research in the ME. A scientometric analysis based on the Web of Science database was conducted on hepatitis-related papers in the ME to determine the current status of research on this topic. Original Article Materials and Methods: A scientometric analysis using the Web of Science database, specifically articles from the Expanded Science Citation Index and Social Sciences Citation Index, was conducted on work published between 2005 and 2014 using the keyword “hepatitis” in conjunction with the names of countries in the ME. Results: Of 103,096 papers that used the word “hepatitis” in their title, abstract, or keywords, only 6,540 papers (6.34%) were associated with countries in the ME. Turkey, Iran, Egypt, Israel, and Saudi Arabia were the top five countries in which hepatitis-related papers were published. Most papers on hepatitis A, B, and D and autoim- mune hepatitis were published in Turkey, and most papers on hepatitis C were published in Egypt. -
Effects of Scientific Collaboration Between Domestic and Foreign Authors on Quality Indices of Journals
Webology, Volume 15, Number 2, December, 2018 Home Table of Contents Titles & Subject Index Authors Index Effects of Scientific Collaboration between Domestic and Foreign Authors on Quality Indices of Journals Masoud Mohammadi Department of Medical Library & Information Sciences, Faculty of Allied Medical Sciences, Tehran University of Medical Sciences, Tehran, Iran. Golestan University of Medical Sciences, Gorgan, Iran. ORCID. E-mail: [email protected] Mohsen Mansouri Department of Computer engineering, Islamic Azad University, Gorgan Branch, Gorgan, Iran. E-mail: [email protected] Mojtaba Azghandi Shahri Central library, Research and Technology Deputy, Gonabad University of Medical Sciences, Gonabad, Iran. E-mail: [email protected] Fatemeh Sheikhshoaei Faculty of Allied Medical Science, Tehran University of Medical Sciences, Tehran, Iran. ORCID. E-mail: [email protected] Auwal Abdullahi Abubakar Department of Library and Information Science, School of Continuing Education, Bayero University Kano, Nigeria. E-mail: [email protected] Morvarid Daemi Golestan University of Medical Sciences, Gorgan, Iran. E-mail: [email protected] Maryam Banisafar* *Corresponding author, Golestan University of Medical Sciences, Gorgan, Iran. E-mail: [email protected] Received June 12, 2018; Accepted November 25, 2018 133 http://www.webology.org/2018/v15n2/a176.pdf Abstract The aim of the study was to determine the effect of scientific collaboration between domestic (Iranian) and foreign authors on their quality indices in articles published in Iranian medical journals, indexed in the Web of Science citation database. The present scientometric study was conducted on the 22 Iranian medical journals that indexed in Web of Science from 2010 to 2016. -
The Political Economy of Youtube
You Tube as an cultural form Wasko & Erickson – The Political Economy of YouTube Janet Wasko and Mary Erickson Political Economy of Communication The discussion in this article draws on a political economic analy- sis of media. The study of political economy is about how societies are organized and controlled and it is very much about the analysis of power. The Political In the 1970s, Graham Murdock and Peter Golding defined political econ- omy of communication as fundamentally interested in studying commu- nication and media as commodities produced by capitalist industries.4 More recent theoretical discussions of this approach have been offered Economy of 5 by Vincent Mosco, Robert McChesney, Janet Wasko and others. How- ever, in general, the study of political economy of the media is about YouTube how the media are organized and controlled within the larger political economy. In other words, it is concerned with who has power to make decisions about the media, and who benefits from these decisions. In Since YouTube was launched in 2005, many have heralded the video- other words, it is about understanding how power relations actually sharing website as a democratizing media platform that would convert work within and around the media. media consumers into producers and reshape the entire landscape of In media studies, critical political economy has grown over the years media. Some argue that the site is contributing to a fundamental trans- and is now recognized as a distinct tradition. While critical political econ- formation in political discourse and policy too.1 From its very early days, omy does not claim to explain everything, political economists have corporate organizations have heralded YouTube as a potential goldmine examined a wide range of communication and media issues and practic- of relatively effortless profit, where millions (and soon to be billions) of es, including the traditional mass media, and more recently, computers eyeballs would translate into huge revenues for YouTube, venture capi- and information technologies. -
Transforming Health Care Big Data Implementing Through Aprior-Mapreduce E
et International Journal on Emerging Technologies 10 (2): 71-77(2019) ISSN No. (Print) : 0975-8364 ISSN No. (Online) : 2249-3255 Transforming Health Care Big Data Implementing through Aprior-Mapreduce E. Laxmi Lydia 1, R. Pandi Selvam 2, U.S. Kirutikaa 3, R. Saranya 4, M. Ilayaraja 5, K. Shankar 6 and Andino Maseleno 7 1Associate Professor, Vignan’s Institute of Information Technology(A), Department of Computer Science and Engineering, Visakhapatnam, Andhra Pradesh, India. 2Assistant Professor & Head, P.G. Department of Computer Science, Ananda College, Devakottai, India. 3Assistant Professor, Department of Information Technology, Dr. Umayal Ramanathan College For Women, Karaikudi, India. 4Assistant Professor, Department of Computer Science, Dr. Umayal Ramanathan College for Women, Karaikudi, India. 5School of Computing Kalasalingam Academy of Research and Education, Krishnankoil, India. 6School of Computing Kalasalingam Academy of Research and Education, Krishnankoil, India. 7Institute of Informatics and Computing Energy, Universiti Tenaga Nasional, Malaysia. (Corresponding author: E. Laxmi Lydia, [email protected] ) (Received 03 April 2019, Revised 25 June 2019 Accepted 08 July 2019) (Published by Research Trend, Website: www.researchtrend.net) ABSTRACT: The healthcare industry routinely has generated massive quantities of knowledge, pushed by way of using record preserving, compliance & regulatory specifications, and patient care. The proposed paper specializes in designing of the radical Framework which consists of components and these accessories can be utilized to perform quick analysis by way of connecting clusters which is designed in this work. The proposed paper implements BigData Analytics capabilities as a part of a “Novel Framework” structure that can meet the wants of Health care vendors searching for to strengthen effects and efficiencies at the same time increasing profitability.