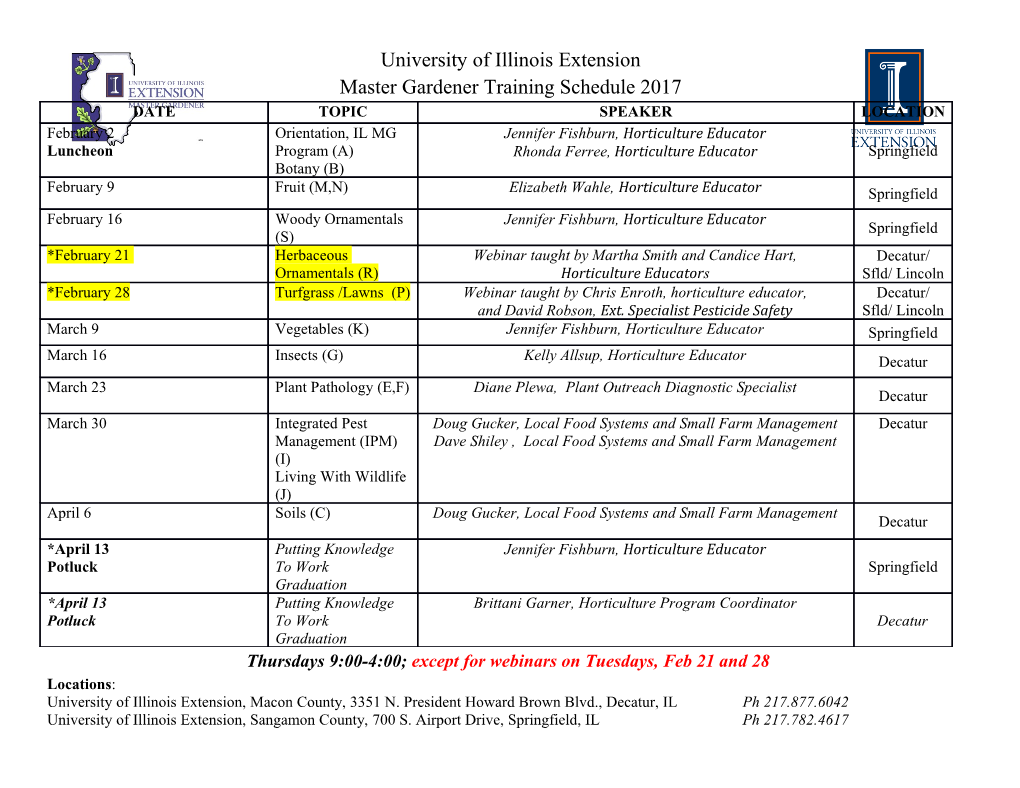
1 On the Relationship Between Environmental Parameters and Cloud Properties in 2 Stratocumulus Clouds Using Four Summers of Airborne Data Off the California Coast 3 4 1Department of Chemical and Environmental Engineering, University of Arizona, Tucson, AZ, 5 USA 6 2Department of Atmospheric Sciences, University of Arizona, Tucson, AZ, USA 7 NOAA 8 3Naval Postgraduate School, Monterey, CA, USA 9 4Department of Chemical Engineering, California Institute of Technology, Pasadena, CA, USA 10 *Corresponding author (phone: 520-626-5858, email: [email protected], address: PO 11 BOX 210011, Tucson, AZ 85721) 1 12 Abstract 13 …. 2 14 1. Introduction 15 Stratocumulus clouds have been studied extensively over numerous decades in part due 16 to both the challenge of explaining their nature and their importance to the planet’s energy 17 balance and other environmental issues (e.g., Wood et al., 2012). These clouds have a significant 18 impact on radiative forcing, physicochemical properties of aerosol particles, and biogeochemical 19 cycling of nutrients. Factors governing the microphysical and macrophysical properties of warm 20 clouds are a major source of uncertainty in climate models (e.g. Levin and Cotton, 2008; Stevens 21 and Feingold, 2009; Tao et al., 2012; Wood 2012; Boucher et al., 2013; Wang et al., 2013) 22 owing to a high degree of dynamical variability and the existence of multiple feedbacks. 23 The largest source of uncertainty in climate change prediction is rooted in the effective 24 radiative forcing linked to aerosol-cloud interactions (IPCC, 2013). As a result, aggressive 25 research has focused on examining relationships between aerosol concentrations and cloud 26 properties such as the incidence and magnitude of precipitation (L’Ecuyer et al., 2009; Wang et 27 al., 2012), cloud microphysical parameters such as droplet effective radius, cloud drop number 28 concentration, and cloud optical depth (e.g., Feingold et al., 2003; McComiskey et al., 2009), 29 cloud fraction and macrophysical properties such as liquid water path (Albrecht et al., 1989; 30 Nakajima et al., 2001), albedo (Twomey et al., 1977a; Platnick and Twomey, 1994), and process 31 timescales such as converting cloud water to rain water (e.g., Sorooshian et al., 2013). Widely 32 accepted principles include that, at fixed LWP, cloud albedo increases with more aerosol due to 33 smaller but more numerous drops (Twomey et al., 1977a), and that collision-coalescence 34 between smaller drops is suppressed for more polluted clouds leading to suppressed precipitation 35 and changes in LWP (Albrecht et al., 1989). 36 A strategy employed to investigate the influence of aerosol on the aforementioned cloud 37 characteristics has been to examine correlations, quantification of physically-relevant metrics 38 (e.g., susceptibilities), and regression techniques to test and improve parameterizations employed 39 in models. A critical issue in these investigations has been the aerosol proxy used as there are 40 limitations associated with each type of measurement platform; for example, remote sensing 41 retrievals struggle to provide information strictly near cloud base while airborne measurements 42 struggle to provide broad spatiotemporal coverage and statistics. Furthermore, past studies have 43 mostly been focused on using only a proxy for aerosol concentration and did not probe deeper to 44 examine characteristics of the aerosol beyond just number concentration using in-situ 45 measurements. Characteristics that may have an impact, depending on environmental conditions, 46 include aerosol size distribution and chemical composition. 47 This study aims to use a multi-campaign dataset to examine interrelationships between 48 numerous cloud properties and aearosol physicochemical properties. A unique aspect of this 49 work is the extensive statistics collected over the course of four field campaigns in the exact 50 same region (coastal California coast) with nearly the same payload in the same months of the 51 year (July-August) when stratocumulus cloud cover is at a maximum. The structure of this paper 52 is as follows: (i) overview of campaigns and instrument datasets; examination of factors 53 governing (ii) cloud drop concentration, (iii) drop effective radiust, (iv) drizzle rate, and (v) 54 relationship between cloud thickness and LWP; and (vi) summary of results. 55 56 2. Experimental Methods 57 Airborne data are used from four field experiments based out of Marina, California using 58 the Center for Interdisciplinary Remotely-Piloted Aircraft Studies (CIRPAS) Twin Otter. The 59 first Marine Stratus/Stratocumulus Experiment (MASE-I; Lu et al., 2007) included 13 flights in 3 60 July 2005, the second Marine Stratus/Stratocumulus Experiment (MASE-II; Lu et al., 2009) 61 included 16 research flights in July 2007, the Eastern Pacific Emitted Aerosol Cloud Experiment 62 (E-PEACE; Russell et al., 2013) included 30 flights between July and August in 2011, and the 63 Nucleation in California Experiment (NiCE) included 23 flights between July and August in 64 2013 (Coggon et al., 2014). 65 During these experiments, the Twin Otter conducted ~4-4.5 h flights at an airspeed of 66 ~50 m s-1 in the area encompassed by 34º N – 40º N and 121.5º W – 125º W with nearly the 67 same payload including the following: (i) particle number concentrations in the different size 68 ranges were observed with a condensation particle counter (CPC 3010; TSI Inc.; Dp > 10 nm), a 69 passive cavity aerosol spectrometer probe (PCASP; Dp ~ 0.1 – 2.6 μm), and a scanning mobility 70 particle sizer (SMPS; Dp ~ 15 nm — 800 nm) comprising a differential mobility analyzer (DMA 71 Model 3081, TSI Inc.) coupled to a CPC (Model 3010, TSI Inc.); (ii) droplet size distributions 72 between 0.4 μm and 1.6 mm were obtained with a cloud aerosol spectrometer (CAS), forward 73 scattering spectrometer probe (FSSP), and cloud imaging probe (CIP); (iii) sub-micrometer mass 74 concentrations of non-refractory aerosol constituents were obtained with an Aerodyne Aerosol 75 Mass Spectrometer (AMS; Drewnick et al., 2005); (iv) meteorological data (e.g., temperature, 76 humidity, winds, Gerber probe liquid water content (LWC; Gerber et al., 1994)). As presented in 77 numerous past studies (e.g., Prabhakar et al., 2014; Crosbie et al., 2016), the general flight 78 pattern used in the marine atmosphere is as follows: level legs below cloud base, immediate 79 above cloud base, at mid-cloud altitude, immediately below cloud top, immediately above cloud 80 top (called “wheels-in” leg), and a few hundred feet higher as part of a “free troposphere” leg. In 81 addition to the leg profiling, vertical soundings, either as a slant or spiral, were conducted before 82 and after each of these sets of leg patterns, from which column-integrated could be calculated. 83 Details of calculations of cloud parameters such as LWP, drop effective radius (re), cloud optical 84 depth (τ) and rain rate are summarized in past studies (e.g., Chen et al., 2012). Table 1 reports a 85 summary of nomenclature used to represent the aforementioned (and other) measurement 86 parameters. 87 Numerous calculations were made that required choices. Clear air is identified as having 88 liquid water content (LWC) values below 0.01 g m-3, and thus values exceeding this value were 89 used to identify cloud base and top heights. Inversion strength is calculated here as the difference 90 of potential temperature between the inversion-top and -base heights; inversion-base height is 91 defined as the altitude where temperature first reaches a minimum above the surface and the top 92 is defined as the height that the 5-s running mean of dθ/dz reaches a maximum. 93 94 3. Results and Discussion 95 In the subsequent discussion we examine interrelationships between microphysical and 96 macrophysical parameters relevant to aerosol particles and clouds, includng the following: (i) 97 cloud drop number concentration (Nd), (ii) cloud drop effective radius (re), (iii) drizzle rate (R), 98 and (iv) LWP. The number of data points used in the following analyses ranges between 135- 99 258, depending on the variables being used as some parameters may have been unavailable or 100 below detection limits. 101 102 3.1 Cloud Drop Number Concentration (Nd) 103 Two common ways field data are often used to examine the relationship between sub- 104 cloud aerosol concentraiton (Na) and cloud drop number concentration is with the ACI metric, 105 , and by power law regression fits, ∝ . ACIN is related to α, with values 4 106 ranging from 0 to 1. A characteristic α value of ~0.7 has been proposed based on heuristics 107 (Twomey, 1977b). Reported α values from MASE I and MASE II were 0.563 and 0.594, 108 respectively (Lu et al., 2009). Factors that have been documented that increase the value of α 109 include increases in updraft velocity and adiabaticity, while reduced values are observed when 110 there is more active collision-coalescence and high Angstrom exponents (i.e., smaller aerosol) 111 (e.g., McComiskey et al., 2009). Leaitch et al. (1996) showed that Nd variance could be 112 explained better by Na and cloud water sulfate concentrations when data were separated into 113 categories of turbulence as quantified by the standard deviation of updraft velocity w΄ (σw). Lu et 114 al. (2007) also confirmed that inclusion of updraft velocity variabiity to Na values improved 115 predictions of Nd. Here we examine the ranking of the most important parameters in our dataset 116 to predict values of Nd. 117 Regression analysis was conducted to identify (i) the best single parameter to predict Nd, 118 (ii) the best two-parameter models, and (iii) the highest level of improvement in a two-parameter 119 model as compared to the individual single-parameter models (Table 2). Model rank is based on 120 values of the coefficient of determination (r2), which quantifies how much of the variabile in a 121 response variable is explained by the predictive variable(s).
Details
-
File Typepdf
-
Upload Time-
-
Content LanguagesEnglish
-
Upload UserAnonymous/Not logged-in
-
File Pages21 Page
-
File Size-