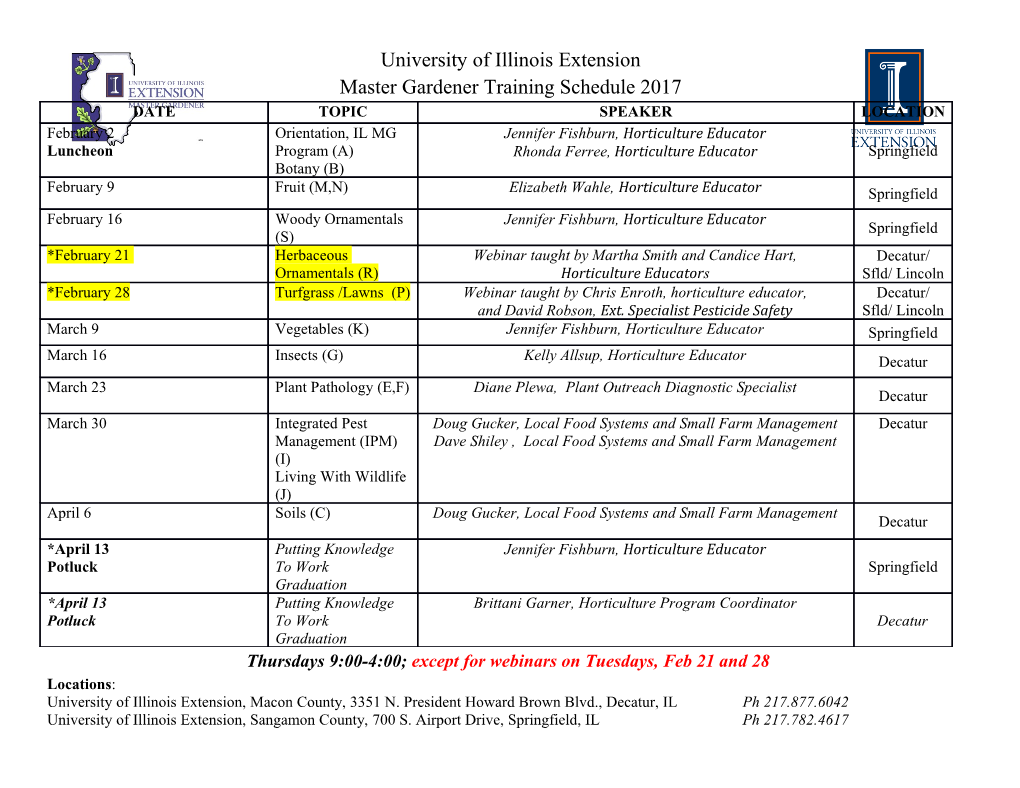
Entropy power inequalities The American Institute of Mathematics The following compilation of participant contributions is only intended as a lead-in to the AIM workshop “Entropy power inequalities.” This material is not for public distribution. Corrections and new material are welcomed and can be sent to [email protected] Version: Fri Apr 28 17:41:04 2017 1 2 Table of Contents A.ParticipantContributions . 3 1. Anantharam, Venkatachalam 2. Bobkov, Sergey 3. Bustin, Ronit 4. Han, Guangyue 5. Jog, Varun 6. Johnson, Oliver 7. Kagan, Abram 8. Livshyts, Galyna 9. Madiman, Mokshay 10. Nayar, Piotr 11. Tkocz, Tomasz 3 Chapter A: Participant Contributions A.1 Anantharam, Venkatachalam EPIs for discrete random variables. Analogs of the EPI for intrinsic volumes. Evolution of the differential entropy under the heat equation. A.2 Bobkov, Sergey Together with Arnaud Marsliglietti we have been interested in extensions of the EPI to the more general R´enyi entropies. For a random vector X in Rn with density f, R´enyi’s entropy of order α> 0 is defined as − 2 n(α−1) α Nα(X)= f(x) dx , Z 2 which in the limit as α 1 becomes the entropy power N(x) = exp n f(x)log f(x) dx . However, the extension→ of the usual EPI cannot be of the form − R Nα(X + Y ) Nα(X)+ Nα(Y ), ≥ since the latter turns out to be false in general (even when X is normal and Y is nearly normal). Therefore, some modifications have to be done. As a natural variant, we consider proper powers of Nα. A.3 Bustin, Ronit Our interest lies in the relationship between information theory and estimation theory. The connection between these two fields goes back to the 1950’s, however it has gained increasing attention in recent years since the fundamental result of Guo, Shamai and Verdu (2005), a.k.a the I-MMSE relationship. The result of Guo, Shamai and Verdu has been used to provide a simple alternative proofs of known entropy power inequalities (Guo and Verdu 2006, Guo, Shamai and Verdu, 2006) and by that have shown the strength of this connection. Since the Guo, Shamai and Verdu result in 2005, the I-MMSE relationship has been extended in different direction, including different noise channels. We are interested whether these extensions can also lead to extensions of entropy power type inequalities. Specifically, we are concerned with two families of noise distributions: the α-stable family and the Gen- eralized Gaussian family which are dual to one another and on which we have recently gain many new and interesting results. Another interesting direction which is also connected is the “single crossing point” property, which in essence shows the advantage of the Gaussian input distribution in the additive white Gaussian noise (AWGN) channel over alternative input distributions. This property has also been used to provide proofs of a special case of the EPI when one of the inputs is Gaussian. We are trying to examine alternative proofs of this property and whether they, again, can be extended to alternative channel models. A.4 Han, Guangyue Consider the following additive channel Y = X + Z, where X, Y, Z are the channel input, output and noise, respectively. If the independence between X and Z is assumed, then the classical entropy power inequality holds, which, upon imposing the extra condition that 4 Z is Gaussian, can be strengthened to Costa’s entropy power inequality. We are interested in further extending the entropy power inequality to the scenarios that memory, feedback or interference may be present in the channel, that is, the extension of the entropy power inequality to the following channel, n−1 n−1 Yn = X(W1 ,Y1 )+ Zn,n =1, 2,..., where W can be interpreted as memory or interference. The same question can be asked in continuous time for the following channel, t s s Y (t)= X(s,W0 ,Y0 )ds + B(t), Z0 where B is the standard Brownion motion. Note that some sort of “normalization” is needed in continuous time since the entropy of continuous-time processes is typically infinite. A.5 Jog, Varun In recent years, the topic of entropy power inequalities has significantly broadened in scope to encompass inequalities in additive combinatorics, convex geometry, optimal trans- port, and other areas. Shannon’s entropy power inequality (EPI), stated in its traditional form, provided a lower bound for the entropy power of the convolution of two densities as the sum of the entropy powers of the individual densities. Many generalizations of the EPI arise by considering random variables taking values in different, possibly discrete spaces, and reinterpreting the operation of convolution and the entropy power functional. Despite their differing forms, most EPIs capture the basic idea that randomness (interpreted via an appropriate notion of entropy power) is super-additive with respect to adding random variables (where addition is defined appropriately). Shannon’s EPI also states that Gaussian random variables achieve equality, and thus generalizations of EPIs also provide analogs of Gaussians obtained by examining equality cases. One of my areas of interest is the topic of discrete entropy power inequalities. To make sense of adding random variables, it is convenient to think of random variables taking values in a discrete group. In an earlier work [jogananth2014] we studied the case of random variables taking values on a group of size 2n, and realized that the key to understanding the behavior of entropy on this group was to understand how entropy behaves with respect to addition on the group of size 2. This observation lead us to believe that groups of order pn for a prime p can be effectively studied by examining the group of order p. Furthermore, since every finite abelian group is isomorphic to a direct sum of groups with prime (or prime power) order, it is likely that we may be able to characterize the EPI for all finite groups via this approach. For infinite groups, the most natural candidate to consider is Z. Since the elements in Z have an ordering, and Zn has a geometric structure of a lattice, it seems that an EPI for Z should readily follows by using some of the existing proofs for EPI’s over R. However, as far as I know, there is no satisfactory extension of the EPI over Z so far. Szarek and Voiculescu [szarek] provide a geometric proof of Shannon’s EPI using the Brunn-Minkowski inequality in geometry — perhaps a similar approach could be utilized in the case of Z by employing results such as Gardner & Gronchi’s [gronchi] inequality for the integer lattice, or Ollivier & Villani’s [villani] inequality for the discrete hypercube. Another area that I am interested is related to the geometrization of probability program as proposed by Milman [milman]. Various concepts in geometry such as volume, surface area, and Minkowski sums have close analogs in information theory. A major challenge would to 5 develop an information-theoretic analog of the Brunn-Minkowski theory in geometry. We have started some work in this direction [jogananth2017], where we propose a notion of intrinsic entropies of random variables as an analog of intrinsic volumes of convex sets in geometry. We also conjecture an EPI for intrinsic entropies, along the lines of the Brunn- Minkowski inequality for intrinsic volumes, which is as yet open. In recent years, optimal transport theory has proved to be a useful tool to employ geometric ideas in analysis. It would be worth examining how optimal transport theory may be used in the geometrization of probability program as well. I would like to use the opportunity of this AIM workshop to discuss these ideas with other participants, and also learn about their open problems and conjectures. Bibliography [jogananth2014] Jog, Varun, and Venkat Anantharam.“The Entropy Power Inequality and Mrs. Gerber’s Lemma for Groups of Order 2n.” IEEE Transactions on Information Theory 60.7 (2014): 3773-3786. [szarek] Szarek, S. J., and D. Voiculescu.“Shannons entropy power inequality via restricted Minkowski sums.” Geometric aspects of functional analysis. Springer Berlin Heidelberg, 2000. 257-262. APA [gronchi] Gardner, R., and P. Gronchi.“A Brunn-Minkowski inequality for the integer lat- tice.” Transactions of the American Mathematical Society 353.10 (2001): 3995-4024. [villani] Ollivier, Yann, and Cdric Villani.“A Curved Brunn–Minkowski Inequality on the Discrete Hypercube, Or: What Is the Ricci Curvature of the Discrete Hypercube?” SIAM Journal on Discrete Mathematics 26.3 (2012): 983-996. [milman] Milman, Vitali D.“Geometrization of probability.” Geometry and dynamics of groups and spaces. Birkhuser Basel, 2007. 647-667. [jogananth2017] Jog, Varun, and Venkat Anantharam. “Intrinsic entropies of log-concave random variables.” ArXiv preprint, 2017. Available online at https://arxiv.org/abs/1702.01203. A.6 Johnson, Oliver Two particular EPI-related topics which are of interest to me are as follows: 1. Developing sharp results of EPI type for discrete random variables. I very much hope that this workshop will help the large community of people who have results in this area to work together, and see how these ideas can be extended and unified. For example, my own work (with Yaming Yu) on thinning and Poisson distributions https://doi.org/10.1109/TIT.2010.2070570 produces some (positive and negative) results, including a Artstein-Ball-Barthe-Naor type monotonicity result, but this (a) has a technical and non-intuitive proof (b) does not covert into a sharp EPI. One particular area of recent interest to me is the beamsplitter addition operation as discussed for example in my recent arxiv paper https://arxiv.org/abs/1701.07089 (with Saikat Guha).
Details
-
File Typepdf
-
Upload Time-
-
Content LanguagesEnglish
-
Upload UserAnonymous/Not logged-in
-
File Pages13 Page
-
File Size-