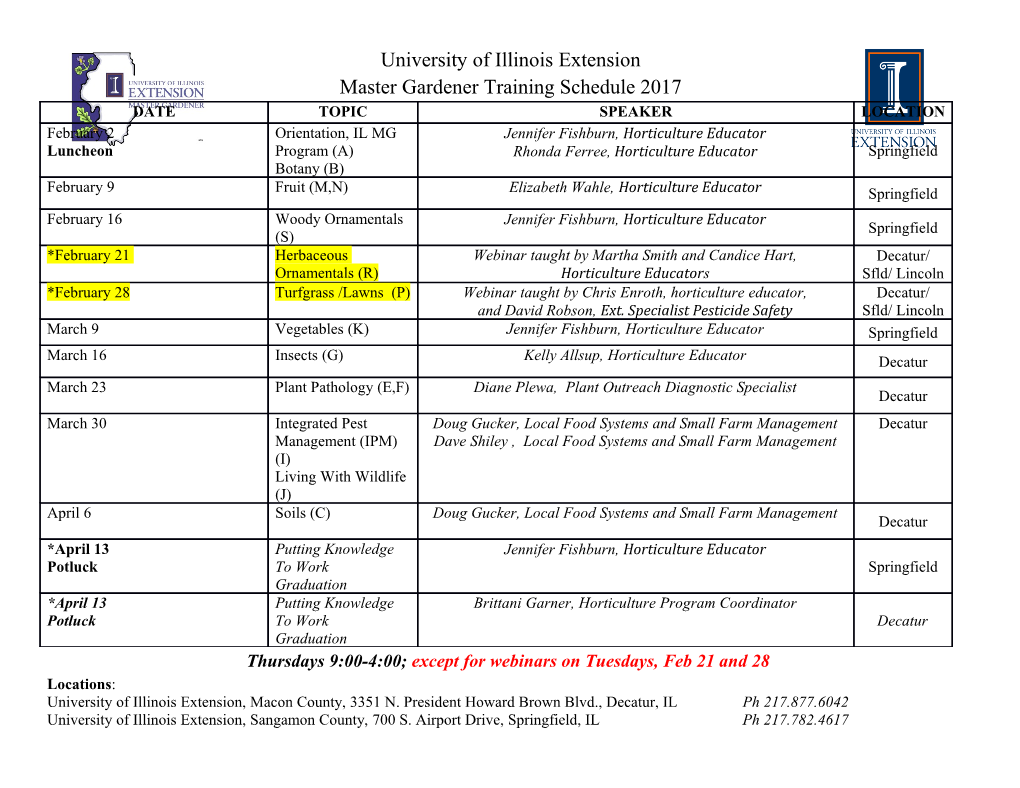
Time Series: Economic Forecasting Brockwell P J, Davis R A 1991 Time Series: Theory and Panel Data; Simultaneous Equation Estimation: Oer- Methods, 2nd edn. Springer, New York iew). Time-series models typically forecast the vari- Crame! r H 1942 On harmonic analysis of certain function spaces. W able(s) of interest by implicitly extrapolating past Arki fur Matematik, Astronomi och Fysik. 28B(12): 1–7 policies into the future, while structural models, Dagum E B 1980 The X-11 ARIMA Seasonal Adjustment Method. Research Paper, Statistics, Canada because they rely on economic theory, can evaluate Geweke J F 1978 The revision of seasonally adjusted time series. hypothetical policy changes. In this light, perhaps it Proceedings of the Business and Economic Statistics Section— is not surprising that time-series models typically American Statistical Association, pp. 320–25 produce forecasts as good as, or better than, far more Granger C W J, Newbold P 1977 Forecasting Economic Time complicated structural models. Still, it was an intel- Series. Academic Press, New York lectual watershed when several studies in the 1970s Hamilton J 1994 Time Series Analysis. Princeton University (reviewed in Granger and Newbold 1986) showed that Press, Princeton, NJ simple univariate time-series models could outforecast Harvey A 1993 Time Series Models, 2nd edn. MIT Press, the large structural models of the day, a result which Cambridge, UK Kolmorgorov A N 1940 Kurven in Hilbertschen Raum die continues to be true (see McNees 1990). This good gegenu$ ber eine einparametrigen Gruppe von Bewegungen forecasting performance, plus the relatively low cost of invariant sind. C. R. (Doklady) de L’Academie des Sciences de developing and maintaining time-series forecasting l’IRSS, New Series 26: 6–9 models, makes time-series modeling an attractive way Nerlove M, Grether D M, Carvalho J L 1979 Analysis of to produce baseline economic forecasts. Economic Time Series. Academic Press, New York At a general level, time-series forecasting models Priestly M B 1981 Spectral Analysis and Time Series. Academic can be written, Press, New York Wallis K F 1974 Seasonal adjustment and relations between y l g(X , θ)jε (1) variables. Journal of the American Statistical Association 69: t+h t t+h 18–31 where yt denotes the variable or variables to be M. W. Watson forecast, t denotes the date at which the forecast is made, h is the forecast horizon, Xt denotes the variables used at date t to make the forecast, θ is a ε vector of parameters of the function g, and t+h denotes the forecast error. The variables in Xt usually in- clude current and lagged values of yt. It is useful to Time Series: Economic Forecasting define the forecast error in (1) such that it has ε Q l conditional mean zero, that is, E( t+h Xt) 0. Thus, Time-series forecasts are used in a wide range of given the predictor variables Xt, under mean-squared economic activities, including setting monetary and error loss the optimal forecast of y is its conditional θ t+h fiscal policies, state and local budgeting, financial mean, g(Xt, ). Of course, this forecast is infeasible management, and financial engineering. Key elements because in practice neither g nor θ are known. The task of economic forecasting include selecting the fore- of the time-series forecaster therefore is to select the θ casting model(s) appropriate for the problem at hand, predictors Xt, to approximate g, and to estimate in assessing and communicating the uncertainty asso- such a way that the resulting forecasts are reliable and ciated with a forecast, and guarding against model have mean-squared forecast errors as close as possible instability. to that of the optimal infeasible forecast. Time-series models are usefully separated into univariate and multivariate models. In univariate 1. Time Series Models for Economic Forecasting models, Xt consists solely of current and past values of yt. In multivariate models, this is augmented by data Broadly speaking, statistical approaches to economic on other time series observed at date t. The next forecasting fall into two categories: time-series subsections provide a brief survey of some leading methods and structural economic models. Time-series time-series models used in economic forecasting. For methods use economic theory mainly as a guide to simplicity attention is restricted to one-step ahead variable selection, and rely on past patterns in the data forecasts (h l 1). Here, the focus is on forecasting in a to predict the future. In contrast, structural economic stationary environment; the issue of nonstationarity in models take as a starting point formal economic theory the form of structural breaks or time varying para- and attempt to translate this theory into empirical meters is returned to below. relations, with parameter values either suggested by theory or estimated using historical data. In practice, time-series models tend to be small with at most a 1.1 Uniariate Models handful of variables, while structural models tend to be large, simultaneous equation systems which some- Univariate models can be either linear, so that g is times incorporate hundreds of variables (see Economic linear in Xt, or nonlinear. All linear time-series models 15721 Time Series: Economic Forecasting can be interpreted as devices for modeling the co- data that switches between the ‘regimes’ α(L) and o q l β variance structure of the data. If yt , t 1, … ,T has a (L). Variations of Eqn. (2) interact dt with only some Gaussian distribution and if this distribution is sta- autoregressive coefficients, the intercept, and\or the tionary (does not depend on time), then the optimal error variance. Various functions are available for dt, forecast is a linear combination of past values of the either a sharp indicator function (the threshold auto- data with constant weights. Different linear time-series regressive model) or a smooth function (smooth l models provide different parametric approximations transition autoregression). For example, setting dt j γ jγh ζ −" to this optimal linear combination. (1 exp [ ! " t]) , yields the logistic smooth tran- The leading linear models are autoregressive sition autoregression (LSTAR) model, where ζ de- ζ t models, autoregressive–integrated moving-average notes current or past data, say, t might equal yt−k for (ARIMA) models, and unobserved components some k. See Granger and Tera$ svirta (1993) for models. additional details. For an application of threshold An autoregressive model of order p (AR(p)) is autoregressions (and other models) to forecasting US written, unemployment, see Montgomery et al. (1998). The Markov switching models are conceptually lµjα j ( jα jε lµjα jε yt+" "yt pyt−p+" t+" (L)yt t+", similar, except that the regime switch depends on an unobserved series. In the context of Eqn. (2), dt is where (µ, α ,…,α ) are unknown parameters, L is the modeled as unobserved and following a two-state " pα lag operator, and (L) is a lag polynomial. If yt is Markov process; see Hamilton (1994). observed at all dates, t l 1, … , T, these parameters An alternative approach is to treat g itself as are readily estimated by ordinary least squares. unknown, which leads to nonparametric methods. ARIMA models extend autoregressive models to These include nearest neighbor, kernel, and artificial include a moving-average term in the error, which has neural network models for the conditional expecta- the effect of inducing long lags in the forecast when it tion. In practice these nonparametric approaches to is written as a function of current and past values of economic forecasting have met with success which is yt. These are discussed in Time Series: ARIMA mixed at best. There are several possible reasons for Methods. this, including that there are insufficiently many In an unobserved components model, yt is repre- observations in typical economic data sets to support sented as the sum of two or more different stochastic the use of these data-intensive methods. components. These components are then modeled, and the combined model provides a parametric ap- proximation to the autocovariances; see Harvey (1989) 1.2 Multiariate Models for a detailed modern treatment. The unobserved components framework can provide a useful frame- In multivariate time-series models, Xt includes mul- work for extracting cyclical components of economic tiple time-series that can usefully contribute to fore- time-series and for seasonal adjustment (see Time casting yt+". The choice of these series is typically Series: Seasonal Adjustment). guided by both empirical experience and by economic o q If yt is not Gaussian, in general the optimal theory, for example, the theory of the term structure of forecast will not be linear, which suggests the use of interest rates suggests that the spread between long forecasts based on nonlinear time-series models. Para- and short term interest rates might be a useful metric nonlinear time-series models posit a functional predictor of future inflation. form g, and take this as known up to the finite- The multivariate extension of the univariate auto- dimensional parameter vector θ. Two leading ex- regression is the vector autoregression (VAR), in amples of parametric nonlinear time-series models, which a vector of time-series variables, Yt+", is repre- currently popular for economic forecasting, are thres- sented as a linear function of Yt,…,Yt−p+", perhaps hold autoregressive models and Markov switching with deterministic terms (an intercept or trends). An models. Both are similar in the sense that they posit interesting possibility arises in VARs that is not two (or more) regimes. In the threshold autoregres- present in univariate autoregressions, specifically, it sion, switches between the regimes occur based on past might be that the time-series are cointegrated (that is, values of the observed data; in Markov switching the individual series are nonstationary in the sense that models, the switches occur based on an unobserved or they are integrated, but linear combinations of the latent variable.
Details
-
File Typepdf
-
Upload Time-
-
Content LanguagesEnglish
-
Upload UserAnonymous/Not logged-in
-
File Pages4 Page
-
File Size-