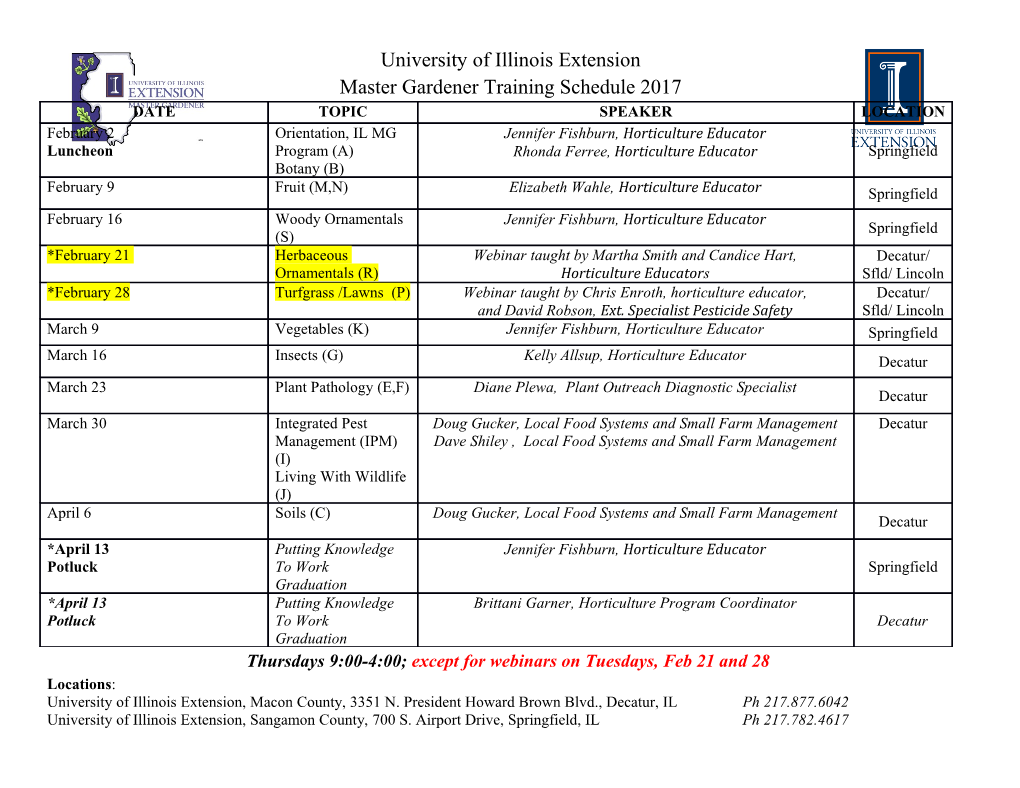
Detecting Shibboleths Jelena Prokic´ C¸agrı˘ C¸ oltekin¨ John Nerbonne Ludwig-Maximilians-Universitat¨ University of Groningen University of Groningen [email protected] [email protected] [email protected] Abstract EAS. But as Chambers & Trudgill (1998) note, the earlier methodology is fraught with prob- A SHIBBOLETH is a pronunciation, or, lems, many of which stem from the freedom of more generally, a variant of speech that choice with respect to isoglosses, and their (nor- betrays where a speaker is from (Judges mal) failure to ‘bundle’ neatly. Nerbonne (2009) 12:6). We propose a generalization of the well-known precision and recall scores to notes that dialectometry improves on the tradi- deal with the case of detecting distinctive, tional techniques in many ways, most of which characteristic variants when the analysis is stem from the fact that it shifts focus to AGGRE- based on numerical difference scores. We GATE LEVEL of differences. Dialectometry uses also compare our proposal to Fisher’s linear large amounts of material; it reduces the sub- discriminant, and we demonstrate its effec- jectivity inherent in choosing isoglosses; it fre- tiveness on Dutch and German dialect data. quently analyzes material in ways unintended by It is a general method that can be applied both in synchronic and diachronic linguis- those who designed dialect data collection efforts, tics that involve automatic classification of including more sources of differences; and finally linguistic entities. it replaces search for categorical overlap by a sta- tistical analysis of differences. 1 Introduction and Background Dialectometry does not enjoy overwhelming The background of this contribution is the line of popularity in dialectology, however, and one of work known as DIALECTOMETRY (Seguy,´ 1973; the reasons is simply that dialectologists, but also Goebl, 1982), which has made computational laymen, are interested not only in the aggregate work popular in dialectology. The basic idea of relations among sites, or even the determination dialectometry is simple: one acquires large sam- of dialect areas (or the structure of other geo- ples of corresponding material (e.g., a list of lex- graphic influence on language variation, such as ical choices, such as the word for carbonated dialect continua), but are quite enamored of the soft drink, which might be ‘soda’, ‘pop’, ‘tonic’ details involved. Dialectology scholars, but also etc.) from different sites within a language area, laymen, wish to now where ‘coffee’ is ordered (in w and then, for each pair of samples, one counts English) with a labialized /k/ sound ([k Ofi]) or where in Germany one is likely to hear [p] and (or more generally measures) the difference at > each point of correspondence. The differences where [pf] in words such as Pfad ‘path’ or Pfund are summed, and, given representative and suffi- ‘pound’. ciently large samples, the results characterizes the Such characteristic features are known as SHIB- degree to which one site differs from another. BOLETHS, following a famous story in the old Earlier work in dialectology mapped the dis- testament where people were killed because of tributions of individual items, recording lines of where they were from, which was betrayed by division on maps, so-called ISOGLOSSES, and their inability to pronounce the initial [S] in the then sought bundles of these as tell-tale indica- word ‘shibboleth’ (Judges 12:6). We propose a tors of important divisions between DIALECT AR- generalization of the well-known precision and 72 Proceedings of the EACL 2012 Joint Workshop of LINGVIS & UNCLH, pages 72–80, Avignon, France, April 23 - 24 2012. c 2012 Association for Computational Linguistics recall scores, appropriate when dealing with dis- introduced a correction for ‘chance instantiation’. tances, and which are designed to detect distinc- This is derived from the relative size of the group tive, characteristic variants when the analysis is in question: based on numerical difference scores. We also RelSize(g) = |g| compare our proposal to Fisher’s linear discrim- |G| inant, and we demonstrate its effectiveness on |gf | Dutch and German dialect data. Finally we eval- RelOcc(f, g) = |Gf | uate the success of the proposal by visually ex- amining an MDS plot showing the distances one RelOcc(f,g)−RelSize(g) Distinct(f, g) = 1− (g) obtains when the analysis is restricted to the fea- RelSize tures determined to be characteristic. where, G is the set of sites in the larger area of The paper proceeds from a dialectometric per- interest. spective, but the technique proposed does not as- As a consequence, smaller clusters are given sume an aggregate analysis, only that a group of larger scores than clusters that contain many ob- sites has been identified somehow or another. The jects. Distinctiveness may even fall below zero, task is then to identify characteristic features of but these will be very uninteresting cases — those (candidate) dialect areas. which occur relatively more frequently outside the group under consideration than within it. 1.1 Related Work Critique Wieling and Nerbonne (2011) introduced two measures seeking to identify elements character- There are two major problems with the earlier istic of a given group, REPRESENTATIVENESS formulation which we seek to solve in this pa- and DISTINCTIVENESS. The intuition behind rep- per. First, the formulation, if taken strictly, applies resentativeness is simply that a feature increases only to individual values of categorical features, in representativeness to the degree that it is found not to the features themselves. Second, many at each site in the group. We simplify their defi- dialectological analyses are based on numerical nition slightly as they focus on sound correspon- measures of feature differences, e.g., the edit dis- dences, i.e. categorical variables, while we shall tance between two pronunciation transcriptions or formulate ideas about features in general. the distance in formant space between two vowel pronunciations (Leinonen, 2010). |gf | We seek a more general manner of detecting Representativeness(f, g) = |g| characteristic features below, i.e. one that applies to features, and not just to their (categorical) val- where f is a feature (in their case sound corre- ues and, in particular, one that can work hand in spondence) in question, g is the set of sites in a hand with numerical measures of feature differ- f given cluster, and g denotes the set of sites where ences. feature f is observed. As Wieling (2012) notes, if one construes the 2 Characteristic Features sites in the given group as ‘relevant documents’ Since dialectometry is built on measuring differ- and features as ‘queries’, then this definition is ences, we assume this in our formulation, and we equivalent to RECALL in information retrieval seek those features which differ little within the (IR). group in question and a great deal outside that The intuition behind distinctiveness is similar group. We focus on the setting where we exam- to that behind IR’s PRECISION, which measures ine one candidate group at a time, seeking fea- the fraction of positive query responses that iden- tures which characterize it best in distinction to tify relevant documents. In our case this would be elements outside the group. the fraction of those sites instantiating a feature We assume therefore, as earlier, a group g that that are indeed in the group we seek to character- we are examining consisting of |g| sites among a ize. In the case of groups of sites in dialectologi- larger area of interest G with |G| sites including cal analysis, however, we are dealing with groups the sites s both within and outside g. We further that may make up significant fractions of the en- explicitly assume a measure of difference d be- tire set of sites. Wieling and Nerbonne therefore tween sites, always with respect to a given feature 73 f. Then we calculate a mean difference with re- spect to f within the group in question: 2 X d¯g = d (s, s0) f |g|2 − |g| f S s,s0∈g and a mean difference with respect f involving elements from outside the group: ¯ 1 X Figure 1: Illustration of the calculation of a distance d6g = d (s, s0) f |g|(|G| − |g|) f function. Our proposal compares the mean distance s∈g,s06∈g of all pairs of sites within a group, including all those shown on the left (in blue) to the mean distance of the We then propose to identify characteristic features pairs of sites where the first is within the group and the as those with relatively large differences between second outside it. ¯6g ¯g df and df . However, we note that scale of these calculations are sensitive to a number of factors, may affect the scale and the reliability of the av- including the size of the group and the number of erage distance calculations presented above. For individual differences calculated (which may vary the experiments reported below, we calculated av- due to missing values). To remedy the difficul- erage scores only if the missing values did not ex- ties of comparing different features, and possibly ceed 20% of the total values used in the calcula- very different distributions, we standardize both tion. ¯6g ¯g df and df and calculate the difference between Fisher’s Linear Discriminant the z-scores, where mean and standard deviation of the difference values are estimated from all dis- The formulation we propose looks a good deal tance values calculated with respect to feature f. like the well-known Fisher’s linear discriminant As a result, we use the measure (FLD) (Schalkoff, 1992, 90ff), which maximizes the differences in means between two data sets ¯6g ¯ ¯g ¯ with respect to (the sum of) their variances. d − df d − df f − f sd(df ) sd(df ) 2 σbetween S = 2 where df represents all distance values with re- σwithin spect to feature f (the formula is not simplified But FLD is defined for vectors, while we wish for the sake of clarity).
Details
-
File Typepdf
-
Upload Time-
-
Content LanguagesEnglish
-
Upload UserAnonymous/Not logged-in
-
File Pages9 Page
-
File Size-