The Role of Empirical Study in Software Engineering
Total Page:16
File Type:pdf, Size:1020Kb
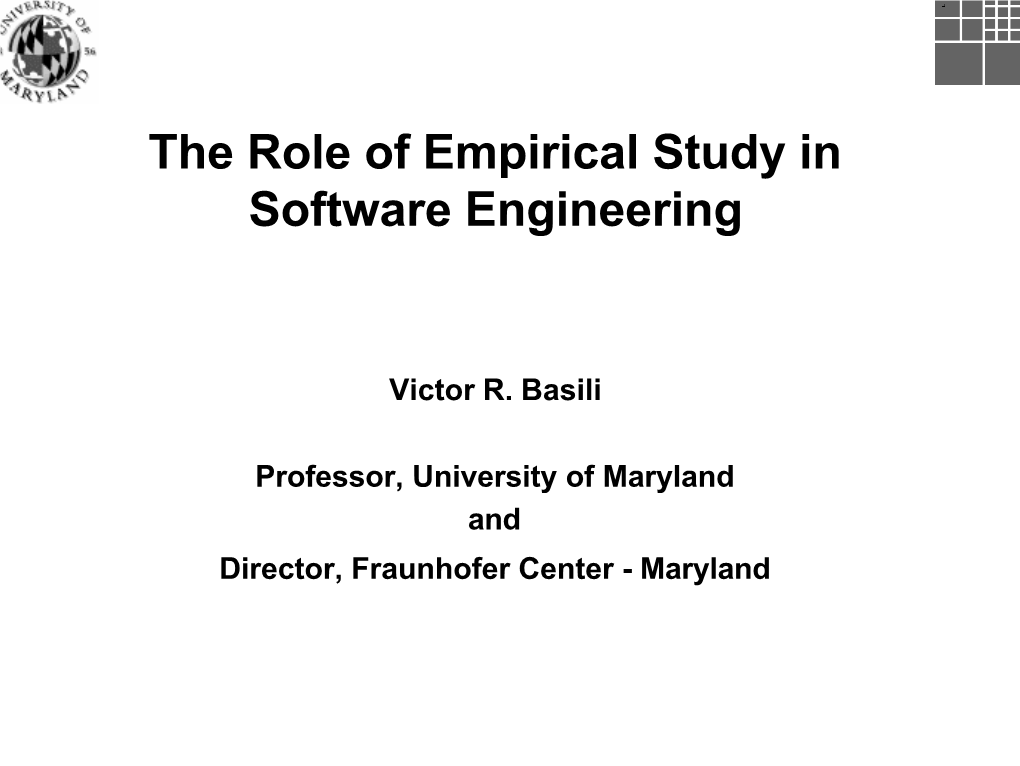
Load more
Recommended publications
-
Rugby - a Process Model for Continuous Software Engineering
INSTITUT FUR¨ INFORMATIK DER TECHNISCHEN UNIVERSITAT¨ MUNCHEN¨ Forschungs- und Lehreinheit I Angewandte Softwaretechnik Rugby - A Process Model for Continuous Software Engineering Stephan Tobias Krusche Vollstandiger¨ Abdruck der von der Fakultat¨ fur¨ Informatik der Technischen Universitat¨ Munchen¨ zur Erlangung des akademischen Grades eines Doktors der Naturwissenschaften (Dr. rer. nat.) genehmigten Dissertation. Vorsitzender: Univ.-Prof. Dr. Helmut Seidl Prufer¨ der Dissertation: 1. Univ.-Prof. Bernd Brugge,¨ Ph.D. 2. Prof. Dr. Jurgen¨ Borstler,¨ Blekinge Institute of Technology, Karlskrona, Schweden Die Dissertation wurde am 28.01.2016 bei der Technischen Universitat¨ Munchen¨ eingereicht und durch die Fakultat¨ fur¨ Informatik am 29.02.2016 angenommen. Abstract Software is developed in increasingly dynamic environments. Organizations need the capability to deal with uncertainty and to react to unexpected changes in require- ments and technologies. Agile methods already improve the flexibility towards changes and with the emergence of continuous delivery, regular feedback loops have become possible. The abilities to maintain high code quality through reviews, to regularly re- lease software, and to collect and prioritize user feedback, are necessary for con- tinuous software engineering. However, there exists no uniform process model that handles the increasing number of reviews, releases and feedback reports. In this dissertation, we describe Rugby, a process model for continuous software en- gineering that is based on a meta model, which treats development activities as parallel workflows and which allows tailoring, customization and extension. Rugby includes a change model and treats changes as events that activate workflows. It integrates re- view management, release management, and feedback management as workflows. As a consequence, Rugby handles the increasing number of reviews, releases and feedback and at the same time decreases their size and effort. -
The Legacy of Victor R. Basili
University of Tennessee, Knoxville TRACE: Tennessee Research and Creative Exchange About Harlan D. Mills Science Alliance 2005 Foundations of Empirical Software Engineering: The Legacy of Victor R. Basili Barry Boehm Hans Dieter Rombach Marvin V. Zelkowitz Follow this and additional works at: https://trace.tennessee.edu/utk_harlanabout Part of the Physical Sciences and Mathematics Commons Recommended Citation Boehm, Barry; Rombach, Hans Dieter; and Zelkowitz, Marvin V., "Foundations of Empirical Software Engineering: The Legacy of Victor R. Basili" (2005). About Harlan D. Mills. https://trace.tennessee.edu/utk_harlanabout/3 This Book is brought to you for free and open access by the Science Alliance at TRACE: Tennessee Research and Creative Exchange. It has been accepted for inclusion in About Harlan D. Mills by an authorized administrator of TRACE: Tennessee Research and Creative Exchange. For more information, please contact [email protected]. University of Tennessee, Knoxville Trace: Tennessee Research and Creative Exchange The Harlan D. Mills Collection Science Alliance 1-1-2005 Foundations of Empirical Software Engineering: The Legacy of Victor R.Basili Barry Boehm Hans Dieter Rombach Marvin V. Zelkowitz Recommended Citation Boehm, Barry; Rombach, Hans Dieter; and Zelkowitz, Marvin V., "Foundations of Empirical Software Engineering: The Legacy of Victor R.Basili" (2005). The Harlan D. Mills Collection. http://trace.tennessee.edu/utk_harlan/36 This Book is brought to you for free and open access by the Science Alliance at Trace: Tennessee Research and Creative Exchange. It has been accepted for inclusion in The Harlan D. Mills Collection by an authorized administrator of Trace: Tennessee Research and Creative Exchange. For more information, please contact [email protected]. -
The Role of Empirical Study in Software Engineering
The Role of Empirical Study in Software Engineering Victor R. Basili Professor, University of Maryland and Director, Fraunhofer Center - Maryland © 2004 Experimental Software Engineering Group, University of Maryland Outline • Empirical Studies – Motivation – Specific Methods – Example: SEL • Applications – CeBASE – NASA High Dependability Computing Project – The Future Combat Systems Project – DoE High Productivity Computing System 2 Motivation for Empirical Software Engineering Understanding a discipline involves building models, e.g., application domain, problem solving processes And checking our understanding is correct, e.g., testing our models, experimenting in the real world Analyzing the results involves learning, the encapsulation of knowledge and the ability to change or refine our models over time The understanding of a discipline evolves over time This is the empirical paradigm that has been used in many fields, e.g., physics, medicine, manufacturing Like other disciplines, software engineering requires an empirical paradigm 3 Motivation for Empirical Software Engineering Empirical software engineering requires the scientific use of quantitative and qualitative data to understand and improve the software product, software development process and software management It requires real world laboratories Research needs laboratories to observe & manipulate the variables - they only exist where developers build software systems Development needs to understand how to build systems better - research can provide models to help Research -
Utilizing GQM+Strategies for Business Value Analysis
Utilizing GQM+Strategies for Business Value Analysis An Approach for Evaluating Business Goals Vladimir Mandic´ Victor Basili Lasse Harjumaa Department of Information Fraunhofer Center for Department of Information Processing Science, Experimental Software Processing Science, University of Oulu Engineering - Maryland University of Oulu Finland USA Finland [email protected].fi [email protected] lasse.harjumaa@oulu.fi Markku Oivo Jouni Markkula Department of Information Department of Information Processing Science, Processing Science, University of Oulu University of Oulu Finland Finland markku.oivo@oulu.fi jouni.markkula@oulu.fi ABSTRACT to define it. The general understanding is that business value is Business value analysis (BVA) quantifies the factors that provide a concept which extends the traditional bookkeeping-like defini- value and cost to an organization. It aims at capturing value, con- tion of the value by including different aspects of the business as trolling risks, and capitalizing on opportunities. GQM+Strategies well. Those different aspects of business (e.g., employees, part- is an approach designed to aid in the definition and alignment of ner networks, ability to adopt new processes rapidly, etc.) form an business goals, strategies, and an integrated measurement program endless list that is under continuous amendment. A rich financial at all levels in the organization. In this paper we describe how apparatus for evaluating business investments (e.g., return on in- to perform business value analysis (BVA) using the GQM+Strate- vestment (ROI), net present value (NPV), portfolio management, gies approach. The integration of these two approaches provides a etc.) is powerless if we are not able to define inputs (components coupling of cost-benefit and risk analysis (value goals) with oper- of the business value). -
Victor Basili Adam Trendowicz Martin Kowalczyk Jens Heidrich Carolyn
The Fraunhofer IESE Series on Software and Systems Engineering Victor Basili Adam Trendowicz Martin Kowalczyk Jens Heidrich Carolyn Seaman Jürgen Münch Dieter Rombach Aligning Organizations Through Measurement The GQM+Strategies Approach The Fraunhofer IESE Series on Software and Systems Engineering Series Editor Dieter Rombach Peter Liggesmeyer Editorial Board W. Rance Cleaveland II Reinhold E. Achatz Helmut Krcmar For further volumes: http://www.springer.com/series/8755 ThiS is a FM Blank Page Victor Basili • Adam Trendowicz • Martin Kowalczyk • Jens Heidrich • Carolyn Seaman • Ju¨rgen Mu¨nch • Dieter Rombach Aligning Organizations Through Measurement The GQM+Strategies Approach Victor Basili Adam Trendowicz Department of Computer Science Jens Heidrich University of Maryland Dieter Rombach College Park Fraunhofer Institute for Experimental Maryland Software Engineering USA Kaiserslautern Germany Martin Kowalczyk Carolyn Seaman Department of Information Systems Department of Information Systems Technical University of Darmstadt University of Maryland Baltimore County Darmstadt Baltimore Germany USA Ju¨rgen Mu¨nch Department of Computer Science University of Helsinki Helsinki Finland ISSN 2193-8199 ISSN 2193-8202 (electronic) ISBN 978-3-319-05046-1 ISBN 978-3-319-05047-8 (eBook) DOI 10.1007/978-3-319-05047-8 Springer Cham Heidelberg New York Dordrecht London Library of Congress Control Number: 2014936596 # Springer International Publishing Switzerland 2014 This work is subject to copyright. All rights are reserved by the Publisher, whether the whole or part of the material is concerned, specifically the rights of translation, reprinting, reuse of illustrations, recitation, broadcasting, reproduction on microfilms or in any other physical way, and transmission or information storage and retrieval, electronic adaptation, computer software, or by similar or dissimilar methodology now known or hereafter developed. -
Arxiv:1609.04886V1 [Cs.SE] 16 Sep 2016 William Nichols, Forrest Shull Software Engineering Institute , Carnegie Mellon University, USA
Noname manuscript No. (will be inserted by the editor) Are Delayed Issues Harder to Resolve? Revisiting Cost-to-Fix of Defects throughout the Lifecycle Tim Menzies, William Nichols, Forrest Shull, Lucas Layman Abstract Many practitioners and academics believe in a delayed issue effect (DIE); i.e. the longer an issue lingers in the system, the more effort it requires to resolve. This belief is often used to justify major investments in new development processes that promise to retire more issues sooner. This paper tests for the delayed issue effect in 171 software projects conducted around the world in the period from 2006–2014. To the best of our knowledge, this is the largest study yet published on this effect. We found no evidence for the delayed issue effect; i.e. the effort to resolve issues in a later phase was not consistently or substantially greater than when issues were resolved soon after their introduction. This paper documents the above study and explores reasons for this mismatch between this common rule of thumb and empirical data. In summary, DIE is not some constant across all projects. Rather, DIE might be an historical relic that occurs intermittently only in certain kinds of projects. This is a significant result since it predicts that new development processes that promise to faster retire more issues will not have a guaranteed return on investment (depending on the context where applied), and that a long-held truth in software engineering should not be considered a global truism. Categories/Subject Descriptors: D.2.8 [Software Engineering]: Process metrics. -
A Study of Design Pattern Grime and Its Impact on Quality and Technical
DESIGN PATTERN DECAY { A STUDY OF DESIGN PATTERN GRIME AND ITS IMPACT ON QUALITY AND TECHNICAL DEBT by Isaac Daniel Griffith A dissertation proposal submitted in partial fulfillment of the requirements for the degree of Doctor of Philosophy in Computer Science MONTANA STATE UNIVERSITY Bozeman, Montana October, 2015 ©COPYRIGHT by Isaac Daniel Griffith 2015 All Rights Reserved ii TABLE OF CONTENTS 1. INTRODUCTION ........................................................................................1 Motivation....................................................................................................1 Organization.................................................................................................3 2. BACKGROUND AND RELATED WORK.....................................................4 Software Aging and Decay.............................................................................4 Design Disharmonies .................................................................................4 Code Smells ..........................................................................................4 Anti-Patterns ........................................................................................5 Modularity Violations ............................................................................5 Design Pattern Disharmonies..................................................................5 Design Disharmony Detection ....................................................................8 Design Disharmonies and Quality............................................................ -
Achieving CMMI Level 5 Improvements with MBASE and the Cebase Method Dr
Achieving CMMI Level 5 Improvements with MBASE and the CeBASE Method Dr. Barry Boehm, Dr. Daniel Port, and Apurva Jain Dr. Victor Basili University of Southern California University of Maryland Each branch of service in the Department of Defense has major initiatives to pursue more advanced software-intensive systems concepts involving network-centric warfare with self- adaptive networks and cooperating human and autonomous agents. The ability to balance discipline and flexibility is critically important to developing such highly dependable soft- ware-intensive systems in an environment of rapid change. Risk-management orientation enables users of Capability Maturity Model® IntegrationSM (CMMISM) to apply risk con- Tuesday, 30 April 2002 siderations to determine how much discipline and how much flexibility is enough in a given Track 1: 1:00 - 1:40 situation. The risk-driven nature of the spiral model and MBASE enables them to achieve Ballroom A a similar balance of discipline and flexibility. When these project-level approaches are com- bined with the organization-level approaches in the Experience Factory, the result is the unified Center for Empirically Based Software Engineering (CeBASE) method described in this article. ecent events in Afghanistan have con- cific techniques for achieving and maintain- In “Using the Spiral Model and MBASE Rvincingly demonstrated the value of ing the right balance of discipline and flexi- to Generate New Acquisition Process software and information technology in bility for a particular program’s evolving sit- Models: SAIV, CAIV and SCQAIV [3],” we achieving military superiority. Each of the uation. showed how programs could use MBASE Department of Defense (DoD) services has In our previous three CrossTalk arti- risk management techniques to avoid many major initiatives to pursue even more cles, we provided some specific methods overruns of fixed schedules and budgets. -
Towards a Reliability Prediction Model Based on Internal Structure and Post-Release Defects Using Neural Networks
Towards a Reliability Prediction Model based on Internal Structure and Post-Release Defects Using Neural Networks Andreea Vescan Camelia Şerban Alisa Budur Babes-Bolyai University Babes-Bolyai University Babes-Bolyai University Cluj-Napoca, Cluj, Romania Cluj-Napoca, Cluj, Romania Cluj-Napoca, Cluj, Romania [email protected] [email protected] [email protected] ABSTRACT 1 INTRODUCTION Reliability is one of the most important quality attributes of a soft- One of the factors that affects the software development process ware system, addressing the system’s ability to perform the required resides in the quality pressure. DevOps help to increase the deploy- functionalities under stated conditions, for a stated period of time. ment speed with high software quality. Several studies investigate Nowadays, a system failure could threaten the safety of human life. the links between DevOps and software quality [19], [24], some Thus, assessing reliability became one of the software engineer- revealed that there is a need for further research in the area of mea- ing‘s holy grails. Our approach wants to establish based on what surement and proposing metrics for various development stages project’s characteristics we obtain the best bug-oriented reliability to assess software performance. Thus, there is a positive relation prediction model. The pillars on which we base our approach are between DevOps’s practices and software quality, i.e. quality is the metric introduced to estimate one aspect of reliability using increased when practice DevOps by following CAMS (Culture, Au- bugs, and the Chidamber and Kemerer (CK) metrics to assess relia- tomation, Measurement, Sharing) framework. -
Understanding and Predicting the Process of Software Maintenance Releases*
Technical Report, University of Maryland, Computer Science Dept., College Park, MD 20770 USA. Aug. 1995. Understanding and Predicting the Process of Software Maintenance Releases* Victor Basili, Lionel Briand, Steven Condon, Yong-Mi Kim, Walcélio L. Melo and Jon D. Valett** To appear in the Proc. of the 18th Int l Conf. on S/W Eng., Berlin, Germany, March 1996. Abstract One of the major concerns of any maintenance organization is to understand and estimate the cost of maintenance releases of software systems. Planning the next release so as to maximize the increase in functionality and the improvement in quality are vital to successful maintenance management. The objective of this paper is to present the results of a case study in which an incremental approach was used to better understand the effort distribution of releases and build a predictive effort model for software maintenance releases. This study was conducted in the Flight Dynamics Division (FDD) of NASA Goddard Space Flight Center (GSFC). This organization is representative of many other software maintenance organizations. Over one hundred software systems totaling about 4.5 million lines of code, are maintained by this organization. Many of these systems are maintained for many years and regularly produce new releases. This paper presents three main results: 1) a predictive effort model developed for the FDD’s software maintenance release process, 2) measurement-based lessons learned about the maintenance process in the FDD, 3) a set of lessons learned about the establishment of a measurement-based software maintenance improvement program. In addition, this study provides insights and guidelines to obtain similar results in other maintenance organizations. -
Empirical Software Engineering at Microsoft Research
Software Analytics Harlan D. Mills Award Acceptance Speech Nachi Nagappan © Microsoft Corporation About Me • My name is Nachiappan. I also go by Nachi. • https://nachinagappan.github.io/ • Graduated with a PhD with Laurie Williams. • I read a lot of Franco-Belgian comics (Bande dessinées) • Attend Comic conventions • Miniature railroad modeling (HO and G). © Microsoft Corporation 3 © Microsoft Corporation 4 © Microsoft Corporation Courtney Miller Jenna Butler Danielle Gonzalez Rangeet Pan Yu Huang Jazette Johnson Paige Rodeghero Rini Chattopadhyay 5 © Microsoft Corporation Courtney Miller Jenna Butler Danielle Gonzalez Rangeet Pan Yu Huang Jazette Johnson Paige Rodeghero Rini Chattopadhyay 6 © Microsoft Corporation What metrics are the If I increase test coverage, will that best predictors of failures? actually increase software quality? What is the data quality level Are there any metrics that are indicators of used in empirical studies and failures in both Open Source and Commercial how much does it actually domains? matter? I just submitted a bug report. Will it be fixed? Should I be writing unit tests in my software How can I tell if a piece of software will have vulnerabilities? project? Is strong code ownership good or Do cross-cutting concerns bad for software quality? cause defects? Does Distributed/Global software Does Test Driven Development (TDD) development affect quality? produce better code in shorter time? 7 © Microsoft Corporation History of Software Analytics 1976: Thomas McCabe code complexity 1971: Fumio Akiyama 1981: -
Protocols in the Use of Empirical Software Engineering Artifacts
Empir Software Eng DOI 10.1007/s10664-006-9030-4 Protocols in the use of empirical software engineering artifacts Victor R. Basili & Marvin V. Zelkowitz & Dag I. K. Sjøberg & Philip Johnson & Anthony J. Cowling # Springer Science + Business Media, LLC 2006 Editor: Lionel Briand Abstract If empirical software engineering is to grow as a valid scientific endeavor, the ability to acquire, use, share, and compare data collected from a variety of sources must be encouraged. This is necessary to validate the formal models being developed within computer science. However, within the empirical software engineering community this has not been easily accomplished. This paper analyses experiences from a number of projects, and defines the issues, which include the following: (1) How should data, testbeds, and artifacts be shared? (2) What limits should be placed on who can use them and how? How does one limit potential misuse? (3) What is the appropriate way to credit the organization and individual that spent the effort collecting the data, developing the testbed, and building the artifact? (4) Once shared, who owns the evolved asset? As a solution to these issues, the paper proposes a framework for an empirical software engineering artifact agreement. Such an agreement is intended to address the needs for both creator and user of such artifacts and should foster a market in making available and using such artifacts. If this framework for V. R. Basili : M. V. Zelkowitz (*) Department Of Computer Science, University of Maryland, AV Williams Building, College Park, MD 20742, USA e-mail: [email protected] V. R. Basili e-mail: [email protected] V.