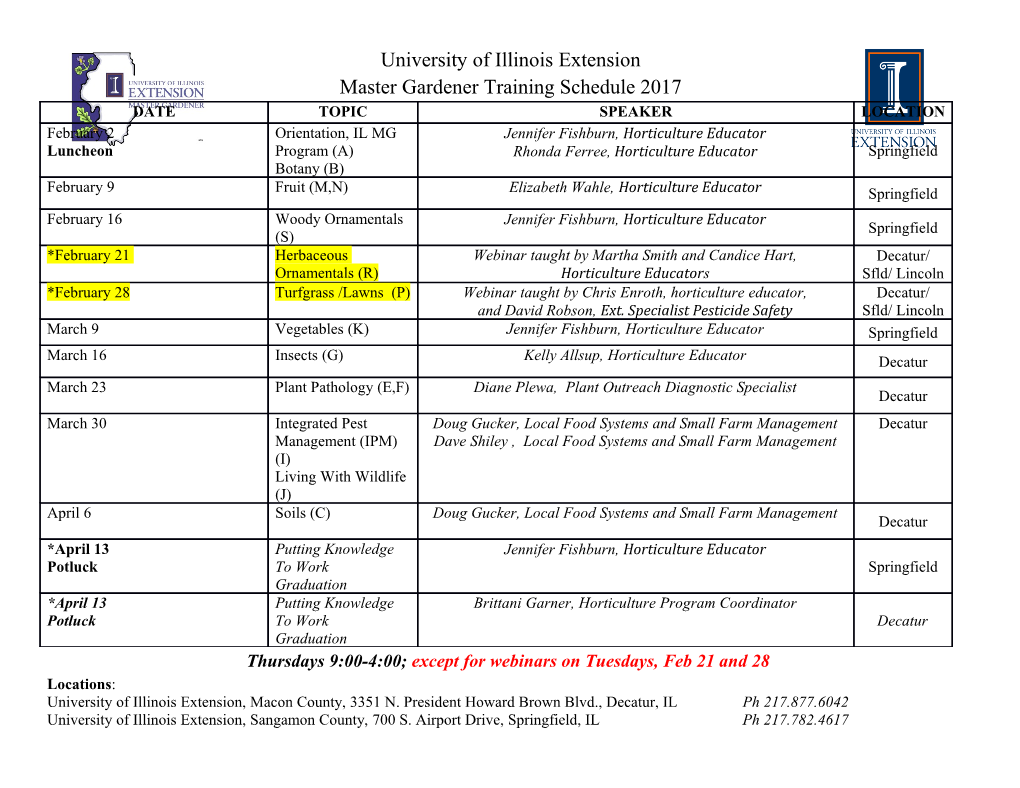
MimicNet: Fast Performance Estimates for Data Center Networks with Machine Learning Qizhen Zhang, Kelvin K.W. Ng, Charles W. Kazerx, Shen Yany, João Sedoc⋄, and Vincent Liu University of Pennsylvania, xSwarthmore College, yPeking University, ⋄New York University {qizhen,kelvinng,liuv}@seas.upenn.edu, [email protected], [email protected], ⋄[email protected] ABSTRACT 0.7 Small-scale Flow-level MimicNet At-scale evaluation of new data center network innovations is 0.6 becoming increasingly intractable. This is true for testbeds, where 0.5 0.4 few, if any, can afford a dedicated, full-scale replica of a data center. 0.3 It is also true for simulations, which while originally designed 0.2 for precisely this purpose, have struggled to cope with the size of Truth Ground to 1 0.1 today’s networks. W 0 This paper presents an approach for quickly obtaining accurate 4 8 16 32 64 128 Network Size (#Clusters) performance estimates for large data center networks. Our system, MimicNet, provides users with the familiar abstraction of a packet- Figure 1: Accuracy for MimicNet’s predictions of the FCT level simulation for a portion of the network while leveraging distribution for a range of data center sizes. Accuracy is redundancy and recent advances in machine learning to quickly quantified via the Wasserstein distance (W1) to the distri- and accurately approximate portions of the network that are not bution observed in the original simulation. Lower is better. directly visible. MimicNet can provide over two orders of magnitude Also shown are the accuracy of a flow-level simulator (Sim- speedup compared to regular simulation for a data center with Grid) and the accuracy of assuming a small (2-cluster) simu- thousands of servers. Even at this scale, MimicNet estimates of the lation’s results are representative. tail FCT, throughput, and RTT are within 5% of the true results. interconnected and filled with dependencies, are particularly chal- CCS CONCEPTS lenging in that regard—small changes in one part of the network • Networks → Network simulations; Network performance mod- can result in large performance effects in others. eling; Network experimentation; • Computing methodologies → Unfortunately, full-sized testbeds that could capture these effects Massively parallel and high-performance simulations; are prohibitively expensive to build and maintain. Instead, most pre- KEYWORDS production performance evaluation comprises orders of magnitude fewer devices and fundamentally different network structures. This Network simulation, Data center networks, Approximation, Ma- is true for (1) hardware testbeds [47], which provide total control of chine learning, Network modeling the system, but at a very high cost; (2) emulated testbeds [43, 54, 56], ACM Reference Format: which model the network but at the cost of scale or network effects; Qizhen Zhang, Kelvin K.W. Ng, Charles W. Kazerx, Shen Yany, João Sedoc⋄, and (3) small regions of the production network, which provide and Vincent Liu. 2021. MimicNet: Fast Performance Estimates for Data Cen- ‘in vivo’ accuracy but force operators to make a trade-off between ter Networks with Machine Learning. In ACM SIGCOMM 2021 Conference scale and safety [48, 59]. The end result is that, often, the only way (SIGCOMM ’21), August 23–27, 2021, Virtual Event, USA. ACM, New York, to ascertain the true performance of the system at scale is to deploy NY, USA, 18 pages. https://doi.org/10.1145/3452296.3472926 it to the production network. 1 INTRODUCTION We note that simulation was originally intended to fill this gap. Over the years, many novel protocols and systems have been pro- In principle, simulations provide an approximation of network be- posed to improve the performance of data center networks [5– havior for arbitrary architectures at an arbitrary scale. In practice, 7, 12, 19, 33, 39]. Though innovative in their approaches and promis- however, modern simulators struggle to provide both simultane- ing in their results, these proposals suffer from a consistent chal- ously. As we show in this paper, even for relatively small networks, lenge: the difficulty of evaluating systems at scale. Networks, highly packet-level simulation is 3–4 orders of magnitude slower than real- time (5 min of simulated time every ∼3.2 days); larger networks Permission to make digital or hard copies of all or part of this work for personal or can easily take months or longer to simulate. Instead, researchers classroom use is granted without fee provided that copies are not made or distributed for profit or commercial advantage and that copies bear this notice and the full citation often either settle for modestly sized simulations and assume that on the first page. Copyrights for components of this work owned by others than the performance translates to larger deployments, or they fall back to author(s) must be honored. Abstracting with credit is permitted. To copy otherwise, or republish, to post on servers or to redistribute to lists, requires prior specific permission approaches that ignore packet-level effects like flow approximation and/or a fee. Request permissions from [email protected]. techniques. Both sacrifice substantial accuracy. SIGCOMM ’21, August 23–27, 2021, Virtual Event, USA In this paper, we describe MimicNet, a tool for fast performance © 2021 Copyright held by the owner/author(s). Publication rights licensed to ACM. estimation of at-scale data center networks. MimicNet presents to ACM ISBN 978-1-4503-8383-7/21/08...$15.00 https://doi.org/10.1145/3452296.3472926 users the abstraction of a packet-level simulator; however, unlike 287 SIGCOMM ’21, August 23–27, 2021, Virtual Event, USA Q. Zhang et al. existing simulators, MimicNet only simulates—at a packet level— 0.1 the traffic to and from a single ‘observable’ cluster, regardless ofthe Single Thread One Machine Second actual size of the data center. Users can then instrument the host and / Two Machines Four Machines network of the designated cluster to collect arbitrary statistics. For 0.01 the remaining clusters and traffic that are not directly observable, Seconds MimicNet approximates their effects with the help of deep learning 0.001 models and flow approximation techniques. As a preview of MimicNet’s evaluation results, Figure 1 shows the accuracy of its Flow-Completion Time (FCT) predictions for various Simulation 0.0001 0 10 20 30 40 50 60 70 data center sizes and compares it against two common alternatives: # of ToRs/Aggs (1) flow-level simulation and (2) running a smaller simulation and assuming that the results are identical for larger deployments. For Figure 2: OMNeT++ performance on leaf-spine topologies of each approach, we collected the simulated FCTs of all flows with various size. Even for these small cases, 5 mins of simulation at least one endpoint in the observable cluster. We compared the time can take multiple days to process. Results were similar distribution of each approach’s FCTs to that of a full-fidelity packet- for ns-3 and other simulation frameworks. level simulation using a ,1 metric. The topology and traffic pattern were kept consistent, except in the case of small-scale simulation • An architecture for composing Mimics into a generative model where that was not possible (instead, we fixed the average load and of a full-scale data center network. For a set of complex protocols packet/flow size). While MimicNet is not and will never be aperfect and real-world traffic patterns, MimicNet can match ground- × portrayal of the original simulation, it is 4.1 more accurate than truth results orders of magnitude more quickly than otherwise the other methods across network sizes, all while improving the possible. For large networks, MimicNet even outperforms flow- time to results by up to two orders of magnitude. level simulation in terms of speed (in addition to producing To achieve these results, MimicNet imposes a few carefully cho- much more accurate results). sen restrictions on the system being modeled: that the data center is • A customizable hyperparameter tuning procedure and loss func- built on a classic FatTree topology, that per-host network demand is tion design that ensure optimality in both generalization and a predictable a priori, that congestion occurs primarily on fan-in, and set of arbitrary user-defined objectives. that a given host’s connections are independently managed. These assumptions provide outsized benefits to simulator performance • Implementations and case studies of a wide variety of network and the scalability of its estimation accuracy, while still permitting protocols that stress MimicNet in different ways. application to a broad class of data center networking proposals, The framework is available at: https://github.com/eniac/MimicNet. both at the end host and in the network. Concretely, MimicNet operates as follows. First, it runs a sim- 2 MOTIVATION ulation of a small subset of the larger data center network. Using Modern data center networks connect up to hundreds of thousands the generated data, it trains a Mimic—an approximation of clus- of machines that, in aggregate, are capable of processing hundreds ters’ ‘non-observable’ internal and cross-cluster behavior. Then, of billions of packets per second. They achieve this via scale-out to predict the performance of an # cluster simulation, it carefully network architectures, and in particular, FatTree networks like the composes a single observable cluster with # − 1 Mimic’ed clusters one in Figure 3 [4, 18, 50]. In the canonical version, the network to form a packet-level generative model of a full-scale data center. consists of Top-of-Rack (ToR), Cluster, and Core switches. We refer Assisting with the automation of this training process is a hyper- to the components under a single ToR as a rack and the components parameter tuning stage that utilizes arbitrary user-defined metrics under and including a group of Cluster switches as a cluster.
Details
-
File Typepdf
-
Upload Time-
-
Content LanguagesEnglish
-
Upload UserAnonymous/Not logged-in
-
File Pages18 Page
-
File Size-