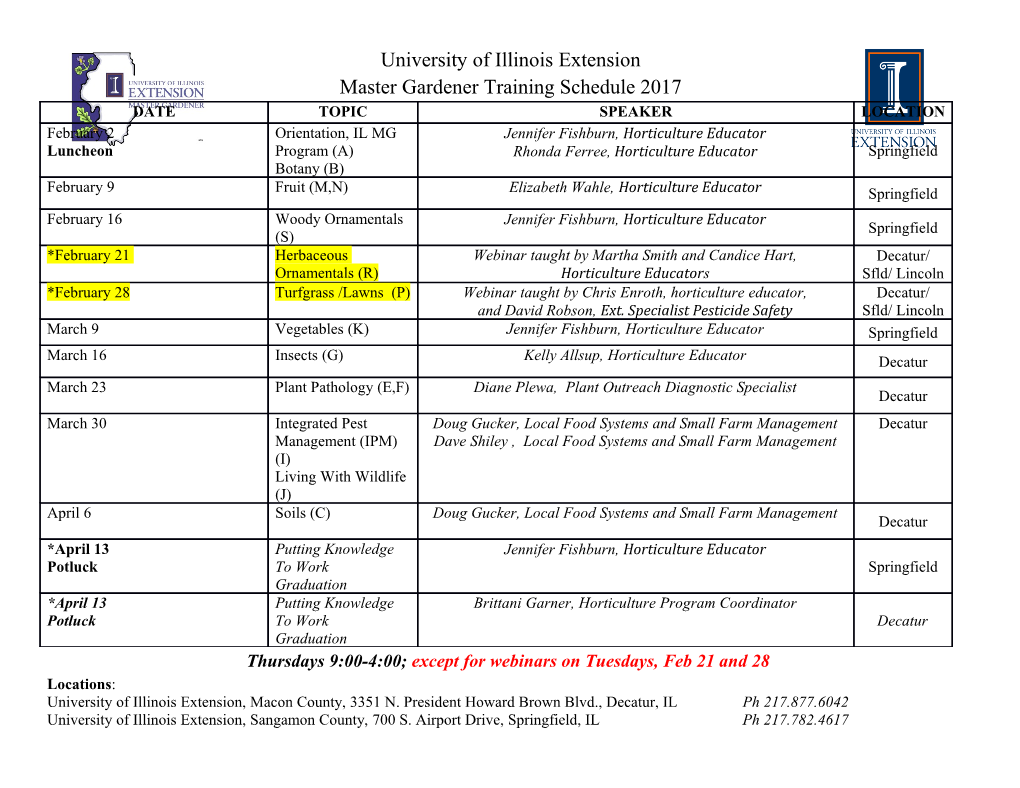
Fakultät für Medizin Deutsches Herzzentrum München - Klinik an der Technischen Universität München Understanding the genetics of coronary artery disease through novel statistical approaches Lingyao Zeng Vollständiger Abdruck der von der Fakultät für Medizin der Technischen Universität München zur Erlangung des akademischen Grades eines Doctor of Philosophy (Ph.D.) genehmigten Dissertation. Vorsitzende/r: Prof. Dr. Dr. Stefan Engelhardt Betreuer/in: Prof. Dr. Adnan Kastrati Prüfer der Dissertation: 1. Prof. Dr. Heribert Schunkert 2. Prof. Dr. Dr. Fabian Theis Die Dissertation wurde am 29.07.2016 bei der Fakultät für Medizin der Technischen Universität München eingereicht und durch die Fakultät für Medizin am 14.09.2016 angenommen. Acknowledgement First of all, I would like to express my sincere gratitude and thanks to my advisor Prof. Heribert Schunkert. It is my honor to join his research group. He has been actively interested in my work and offered tremendous support and guidance at all levels of my PhD research over the last few years. I greatly appreciate all his knowledge, kindness, enthusiasm, and encouragement. I would like to thank the other members of my committee, Prof. Adnan Kastrati and Prof. Fabian Theis. I thank Prof. Adnan Kastrati, who as my supervisor has given me the opportunity to pursue my PhD research at the Deutsches Herzzentrum München and Technische Universität München. I also thank him for the generosity and patience offering the clinical data and descriptions. I thank Prof. Fabian Theis for all his valuable comments and encouragement at both the committee meetings and e:AtheroSysMed project conferences. I am grateful to Prof. Bertram Müller-Myhsok from the Department of Statistical Genetics, Max Planck Institute of Psychiatry, and his team member Dr. Nazanin Mirza- Schreiber, for their collaborative efforts in the epistasis project. We have spent much time together in co-running the analysis, trouble shooting, and stimulating discussions. Also many thanks to Dr. Till Andlauer who has offered us his scripts for a scrutinized genotype QC and imputation procedure. My sincere thanks also goes to Prof. Jeanette Erdmann, who has warmly hosted me at Institut für Integrative und Experimentelle Genomik, Universität zu Lübeck, in my i early days of PhD research, and to Dr. Christina Willenborg in her team for her help with all data issues. I wish to thank Prof. Inke König for well hosting me at Institut für Medizinische Biometrie und Statistik, Universität zu Lübeck, in my early days of PhD research as well, and Dr. Christina Loley in her team for the patient instructions on basic data processing. I specially thank my colleague Dr. Thorsten Keßler for his careful reviewing this thesis and offering comments and advices. Special thanks also goes to my colleague Dr. Barbara Stiller, who has largely helped in proof-reading and offered great suggestions. I also thank all my other colleagues in AG Schunkert for contributing to the nice working atmosphere, stimulative discussion and supportive collaboration. Grateful thanks to all my friends for always supporting me and for all the good times we have had. Finally, I would like to sincerely thank my parents who have given me inspiration, encourage, and enlightenment over all years of my life. ii Abstract Coronary artery disease (CAD) is a complex disease with a strong genetic component. A better understanding of the molecular-genetic basis of CAD susceptibility is of vital importance for better prevention and treatment of this multifactorial and often lethal disease. The study of human genomes on a genome-wide scale may help to uncover novel genes involved in the pathophysiology and therefore broaden the knowledge from a systematic perspective. With the development of genotyping techniques and the progress of the Human 1000 Genome Project (1000G), quantification of variations on a genome- wide scale became feasible, which not only provides a powerful molecular repository for identifying complex genetic architectures of multifactorial diseases but also presents unprecedented data analysis challenges. The aim of this thesis was to assess the possible genetic impact on the susceptibility of CAD using quantitative and statistical genetics research approaches for three aspects: 1. to conduct genome-wide association studies (GWAS) for CAD in the 1000G era; 2. to contribute to the understanding of the genetic complexity of GWAS signals of CAD; and 3. to detect whether CAD risk is modified by the interaction of different variants. The first part of my research included GWAS analyses with the aim to uncover additional risk loci in the 1000G era. Specifically, I performed GWAS analyses after 1000G imputation on four German cohort studies based on the whole autosomal genome (1000G CAD GWAS), and also on one German cohort focused on the X-chromosome (1000G CAD X-Chr). The resultant summary statistics of these analyses served as iii valuable components to the CARDIoGRAMplusC4D consortium, which has organized international collaborative efforts for the meta-analysis of genome-wide associations studies. So far one such meta-analysis of 1000G CAD GWAS, which was finally based on more than 185,000 CAD cases and controls and identified 10 novel CAD-associated loci, has been published. Further publications are under review. Typically, only the best SNP at each locus from GWAS is reported. However, as typical for a complex disease, the genetic architecture of CAD is not monogenic. On one hand, multiple genes/loci are contributing to the CAD susceptibility. Within each of them there may also exist allelic heterogeneity. On the other hand, the genetic susceptibility loci to other complex traits may also contribute to CAD risk, due to a potentially shared genetic and functional background. The second part of my thesis was therefore to investigate the genetic complexity of GWAS signals of CAD, with the aim to examine and confirm - based on the individual-level genotype data - both the intra-locus allelic heterogeneity within known CAD loci and the multi-locus polygenic pleiotropic effect from susceptible loci of other traits. At known CAD loci, I collected individual-level genotype data from eight cohorts including over 10,000 cases and 10,000 controls, and examined the additive effect of multiple alleles, which were found to be independently associated with CAD risk at the respective locus based on a multi-locus polygenic score (PGS) approach. Indeed, at some loci multiple independent signals could be recovered with a combined effect that conferred incremental risk of CAD with the increase of the number of independent risk alleles. The results improved our understanding of the allelic structure at known CAD-associated loci, and also highlighted the importance and complexity of genetic heterogeneity. To investigate the potential pleiotropic effect of the genetic susceptible loci of other traits on CAD, I further constructed multi-locus polygenic scores for height and rheumatoid arthritis (RA) based on five and seven individual-level genotype datasets, respectively, and examined their effect on CAD onset. The results helped to support the notion that height directly and indirectly affects CAD iv risk, as well as that genetic factors underlying RA carry a low likelihood to affect CAD risk. My analysis contributed to the respective publications. GWAS are usually analyzed with the assumption that the genetic variants involved in a complex disease act independently and that their combined single effects are responsible for the observed phenotypes. It has now been accepted that epistatic effects, i.e., gene- gene interactions, may also play a significant role in determining complex traits. Limited by methodological issues, GWAS signals usually do not cover the higher order genetic architecture underlying CAD risk. The third part of my thesis was thus dedicated to detect gene-gene interactions. So far no large-scale systematic investigation of epistasis had been made in the context of CAD, mainly due to the challenge in both computation power and sample size. To enable such analysis, I collected individual-level genotype data of nine cohorts including 27,360 individuals, and, in collaboration with Max Planck Institute of Psychiatry, implemented the computation with a powerful GPU-based parallel computing tool. By strategy, I started with a searching space of broad sense CAD susceptibility regions, and investigated two-variant statistical epistasis assuming all possible genetic models. For each statistically significant epistasis pair, I subsequently searched for potential biological epistasis. Finally, I postulated a novel hypothesis on how genetic loci could convey their epistatic effect, firstly through perturbation of nuclear protein interactions, and secondly through perturbation of downstream pathways. These epistasis results make a great extension to our current knowledge of CAD genetics. The applied scheme and the GPU-based parallel computing tool may also enable researchers to further explore CAD epistasis at a genome-wide scale in the future. In summary, the studies in my thesis, including statistical genetics approaches such as GWAS analyses, polygenic score calculation, and epistasis investigation, made efforts and contributions to the improvement of our understanding of the genetic etiology of CAD from several perspectives. v vi Contents Abstract v List of Abbreviations xii List of Figures xiv List of Tables xv 1 Introduction 1 1.1 Background of statistical genetics research in CAD . 1 1.1.1 CAD is a complex disease with a strong genetic component . 1 1.1.2 Human genetic variations and 1000G project . 2 1.1.3 Genotyping arrays . 4 1.2 Genome-Wide Association Studies . 4 1.2.1 What is a GWAS analysis? . 4 1.2.2 Previous achievements and open challenges . 5 1.3 Genetic complexity of GWAS signals of CAD . 6 1.3.1 What is intra-locus allelic heterogeneity? . 6 1.3.2 What is multi-locus polygenic pleiotropy? . 7 1.3.3 Previous achievements and open challenges . 7 1.4 Epistasis . 8 vii 1.4.1 What is epistasis? . 8 1.4.2 Previous achievements and open challenges .
Details
-
File Typepdf
-
Upload Time-
-
Content LanguagesEnglish
-
Upload UserAnonymous/Not logged-in
-
File Pages159 Page
-
File Size-