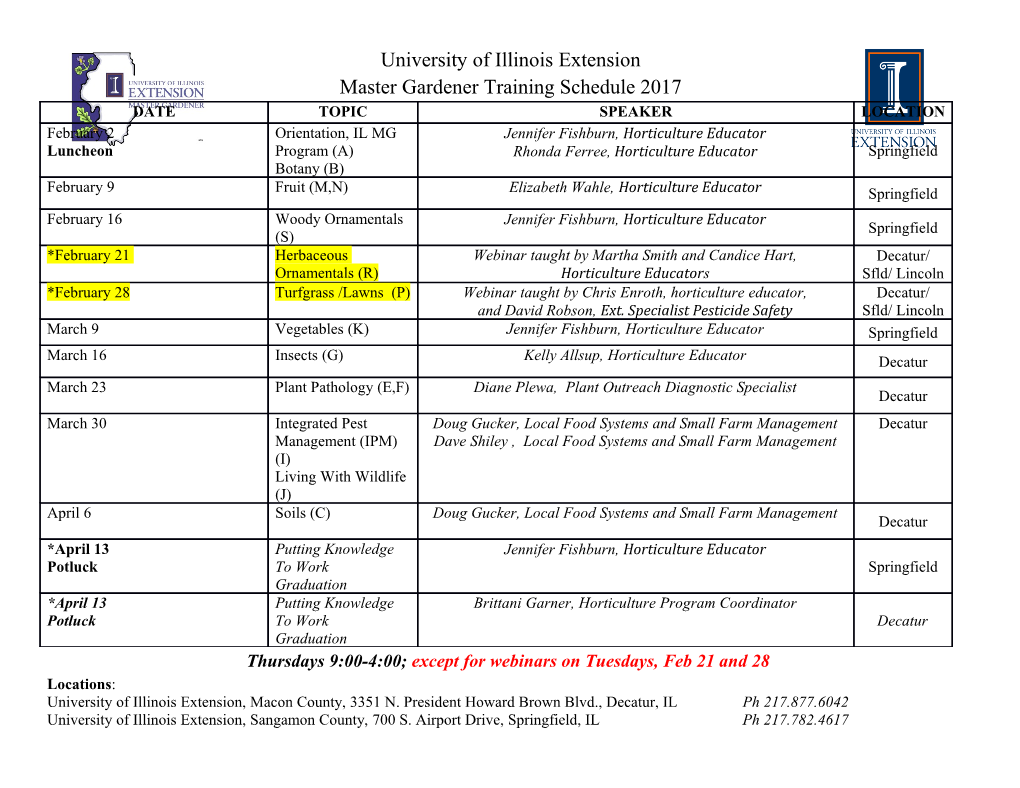
Nonparametric Location Tests: One-Sample Nathaniel E. Helwig Assistant Professor of Psychology and Statistics University of Minnesota (Twin Cities) Updated 04-Jan-2017 Nathaniel E. Helwig (U of Minnesota) Nonparametric Location Tests: One-Sample Updated 04-Jan-2017 : Slide 1 Copyright Copyright c 2017 by Nathaniel E. Helwig Nathaniel E. Helwig (U of Minnesota) Nonparametric Location Tests: One-Sample Updated 04-Jan-2017 : Slide 2 Outline of Notes 1) Background Information: 3) Sign Test (Fisher): Hypothesis testing Overview Location tests Hypothesis testing Order and rank statistics Estimating location One-sample problem Confidence intervals 2) Signed Rank Test (Wilcoxon): 4) Some Considerations: Overview Choosing a location test Hypothesis testing Univariate symmetry Estimating location Bivariate symmetry Confidence intervals Nathaniel E. Helwig (U of Minnesota) Nonparametric Location Tests: One-Sample Updated 04-Jan-2017 : Slide 3 Background Information Background Information Nathaniel E. Helwig (U of Minnesota) Nonparametric Location Tests: One-Sample Updated 04-Jan-2017 : Slide 4 Background Information Hypothesis Testing Neyman-Pearson Hypothesis Testing Procedure Hypothesis testing procedure (by Jerzy Neyman & Egon Pearson1): (a) Start with a null and alternative hypothesis (H0 and H1) about θ (b) Calculate some test statistic T from the observed data (c) Calculate p-value; i.e., probability of observing a test statistic as or more extreme than T under the assumption H0 is true (d) Reject H0 if the p-value is below some user-determined threshold Typically we assume observed data are from some known probability distribution (e.g., Normal, t, Poisson, binomial, etc.). 1Egon Pearson was the son of Karl Pearson (very influential statistician). Nathaniel E. Helwig (U of Minnesota) Nonparametric Location Tests: One-Sample Updated 04-Jan-2017 : Slide 5 Background Information Hypothesis Testing Confidence Intervals In addition to testing H0, we may want to know how confident we can be in our estimate of the unknown population parameter θ. A symmetric 100(1 − α)% confidence interval (CI) has the form: ^ ∗ θ ± T1−α/2σθ^ ^ ^ ∗ where θ is our estimate of θ, σθ^ is the standard error of θ, and T1−α/2 is ∗ the critical value of the test statistic, i.e., P(T ≤ T1−α/2) = 1 − α=2. Interpreting Confidence Intervals: Correct: through repeated samples, e.g., 99 out of 100 confidence intervals would be expected to contain true θ with α = :01 Wrong: through one sample; e.g., there is a 99% chance the confidence interval around my θ^ contains the true θ (with α = :01) Nathaniel E. Helwig (U of Minnesota) Nonparametric Location Tests: One-Sample Updated 04-Jan-2017 : Slide 6 Background Information Location Tests Definition of “Location Test” Allow us to test hypotheses about mean or median of a population. There are one-sample tests and two-sample tests. One-Sample: H0 : µ = µ0 vs. H1 : µ 6= µ0 Two-Sample: H0 : µ1 − µ2 = µ0 vs. H1 : µ1 − µ2 6= µ0 There are one-sided tests and two-sided tests. One-Sided: H0 : µ = µ0 vs. H1 : µ < µ0 or H1 : µ > µ0 Two-Sided: H0 : µ = µ0 vs. H1 : µ 6= µ0 Nathaniel E. Helwig (U of Minnesota) Nonparametric Location Tests: One-Sample Updated 04-Jan-2017 : Slide 7 Background Information Location Tests Problems with Parametric Location Tests Typical parametric location tests (e.g., Student’s t tests) focus on analyzing mean differences. Robustness: sample mean is not robust to outliers Consider a sample of data x1;:::; xn with expectation µ < 1 Suppose we fix x1; x2;:::; xn−1 and let xn ! 1 ¯ 1 Pn Note x = n i=1 xi ! 1, i.e., one large outlier ruins sample mean Generalizability: parametric tests are meant for particular distributions Assume data are from some known distribution Parametric inferences are invalid if assumption is wrong Nathaniel E. Helwig (U of Minnesota) Nonparametric Location Tests: One-Sample Updated 04-Jan-2017 : Slide 8 Background Information Order and Rank Statistics Order Statistics Given a sample of data X1; X2; X3;:::; Xn from some cdf F, the order statistics are typically denoted by X(1); X(2); X(3);:::; X(n) where X(1) ≤ X(2) ≤ X(3) ≤ · · · ≤ X(n) are the ordered data Note that. the 1st order statistic X(1) is the sample minimum the n-th order statistic X(n) is the sample maximum Nathaniel E. Helwig (U of Minnesota) Nonparametric Location Tests: One-Sample Updated 04-Jan-2017 : Slide 9 Background Information Order and Rank Statistics Rank Statistics Given a sample of data X1; X2; X3;:::; Xn from some cdf F, the rank statistics are typically denoted by R1; R2; R3;:::; Rn where Ri 2 [1; n] for all i 2 f1;:::; ng are the data ranks If there are no ties (i.e., if Xi 6= Xj 8i; j), then Ri 2 f1;:::; ng Nathaniel E. Helwig (U of Minnesota) Nonparametric Location Tests: One-Sample Updated 04-Jan-2017 : Slide 10 Background Information Order and Rank Statistics Order and Rank Statistics: Example (No Ties) Given a sample of data X1 = 3; X2 = 12; X3 = 11; X4 = 18; X5 = 14; X6 = 10 from some cdf F, the order statistics are X(1) = 3; X(2) = 10; X(3) = 11; X(4) = 12; X(5) = 14; X(6) = 18 and the ranks are given by R1 = 1; R2 = 4; R3 = 3; R4 = 6; R5 = 5; R6 = 2 Nathaniel E. Helwig (U of Minnesota) Nonparametric Location Tests: One-Sample Updated 04-Jan-2017 : Slide 11 Background Information Order and Rank Statistics Order and Rank Statistics: Example (With Ties) Given a sample of data X1 = 3; X2 = 11; X3 = 11; X4 = 14; X5 = 14; X6 = 11 from some cdf F, the order statistics are X(1) = 3; X(2) = 11; X(3) = 11; X(4) = 11; X(5) = 14; X(6) = 14 and the ranks are given by R1 = 1; R2 = 3; R3 = 3; R4 = 5:5; R5 = 5:5; R6 = 3 This is fractional ranking where we use average ranks: Replace Ri 2 f2; 3; 4g with the average rank 3 = (2 + 3 + 4)=3 Replace Ri 2 f5; 6g with the average rank 5:5 = (5 + 6)=2 Nathaniel E. Helwig (U of Minnesota) Nonparametric Location Tests: One-Sample Updated 04-Jan-2017 : Slide 12 Background Information Order and Rank Statistics Order and Rank Statistics: Examples (in R) Revisit example with no ties: > x = c(3,12,11,18,14,10) > sort(x) [1] 3 10 11 12 14 18 > rank(x) [1] 1 4 3 6 5 2 Revisit example with ties: > x = c(3,11,11,14,14,11) > sort(x) [1] 3 11 11 11 14 14 > rank(x) [1] 1.0 3.0 3.0 5.5 5.5 3.0 Nathaniel E. Helwig (U of Minnesota) Nonparametric Location Tests: One-Sample Updated 04-Jan-2017 : Slide 13 Background Information Order and Rank Statistics Summation of Integers (Carl Gauss) Carl Friedrich Gauss was a German mathematician who made amazing contributions to all areas of mathematics (including statistics). According to legend, when Carl was in primary school (about 8 y/o) the teacher asked the class to sum together all integers from 1 to 100. This was supposed to occupy the students for several hours After a few seconds, Carl wrote down the correct answer of 5050! Carl noticed that 1 + 100 = 101, 2 + 99 = 101, 3 + 98 = 101, etc. P100 There are 50 pairs that sum to 101 =) i=1 i = 5050 Nathaniel E. Helwig (U of Minnesota) Nonparametric Location Tests: One-Sample Updated 04-Jan-2017 : Slide 14 Background Information Order and Rank Statistics General Summation Formulas Pn Summation of Integers: i=1 i = n(n + 1)=2 From pattern noticed by Carl Gauss Pn 2 Summation of Squares: i=1 i = n(n + 1)(2n + 1)=6 Can prove using difference approach (similar to Carl Gauss idea) These formulas relate to test statistics that we use for rank data. Nathaniel E. Helwig (U of Minnesota) Nonparametric Location Tests: One-Sample Updated 04-Jan-2017 : Slide 15 Background Information One-Sample Problem Problem(s) of Interest For the one-sample location problem, we could have: Paired-replicates data: (Xi ; Yi ) are independent samples One-sample data: Zi are independent samples We want to make inference about: Paired-replicates data: difference in location (treatment effect) One-sample data: single population’s location Nathaniel E. Helwig (U of Minnesota) Nonparametric Location Tests: One-Sample Updated 04-Jan-2017 : Slide 16 Background Information One-Sample Problem Typical Assumptions Independence assumption: Paired-replicates data: Zi = Yi − Xi are independent samples One-sample data: Zi are independent samples Symmetry assumption: Paired-replicates data: Zi ∼ Fi which is continuous and symmetric around θ (common median) One-sample data: Zi ∼ Fi which is continuous and symmetric around θ (common median) Nathaniel E. Helwig (U of Minnesota) Nonparametric Location Tests: One-Sample Updated 04-Jan-2017 : Slide 17 Wilcoxon’s Signed Rank Test Wilcoxon’s Signed Rank Test Nathaniel E. Helwig (U of Minnesota) Nonparametric Location Tests: One-Sample Updated 04-Jan-2017 : Slide 18 Wilcoxon’s Signed Rank Test Overview Assumptions and Hypothesis Assumes both independence and symmetry. The null hypothesis about θ (common median) is H0 : θ = θ0 and we could have one of three alternative hypotheses: One-Sided Upper-Tail: H1 : θ > θ0 One-Sided Lower-Tail: H1 : θ < θ0 Two-Sided: H1 : θ 6= θ0 Nathaniel E. Helwig (U of Minnesota) Nonparametric Location Tests: One-Sample Updated 04-Jan-2017 : Slide 19 Wilcoxon’s Signed Rank Test Hypothesis Testing Test Statistic Let Ri for i 2 f1;:::; ng denote the ranks of jZi − θ0j. Defining the indicator variable 1 if Zi − θ0 > 0 i = 0 if Zi − θ0 < 0 the Wilcoxon signed rank test statistic T + is defined as n + X T = Ri i i=1 where Ri i is the positive signed rank of Zi − θ0 Nathaniel E.
Details
-
File Typepdf
-
Upload Time-
-
Content LanguagesEnglish
-
Upload UserAnonymous/Not logged-in
-
File Pages63 Page
-
File Size-