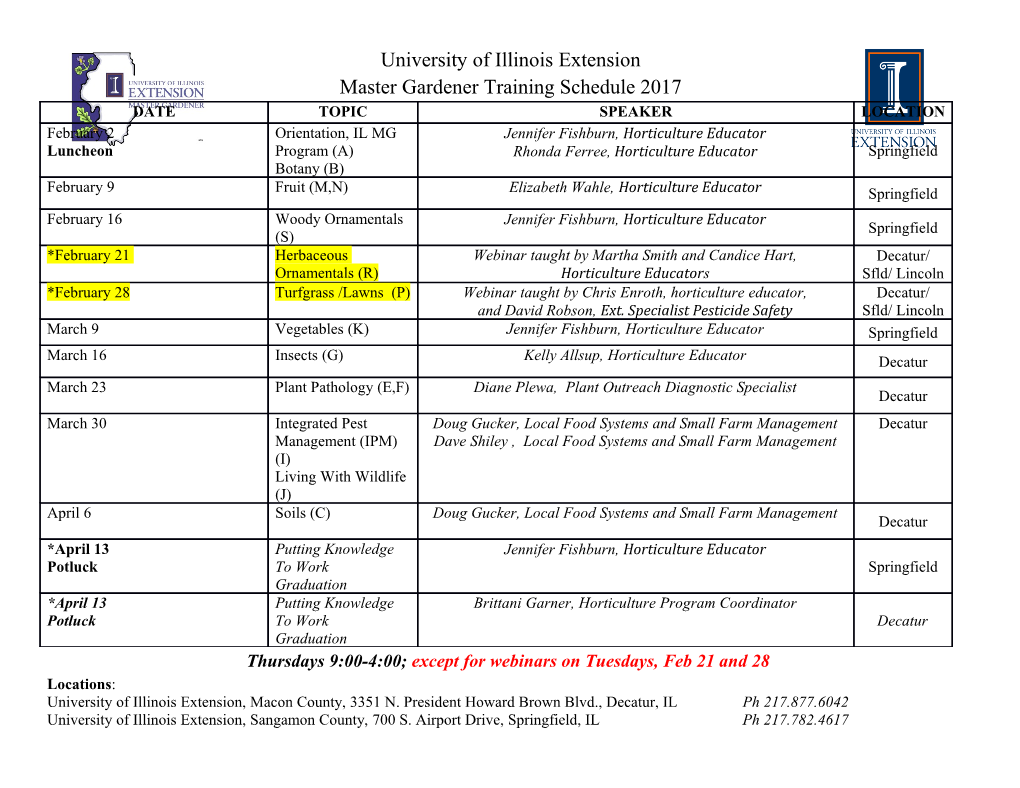
3D Modelling Using Geometric Constraints: A Parallelepiped Based Approach Marta Wilczkowiak, Edmond Boyer, and Peter Sturm MOVI–GRAVIR–INRIA Rhˆone-Alpes, 38330 Montbonnot, France, [email protected], http://www.inrialpes.fr/movi/people/Surname Abstract. In this paper, efficient and generic tools for calibration and 3D reconstruction are presented. These tools exploit geometric con- straints frequently present in man-made environments and allow cam- era calibration as well as scene structure to be estimated with a small amount of user interactions and little a priori knowledge. The proposed approach is based on primitives that naturally characterize rigidity con- straints: parallelepipeds. It has been shown previously that the intrinsic metric characteristics of a parallelepiped are dual to the intrinsic charac- teristics of a perspective camera. Here, we generalize this idea by taking into account additional redundancies between multiple images of multiple parallelepipeds. We propose a method for the estimation of camera and scene parameters that bears strongsimilarities with some self-calibration approaches. Takinginto account prior knowledgeon scene primitives or cameras, leads to simpler equations than for standard self-calibration, and is expected to improve results, as well as to allow structure and mo- tion recovery in situations that are otherwise under-constrained. These principles are illustrated by experimental calibration results and several reconstructions from uncalibrated images. 1 Introduction This paper is about using partial information on camera parameters and scene structure, to simplify and enhance structure from motion and (self-) calibration. We are especially interested in reconstructing man-made environments for which constraints on the scene structure are usually easy to provide. Constraints such as parallelism, orthogonality or length ratios, are often available, and we show that they are especially powerful if they can be encapsulated in higher-level primitives. Concretely, man-made environments are rich in elements that may be defined as parallelepipeds or parallelograms. Encoding constraints using these, reinforces them, simplifies the user interactions necessary to provide them, and allows an easier analysis of issues such as the existence of solutions. In the following, we briefly review some existing works that use constraints on scene structure. Geometric information about the scene can be used in many different ways. In a seminal work, Caprile and Torre [2] used cuboids, i.e. par- allelepipeds with right angles, to estimate some camera parameters. Their ap- proach is based on vanishing points defined by the cuboid’s projected edges. Such A. Heyden et al. (Eds.): ECCV 2002, LNCS 2353, pp. 221–236, 2002. c Springer-Verlag Berlin Heidelberg 2002 222 M. Wilczkowiak, E. Boyer, and P. Sturm vanishing points correspond to perpendicular directions in space and therefore impose constraints on the transformation between 3D space and the image plane. Following this idea, several approaches making use of vanishing points and lines, have been proposed to either partially calibrate cameras or reconstruct scenes [4,14,5,12]. However, dealing with individual vanishing points does not allow to fully exploit the redundancy contained in the input, i.e. that all the vanishing points stem from the projection of a single parallelepiped. In contrast to the above mentioned approaches, we do not compute vanishing points or lines ex- plicitly, but projection matrices such that a parallelepiped’s projection fits the concerned image points. In [7], different kinds of primitives, including cubes, are used for 3D reconstruction. However, this approach, cast as a bundle adjustment, has only a very simple initialization step. In [3], parallelepipeds are used for cal- ibration in AR applications. The proposed approach has a limited application domain since the camera must satisfy a strong constraint – unit aspect ratio – and only partial knowledge on the parallelepiped – angles – is used. In addition to using scene constraints, our approach also takes into account any constraint on intrinsic parameters of any camera involved. There is a perfect duality between the intrinsic parameters of a perspective camera and those of a parallelepiped [20]. We formalize this in a generic framework, in which cam- eras and parallelepipeds are treated in a dual manner. One of the main issues of this paper is the use of multi-view and multi-parallelepiped constraints, as op- posed to using knowledge on single images or parallelepipeds only. Multi-view or multi-parallelepiped constraints arise when it is known that several entities share properties (e.g. two views with identical aspect ratio, or two parallelepipeds with identical shape). These constraints are incorporated in our dual framework. For the various types of constraints, it is shown which types of equations can be ob- tained. In many practical circumstances, (self-) calibration of intrinsic cameras and parallelepiped parameters can be done by solving linear equation systems. Our approach has some similarities with methods based on planar patterns and homographies [18,21]. While more flexible than standard calibration tech- niques, homography based approaches require either Euclidean information or, for self-calibration, many images in general position [19]. The approach presented in this paper works for a small number of images (a single image for example) and can make use of any metric information on calibration primitives independently, for example one angle between directions, or one length ratio, give additional constraints. In this sense, our approach is a generalization of plane-based meth- ods with metric information to three-dimensional parallelepipedic patterns. While the main contributions of the paper concern the estimation of intrinsic camera and parallelepiped parameters, we also propose methods for subsequent pose estimation, as well as ways of enhancing reconstructions with primitives other than parallelepipeds. The complete system allows calibration and 3D model acquisition from a small number of arbitrary images, taken for instance from the Internet, with a reasonable amount of user interaction. 3D ModellingUsingGeometric Constraints 223 The paper is organized as follows. Section 2 gives definitions and some back- ground. Calibration using parallelepipeds is studied in section 3. Sections 4 and 5 describe our approaches for pose estimation and 3D reconstruction. 2 Preliminaries 2.1 Camera Parameterization We assume that all cameras can be described by the pinhole model. The pro- jection from a 3D point P to the 2D image point p is expressed by: p ∼ M · P , where M is a 3x4 matrix, which can be decomposed as: M = K · [Rt]. The 3 × 4 matrix [Rt] encapsulates the relative orientation R and translation t be- tween the camera and the world coordinate system. The matrix K is the 3 × 3 calibration matrix containing the camera’s intrinsic parameters: αu su0 K = 0 αv v0 , 001 where αu and αv stand for the focal length, expressed in horizontal and vertical pixel dimensions, s is a skew parameter considered here as equal to zero, and u0, v0 are the pixel coordinates of the principal point. In the following, we will also use the IAC (image of the absolute conic) representation of the intrinsic parameters, namely the matrix ω ∼ K−T · K−1. 2.2 Parallelepiped Parameterization A parallelepiped is defined by twelve parameters: six extrinsic parameters de- scribing its orientation and position, and six intrinsic parameters describing its Euclidean shape: three dimension parameters (edge lengths l1,l2 and l3) and the three angles between parallelepiped edges (θ12,θ23,θ13). The internal character- istics of a parallelepiped can be expressed by a matrix Λ˜ which represents the transformation between a canonic cube and the shape of the concerned paral- lelepiped (see [20] for details): l1 l2c12 l3c13 0 c23−c13c12 0 l2s12 l3 0 ˜ s12 Λ = 2 2 2 2 , s12−c13s12−(c23−c13c12) 00l3 2 0 s12 00 0 1 where sij = sin θij and cij = cos θij. This matrix encodes all Euclidean charac- teristics of the parallelepiped. The analogous entity to a camera’s IAC ω,isthe matrix µ, defined by: 2 l1 l1l2 cos θ12 l1l3 cos θ13 T 2 µ ∼ Λ · Λ ∼ l1l2 cos θ12 l2 l2l3 cos θ23 , 2 l1l3 cos θ13 l2l3 cos θ23 l3 where Λ is the upper left 3 × 3 matrix of Λ˜. 224 M. Wilczkowiak, E. Boyer, and P. Sturm 2.3 One Parallelepiped in a Single View In this section, we briefly recall the duality between the intrinsic characteristics of a camera and those of a parallelepiped, as established in [20]. Without loss of generality, suppose that the scene coordinate system is attached to a paral- lelepiped. The projection of the parallelepiped is then defined by the relative pose [Rt] between the parallelepiped and the camera, the camera calibration matrix K and the parallelepiped’s intrinsic parameters, given by Λ˜. In the following, we denote by C the matrix whose columns Ci,i∈[1..8] are the homogeneous coordi- nates of a canonic cube’s vertices. Thus, image projections pi∈[1..8] =[ui vi 1] of the parallelepiped’s vertices satisfy: 1 ... −1 α u ... α u 1 1 8 8 1 ... −1 α v ... α v = X˜ · C = X˜ · , (1) 1 1 8 8 1 ... −1 α ... α 1 8 1 ... 1 where the 3 × 4 matrix X˜, is defined up to a scalar factor by: X˜ ∼ M · Λ˜ ∼ K · [Rt] · Λ.˜ (2) We call this matrix the canonic projection matrix. Five image points and one image direction are, in general, sufficient to compute its eleven independent entries. Additional points make the computation more stable. For further derivations, let us also introduce the leading 3 × 3 sub-matrices X and Λ of X˜, Λ˜ respectively, such that: X ∼ K · R · Λ. (3) The matrix X captures all geometric information given by the projection of a parallelepiped. From equation (3), it is simple to derive the following relation (using ω = K−T · K−1 and µ = ΛT · Λ): XT · ω · X ∼ µ. (4) This equation establishes the duality between the intrinsic characteristics of a camera and those of a parallelepiped.
Details
-
File Typepdf
-
Upload Time-
-
Content LanguagesEnglish
-
Upload UserAnonymous/Not logged-in
-
File Pages16 Page
-
File Size-