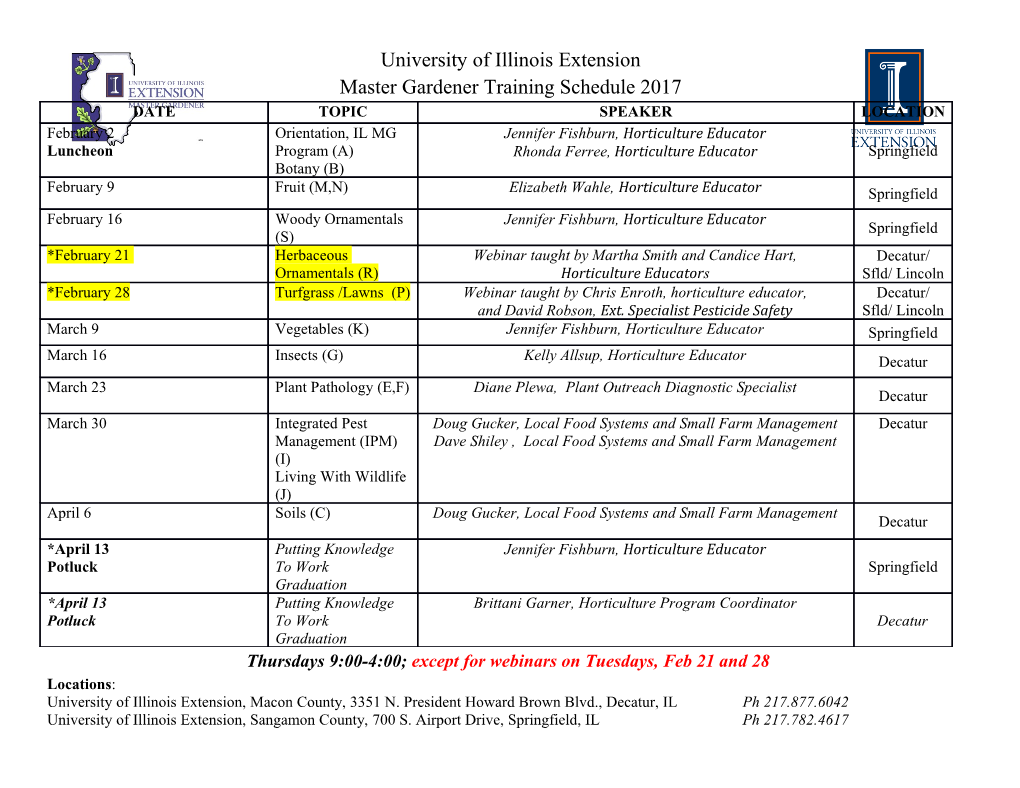
SUPPLEMENTARY MATERIALS An Anatomic Gene Expression Atlas of the Mouse Brain Lydia Ng¹, Amy Bernard¹, Chris Lau¹, Caroline C. Overly¹, Hong‐Wei Dong², Leonard Kuan¹, Sayan Pathak¹, Susan M. Sunkin¹, Chinh Dang¹, Jason W. Bohland3, Hemant Bokil3, Partha P. Mitra3, Luis Puelles4, John Hohmann¹, David J. Anderson5, Ed S. Lein¹, Allan R. Jones¹, & Michael Hawrylycz¹,6 ¹Allen Institute for Brain Science, Seattle, WA USA 98103 ²Laboratory for NeuroImaging, UCLA, Los Angeles, CA 90095 3Cold Spring Harbor Laboratory, Cold Spring Harbor, NY, 11724 4Department of Human Anatomy and Psychobiology, and CIBER en Enfermedades Raras, U736, Faculty of Medicine, University of Murcia, Spain 5Division of Biology, California Institute of Technology, Pasadena CA 91125 6Corresponding author, [email protected] A. Supplementary Methods ISH processing and imaging. ISH tissue sections (25μm thick) were generated from 56 day old male C57BL/6J mouse brains. Each section was labeled for a specific gene using a semi‐automated ISH protocol1. The Image Capture System consists of 10 independent Leica DM6000B microscopes with a Leica DC500 camera, and mounted on an air table to isolate the microscope from external vibration sources. The image capture procedure is essentially fully automated and collects each tissue section at 10x magnification and 1.07 µm/pixel resolution storing directly into the JPEG2000 format. Details can be found in1. 3D reference atlas. The process of 3D mapping starts with atlas‐based annotation using a novel high‐ resolution anatomic atlas, the Allen Reference Atlas (ARA). The ARA volume was derived from 528 coronal Nissl stained sections (25μm thick) from an unfixed, frozen mouse brain. From this set, 132 sections, with 100μm spacing, were annotated for over 1000 brain structures to form the ARA (Supplementary Fig. 1). The 3D Nissl volume is a result of a rigid reconstruction process where each section is reoriented to match the adjacent images as closely as possible, forming an assembled 3D volume. A 1.5T low resolution 3D average MRI volume was used to ensure that the reconstruction resulted in a realistic volume2. Each reoriented Nissl image was then downsampled and converted to grayscale to form an isotropic 25μm grayscale volume. Further boundaries for 208 large structures and structural groupings were extracted from the ARA and projected and smoothed onto the 3D atlas volume to provide the accompanying structural annotation in the AGEA interface (Fig. 1b). An additional decomposition of the cortex into an intersection of 202 regions and areas was performed. The 3D reference atlas is divided into a 200µm3 grid consisting of 67 coronal x 41 horizontal x 58 sagittal cells or voxels (sum=159,326) of which 51,533 voxels are masked to intersect the ABA data. These 200µm voxels form the smallest spatial unit for analysis. Nature Neuroscience: doi:10.1038/nn.2281 Supplementary Figure 1. Neuroanatomy of the Allen Reference Atlas. (a) Web‐view versions (screen‐ shots) of the Allen Brain Atlas that are accessible through the AGEA interface, with the neuroanatomical ontology used in the Allen Reference Atlas. A direct link is available from each structure in AGEA to detailed anatomy and parent/child relationships. (b) A representative full reference atlas plate, which can be viewed in high resolution, is shown. Expression energy statistics. An adaptive threshold algorithm3 identifies expressing cells in each 2D tissue section, resulting in an expression mask that effectively classifies each pixel in the image as to whether that pixel represents expressing cells (Fig. 1d). A single statistic called expression energy is a convenient representation of the information content in each voxel and represents the product of expression area and expression intensity in that voxel1, 3. Expression energy E(C) for a cubic voxel C is defined as follows: ∑ M ()pIp× () EC()= ∀∈pC ||C where p is a image pixel that intersects voxel C, |C| is the total number of pixels that intersect C, M(p) is the expression segmentation mask which is either 1 (“expressing” pixel) or 0 (“non expressing” pixel) and I(p) is the grayscale value of the ISH image intensity defined as Gray = 0.3*Red + 0.59*Green + 0.11*Blue. The advantages of defining expression energy in this way is that it can be robustly computed over all brain regions; it is easily normalized to account for different image resolution and/or section sampling frequency; it is amenable to smoothing for noise suppression; and it combines features of expression intensity and expression density into a single measurement. Transcriptome profile. For each voxel, a transcriptome profile, over a predefined set of genes, can be constructed as a vector of expression energy values where each component of the vector represents a gene in the dataset. AGEA transcriptome profiles were constructed from 4,376 coronal image series in the ABA (See Methods: AGEA dataset). Nature Neuroscience: doi:10.1038/nn.2281 AGEA Application. The AGEA application is multi‐user, has no plug‐ins and uses AJAX Java server and C++ in a servlet application with Java script on the client. It is fully linked with the ARA and ontology and does slicing and threshold computation of the 3D correlation volumes at user access time. Detailed description of the user interface can be found on the accompanying online tutorial (http://mouse.brain‐ map.org/pdf/AGEA.pdf). AGEA Correlation maps. Pearson correlation4 was used to measure the similarity between two transcriptome profiles or vectors for each pair of distinct voxels. Correlation essentially measures linear relationship; two voxels have high positive correlation when high (or low) expression energy in one voxel is associated with high (or low) expression energy in the other voxel. A higher relative correlation value indicates greater fidelity to the linear relationship model. To make navigation and display of this large set (>109) pair‐wise correlation values intuitive, we have used a reference or “seed” voxel paradigm. To use the Correlation map tool, a user selects the “seed voxel” by moving the red cross‐hairs on the 3D Nissl atlas (Fig. 2a, upper panels), the corresponding 3D correlation map is then displayed (Fig. 2b, lower panels) as a 24‐bit false color images. The color at each voxel in the correlation map represents the correlation between this voxel and the “seed voxel”. A scale bar enables users to change the dynamic range of the color map, in which hot and cool colors represent higher and lower correlation respectively. The associated numerical data can be downloaded via links below the correlation maps. Supplementary Figure 2 illustrates the multiple scales on which gene expression can be analyzed. In the first panel, the mass of orange/red voxels indicates the cerebral cortex as a co‐ expressing unit. A subtle change from dark orange and light orange reflects the demarcation between the isocortex (or neocortex) and the olfactory areas. As we decrease the mapping range to [0.85,1] (Supplementary Fig. 2b), the existence of a coherent laminar layer 5 becomes more apparent. By decreasing the range even further to [0.92,1] in (Supplementary Fig. 2c), a more local region in the somatosensory cortex emerges. a b c Supplementary Figure 2. Changing correlation dynamic range. The effect of changing the threshold color scale bar for a voxel seed in Layer 5 of the cortex. Contracting toward a more restricted dynamic range focuses the spatial relationship first on the cortex–wide scale and then subsequently on a more regional intra‐layer 5 scale. Thresholds are [0.5,1], [0.85,1], and [0.92,1] for a, b , and c respectively. AGEA Clusters. This tool represents a purely transcriptome derived hierarchical spatial organization of the adult mouse brain. The 51,533 AGEA correlation maps were used to create hierarchical grouping or clustering of voxels with similar composite gene expression profiles across the AGEA dataset. The Nature Neuroscience: doi:10.1038/nn.2281 spectrum of gene expression patterns in the brain is complex displaying both intra‐structure widespread expression and multifarious regional specificity. We have used a simple strategy that captures the various scales of spatial co‐expression as described below. To construct the hierarchical clustering, voxels are spatially organized as a binary tree5 where each node represents a collection of voxels and has zero or two branches (children). To initialize, all 51,533 voxels were assigned to the root node of the tree. As we descend the tree, a node is bifurcated into two nodes to achieve maximal dissimilarity between two groups of voxels based on correlation values. The bifurcation terminates whenever a node contains only a single voxel. This recursive division scheme is effectively multi‐scale processing. The final tree consists of 103,065 nodes with a maximum depth of 53 levels and 51,533 leaf nodes (one for each voxel in the brain). At each bifurcation an ordering (albeit arbitrary) is assigned to each child to enable the definition a global “depth first” ordering for all leaf nodes. Effective visualization of this large tree data structure is a challenging problem. A viewer has been implemented to provide an easy‐to‐use mechanism to navigate the tree, provide 3D context and visualize the multi‐scale partitioning. Navigation works on the principle that from each leaf note there is only one path back to the root. To use the Cluster tool, a user selects a leaf node of the tree by choosing a voxel within the 3D reference volume and moves up and down the tree (along the root to leaf path) using the Tree Depth slider control below the cluster map. At each level of the tree, the voxels corresponding to the node can be browsed within its 3D spatial context. The voxels of a node are visualized with a systematic color coding scheme.
Details
-
File Typepdf
-
Upload Time-
-
Content LanguagesEnglish
-
Upload UserAnonymous/Not logged-in
-
File Pages131 Page
-
File Size-