Sensitivity of Structural Demand to Ground Motion Selection and Modification Methods
Total Page:16
File Type:pdf, Size:1020Kb
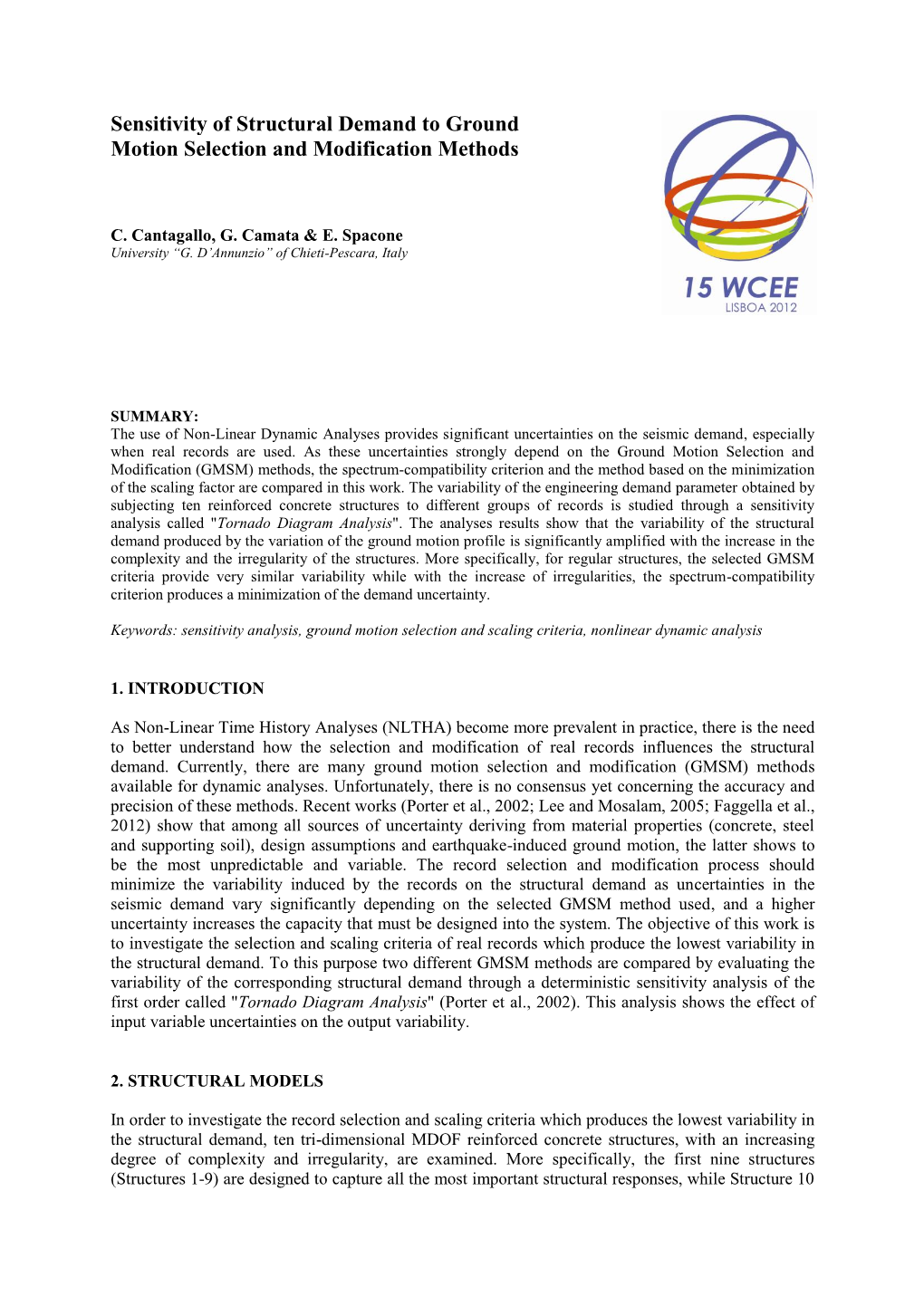
Load more
Recommended publications
-
Supplementary Online Content Chahal HS, Marseille EA, Tice JA, Et Al
Supplementary Online Content Chahal HS, Marseille EA, Tice JA, et al. Cost-effectiveness of early treatment of hepatitis C virus genotype 1 by stage of liver fibrosis in a US treatment-naive population. JAMA Intern Med. Published online November 23, 2015. doi:10.1001/jamainternmed.2015.6011. eMethods. eTable 1. METAVIR Fibrosis Score, Treatment Policies for Evaluation and Modeled Treatment Options eTable 2. Model Comparison Using Sim/Sof and Sof/R Treatment Regimens eTable 3. Distribution of Fibrosis Stages in Chronic Hepatitis C Population eTable 4. Chronic Hepatitis C Natural History Disease Progression, Post-SVR Progression, and Regression and Mortality eTable 5. Weekly Cost of Drugs for the Modeled Therapies eTable 6. Chronic Hepatitis C Health Care Costs by Disease State eTable 7. Other Health Care–Related Costs: Follow-up, Testing, and Management of Treatment eTable 8. Frequency, by Week, of Follow-up/Testing/Management of Each Treatment Modality eTable 9. Total Cost of Treatment-Associated Adverse Events eTable 10. Health State Utilities in Chronic Hepatitis C eTable 11. Utility Loss With Chronic Hepatitis C Treatment eTable 12. SVR and Treatment Discontinuation Rates of All Modeled Therapies, Based on Meta-analyses of Clinical Trials eTable 13. Base-Case Results: Treatment by Fibrosis Stage and Treat All vs Treat at F3/F4 Strategies, for All Treatment Options eTable 14. Long-term Health Outcomes With Treatment at an Earlier Fibrosis Stage (or Treat All) vs Treating at a Later Fibrosis Stage (or Treating at F3/F4): Number of Advanced Liver Disease Cases per 100 000 Treated Patients eTable 15. Budget Impact, in Total Drug and Health Care Costs, of Therapies: Treating All vs Treating at F3/F4 eTable 16. -
Effect of Spatial Variability of Soil Properties on the Seismic Response of Earth Dams
Effect of Spatial Variability of Soil Properties on the Seismic Response of Earth Dams H. Sanchez Lizarraga EUCENTRE, European Centre for Training and Research in Earthquake Engineering, Italy C.G. Lai University of Pavia, and EUCENTRE, European Centre for Training and Research in Earthquake Engineering, Italy SUMMARY: Variability of soil properties is a major source of uncertainty in assessing the seismic response of geotechnical systems. This study presents a probabilistic methodology to evaluate the seismic response of earth dams. A sensitivity analysis is performed by means of Tornado diagrams. Two-dimensional, anisotropic, cross-correlated random fields are generated based on a specific marginal distribution function, auto-correlation, and cross- correlation coefficient. Nonlinear time-history analyses are then performed using an advanced finite difference software (FLAC 2D). The study is performed using Monte Carlo simulations that allowed to estimate the mean and the standard deviation of the maximum crest settlement. The statistical response is compared with results of a deterministic analysis in which the soil is assumed homogeneous. This research will provide insight into the implementation of stochastic analyses of geotechnical systems, illustrating the importance of considering the spatial variability of soil properties when analyzing earth dams subjected to earthquake loading. Keywords: Earth Dams, Spatial Variability, Cross Correlated Random Field, Seismic Response. 1. INTRODUCTION It is well known that soil properties vary in space even within otherwise homogeneous layers. This spatial variability is highly dependent on soil type or the method of soil deposition or geological formation. Nevertheless, many geotechnical analyses adopt a deterministic approach based on a single set of soil parameters applied to each distinct layer. -
An Anatomy of Data Visualization
IJCSNS International Journal of Computer Science and Network Security, VOL.16 No.2, February 2016 77 An Anatomy of Data Visualization Abhishek Kaushik† and Sudhanshu Naithani††, Kiel University of Applied Sciences† Kurukshetra University †† Summary As data is being generated each and every time in the world, the importance of data mining and visualization will always be on increase. Mining helps to extract significant insight from large volume of data. After that we need to present that data in such a way so that it can be understood by everyone and for that visualization is used. Most common way to visualize data is chart and table. Visualization is playing important role in decision making process for industry. Visualization makes better utilization of human eyes to assist his brain so that datasets can be analyzed and visual presentation can be prepared. Visualization and Data Mining works as complement for each other. Here in this paper we present anatomy of Visualization process. Key words: Information Visualization, Scientific Visualization, Decision Making, Graph, Chart, Xmdv tool. Fig.1. General steps in the process of Visualization. 1. Introduction a) Try to understand size and cardinality of the data In simple worlds Visualization is a process to form a given. picture in order to make it easily imaginable and b) Determine kind of information which is to understandable for other people. With Visualization, communicate. process of Data Mining and Human Computer Interaction c) Process visual information according to targeted provides better results for visual data analysis. Initially audience. visualization was of two types - Information Visualization d) Use the visual portraying best and easiest form of and Scientific Visualization. -
Stochastic One-Way Sensitivity Analysis Christopher Mccabe1, Mike Paulden2, Isaac Awotwe3, Andrew Sutton1, Peter Hall4
Stochastic One-Way Sensitivity Analysis Christopher McCabe1, Mike Paulden2, Isaac Awotwe3, Andrew Sutton1, Peter Hall4 1Institute of Health Economics, Alberta Canada 2Department of Emergency Medicine, University of Alberta, Canada 3School of Public Health, University of Alberta 4Department of Oncology, University of Edinburgh, United Kingdom Introduction •Using decision analytic modelling as part of cost effectiveness analysis allows us to: •Facilitate use of multiple sources of data •Go beyond scope of single-clinical trial in terms of: •Patient group •Interventions •Time horizon Introduction •Need to consider uncertainty in parameter values, how these impact on conclusions drawn from a modelling analysis •Sensitivity analysis: •is the process of varying model input values and recording the impact of those changes on the model outputs •A deterministic one-way sensitivity analysis - varies the value of the parameter of interest whilst holding all other parameters constant at their expected value. 1-Way Sensitivity Analysis Tornado Diagram -60000 -40000 -20000 0 20000 40000 60000 80000 100000 120000 Loco-regional recurrence Distant recurrence Mortality after distant recurrence Cost of Treatment Adverse Event on Chemotherapy Utility in Remission Utility in distant recurrent Cost in remission Cost of surgery Peri-surgical utility Issues with this approach: •Probability that parameter takes extreme values not considered •Correlation between parameters also not considered Stochastic One-Way Sensitivity Analysis • The analysis of the impact of varying the value of one parameter on the expected cost effectiveness that: – incorporates the probability that the parameter will take that value; – and – respects the correlation between that parameter and other parameters in the model Stochastic one-way sensitivity analysis Calculating SOWSA 1. -
Literature Review of Visual Representation of the Results of Benefit–Risk Assessments of Medicinal Products†
pharmacoepidemiology and drug safety 2016; 25: 238–250 Published online 2 November 2015 in Wiley Online Library (wileyonlinelibrary.com) DOI: 10.1002/pds.3880 REVIEW Literature review of visual representation of the results of benefit–risk assessments of medicinal products† Christine E. Hallgreen1*, Shahrul Mt-Isa1, Alfons Lieftucht2, Lawrence D. Phillips3, Diana Hughes4, Susan Talbot5, Alex Asiimwe6, Gerald Downey5, Georgy Genov7, Richard Hermann8, Rebecca Noel9, Ruth Peters1, Alain Micaleff10, Ioanna Tzoulaki1, Deborah Ashby1 and On behalf of PROTECT Benefit–Risk group 1School of Public Health, Imperial College London, London, UK 2GlaxoSmithKline UK, Middlesex, UK 3Department of Management, London School of Economics and Political Science, London, UK 4Pfizer, New York, NY, USA 5Amgen Limited, Uxbridge, UK 6Bayer Pharma AG, Berlin, Germany 7European Medicines Agency, London, UK 8AstraZeneca LP, Wilmington, DE, USA 9Eli Lilly and Company, Lilly Corporate Center, Indianapolis, IN, USA 10MerckSerono International SA, Geneva, Switzerland ABSTRACT Background The PROTECT Benefit–Risk group is dedicated to research in methods for continuous benefit–risk monitoring of medicines, including the presentation of the results, with a particular emphasis on graphical methods. Methods A comprehensive review was performed to identify visuals used for medical risk and benefit–risk communication. The identified visual displays were grouped into visual types, and each visual type was appraised based on five criteria: intended audience, intended mes- sage, knowledge required to understand the visual, unintentional messages that may be derived from the visual and missing information that may be needed to understand the visual. Results Sixty-six examples of visual formats were identified from the literature and classified into 14 visual types. -
Application of Decision Analysis to Milling Profit Maximisation: an Introduction
See discussions, stats, and author profiles for this publication at: https://www.researchgate.net/publication/228368950 Application of decision analysis to milling profit maximisation: An introduction Article in International Journal of Materials and Product Technology · May 2009 DOI: 10.1504/IJMPT.2009.025220 CITATIONS READS 10 127 6 authors, including: Raul Zapata Tony L. Schmitz United States Navy University of North Carolina at Charlotte 20 PUBLICATIONS 81 CITATIONS 254 PUBLICATIONS 2,714 CITATIONS SEE PROFILE SEE PROFILE All content following this page was uploaded by Tony L. Schmitz on 21 May 2014. The user has requested enhancement of the downloaded file. 64 Int. J. Materials and Product Technology, Vol. 35, Nos. 1-2, 2009 ) Application of decision analysis to milling profit maximisation: an introduction A.E. Abbas and L. Yang Department of Industrial and Enterprise Systems Engineering, University of Illinois at Urbana-Champaign, 117 Transportation Building, MC-238, Urbana, IL 61801, USA E-mail: [email protected] E-mail: [email protected] R. Zapata and T.L. Schmitz* Department of Mechanical and Aerospace Engineering, University of Florida, 237 MAE-B, Gainesville, FL 32611, USA E-mail: [email protected] E-mail: [email protected] *Corresponding author Abstract: This paper describes the application of decision analysis to milling optimisation. In this initial study we include the effects of uncertainty in tool life and force model coefficients. The decisions represent the milling operating parameters. A single-attribute value function is used to maximise profit. Stability of the milling operation, surface location error, and surface roughness pose constraints on the feasible decision alternatives. -
Trimming the UCERF2 Hazard Logic Tree
Trimming the UCERF2 Hazard Logic Tree a) b) c) Keith Porter , Edward Field , and Kevin Milner The Uniform California Earthquake Rupture Forecast version 2.0 (UCERF2) has 480 branches on its logic tree of 9 modeling decisions, often called epistemic uncertainties. In combination with 4 next-generation attenuation (NGA) relationships, UCERF2 has 1,920 branches. This study examines the sensitivity of a statewide risk metric to each epistemic uncertainty. The metric is expected annualized loss (EAL) to an estimated statewide portfolio of single-family woodframe dwellings. The results are depicted in a so-called tornado diagram. The uncertainties that matter most to this risk metric are the recurrence model, aperiodocity under a Brownian-passage-time recurrence model, and the choice of ground-motion-prediction equation. Risk is much less sensitive to the other uncertainties. One implication is that a statewide risk calculation employing only 5 branches of the UCERF2 tree and 4 NGA relationships captures virtually all the uncertainty produced by the full complement of 1,920 branches, allowing for a 100x reduction in computational effort with no corresponding underestimation of uncertainty. Another implication is that efforts to reduce these two epistemic uncertainties might be the most cost-effective way of reducing uncertainty in statewide risk resulting from UCERF2 and ground-motion-prediction equations. INTRODUCTION Assessing which sources of hazard uncertainty matter. The Uniform California Earthquake Rupture Forecast 2 (UCERF 2) is a fully time-dependent hazard model developed under the sponsorship of the California Earthquake Authority and delivered in September, 2007 (WGCEP 2007; Field et al. 2009). UCERF 2 contains 480 logic-tree branches reflecting choices among 9 modeling uncertainties or adjustable parameters in the earthquake rate model shown in Figure 1. -
Graphs in the LINGO Modeling Language LINDO Systems
Graphs in the LINGO Modeling Language LINDO Systems www.lindo.com LINGO is a general purpose algebraic language for describing and solving optimization problems. It also has extensive graphing/charting capabilities for describing the results of your analysis. Graphs in LINGO Both your audience and you can more quickly understand the results of your optimization analysis if you use graphs/charts. LINGO provides over a dozen different graph types to help you easily describe the results of your optimization analysis. Here are examples of the half dozen more popular types. In LINGO, graphs/plots/charts are called “charts” for short. Simple calls to all chart types are illustrated in the LINGO directory in: Samples¥Charts.lg4 Graphs in LINGO In the following we illustrate: + Function plots of one variable: @CHARTPCURVE + Function and contour plots of two variables: @CHARTPSURFACE , @CHARTPCONTOUR + Histograms: @CHARTHISTO + Bar charts: @CHARTBAR + Networks: @CHARTNETNODE + Space-Time diagrams: @CHARTSPACETIME + Trade-off curves, tabulated: @CHARTCURVE + Tornado diagrams: @CHARTTORNADO + Scatter charts: @CHARTSCATTER All the examples here can be found in the MODELS library at www.lindo.com. Click on: HELP -> MODELS Library -> Alphabetical index. Which Chart Types are Available? In LINGO Click on: Edit -> Paste Function -> Charting Plots of Functions in LINGO In finance, the “Student t ” distribution, because it has “fatter tails” than the Normal, is sometimes recommended as better than the Normal as a model of the randomness of financial returns from a stock investment. A graph or chart is a good way of showing the difference. The following little LINGO program illustrates: a) how to generate a chart/plot/graph of a function, b) the wide range of probability distributions available in LINGO, c) how to use a procedure or function to record a computation that will be used repeatedly. -
Literature Review of Visual Representation of the Results of Benefit–Risk Assessments of Medicinal Products
David Hughes, S. Talbot, S. Mt-Isa, Alfons Lieftucht, L.D. Phillips, Alex Asiimwe, C. E. Hallgreen, G. Downey, G. Genov, Richard Hermann, M.A. Metcalf, R.A. Noel, I. Tzoulaki, Deborah Ashby and Alain Micaleff Literature review of visual representation of the results of benefit–risk assessments of medicinal products Article (Accepted version) (Refereed) Original citation: Hughes, David, Talbot, S., Mt-Isa, S., Lieftucht, Alfons, Phillips, L.D., Asiimwe, Alex, Hallgreen, C. E., Downey, G., Genov, G., Hermann, Richard, Metcalf, M.A., Noel, R.A., Tzoulaki, I., Ashby, Deborah and Micaleff, Alain (2016) Literature review of visual representation of the results of benefit–risk assessments of medicinal products. Pharmacoepidemiology and Drug Safety, 25 (3). pp. 238-250. ISSN 1053-8569 DOI: 10.1002/pds.3880 © 2016 John Wiley & Sons This version available at: http://eprints.lse.ac.uk/66151/ Available in LSE Research Online: April 2016 LSE has developed LSE Research Online so that users may access research output of the School. Copyright © and Moral Rights for the papers on this site are retained by the individual authors and/or other copyright owners. Users may download and/or print one copy of any article(s) in LSE Research Online to facilitate their private study or for non-commercial research. You may not engage in further distribution of the material or use it for any profit-making activities or any commercial gain. You may freely distribute the URL (http://eprints.lse.ac.uk) of the LSE Research Online website. This document is the author’s final accepted version of the journal article. -
Cost-Effectiveness Analysis
This work is licensed under a Creative Commons Attribution-NonCommercial-ShareAlike License. Your use of this material constitutes acceptance of that license and the conditions of use of materials on this site. Copyright 2007, The Johns Hopkins University and Kevin Frick. All rights reserved. Use of these materials permitted only in accordance with license rights granted. Materials provided “AS IS”; no representations or warranties provided. User assumes all responsibility for use, and all liability related thereto, and must independently review all materials for accuracy and efficacy. May contain materials owned by others. User is responsible for obtaining permissions for use from third parties as needed. SENSITIVITY ANALYSIS AND INFERENCE Lecture 12 Points of Discussion 1. Univariate Sensitivity Analyses 2. Tornado Diagrams (many univariate) 3. Bivariate/multivariate sensitivity analyses 4. How to think about confidence intervals 5. How to calculate/interpret confidence intervals with primary data (bootstrapping) 6. How to calculate/interpret confidence intervals with simulations (probabilistic SA) 7. How to combine 5 and 6 8. Cost-effectiveness acceptability curves 9. How to transform back to something that looks more like a net benefit measure Sensitivity analysis • Simple definition – Vary a ‘critical component’ of the calculation by a meaningful amount •Objective – To determine whether results are insensitive to substantial but plausible variation in a parameter – To judge the robustness of conclusions – To indicate where more detailed -
Mt-Isa Et Al, Review of Visualisation Methods for the Representation Of
Pharmacoepidemiological Research on Outcomes of Therapeutics by a European ConsorTium Review of visualisation methods for the representation of benefit-risk assessment of medication: Stage 2 of 2 Shahrul Mt-Isa,1 Christine E. Hallgreen,2 Alex Asiimwe,3 Gerry Downey,4 Georgy Genov,5 Richard Hermann,6 Diana Hughes,7 Alfons Lieftucht,8 Rebecca Noel,3 Ruth Peters,1 Lawrence D. Phillips,5 Susan Shepherd,4 Alain Micaleff,9 Deborah Ashby,1 Ioanna Tzoulaki.1 On behalf of the PROTECT Work Package 5 participants 1 School of Public Health, Imperial College London, London, United Kingdom 2 Novo Nordisk A/S, Søborg, Denmark 3 Eli Lilly, Surrey, UK / Eli Lilly and Company, USA 4 Amgen Limited, Uxbridge, United Kingdom 5 European Medicines Agency, London, United Kingdom 6 AstraZeneca AB, Macclesfield, United Kingdom 7 Pfizer Inc., New York, USA 8 GlaxoSmithKline Research and Development LTD, Middlesex, United Kingdom 9 Merck KGaA, Geneva, Switzerland Version 1 Date: 15 Feb 2013 Date of any subsequent amendments Person making below amendments Brief description of amendments 09 Apr 2013 S Mt-Isa Added a reference (Beyer et al., 2013) Disclaimer: The processes described and conclusions drawn from the work presented herein relate solely to the testing of methodologies and representations for the evaluation of benefit and risk of medicines. This report neither replaces nor is intended to replace or comment on any regulatory decisions made by national regulatory agencies, nor the European Medicines Agency Acknowledgements: The research leading to these results was conducted as part of the PROTECT consortium (Pharmacoepidemiological Research on Outcomes of Therapeutics by a European ConsorTium, www.imi- protect.eu) which is a public-private partnership coordinated by the European Medicines Agency. -
Picture As Pdf PDF Version
The Natural Capital Project March 2017 NATURAL CAPITAL DATA DISPLAY A toolbox to synthesize and visualize multiple complex ecosystem services model outputs Author Supervised by Charlotte Gisèle Weil Dr Benjamin P.Bryant [email protected] [email protected] CONTENTS Contents 1 1 Introduction 3 1.1 How to read this document ..................................... 3 1.2 Background & motivation ...................................... 4 1.2.1 Ecosystem services & natural capital assessments ................... 4 1.2.2 Communicating natural capital information....................... 4 1.3 Objectives & outline.......................................... 5 1.4 Assessment of the quality of a visualization............................ 5 1.5 Acronyms & Terminology....................................... 6 2 Elements of data visualization8 2.1 Notions and techniques in data visualization........................... 8 2.1.1 Information visualization and graphical integrity.................... 8 2.1.2 Vocabulary and grammar of graphics........................... 9 2.1.3 Modes of visualization.................................... 9 2.1.4 Distortion techniques.................................... 10 2.1.5 Interaction techniques ................................... 10 2.2 Graphical best practices and guidelines.............................. 11 2.2.1 Legibility and intuitiveness................................. 11 2.2.2 Scale and ratios........................................ 11 2.2.3 Legend............................................. 12 2.2.4 Color scheme........................................