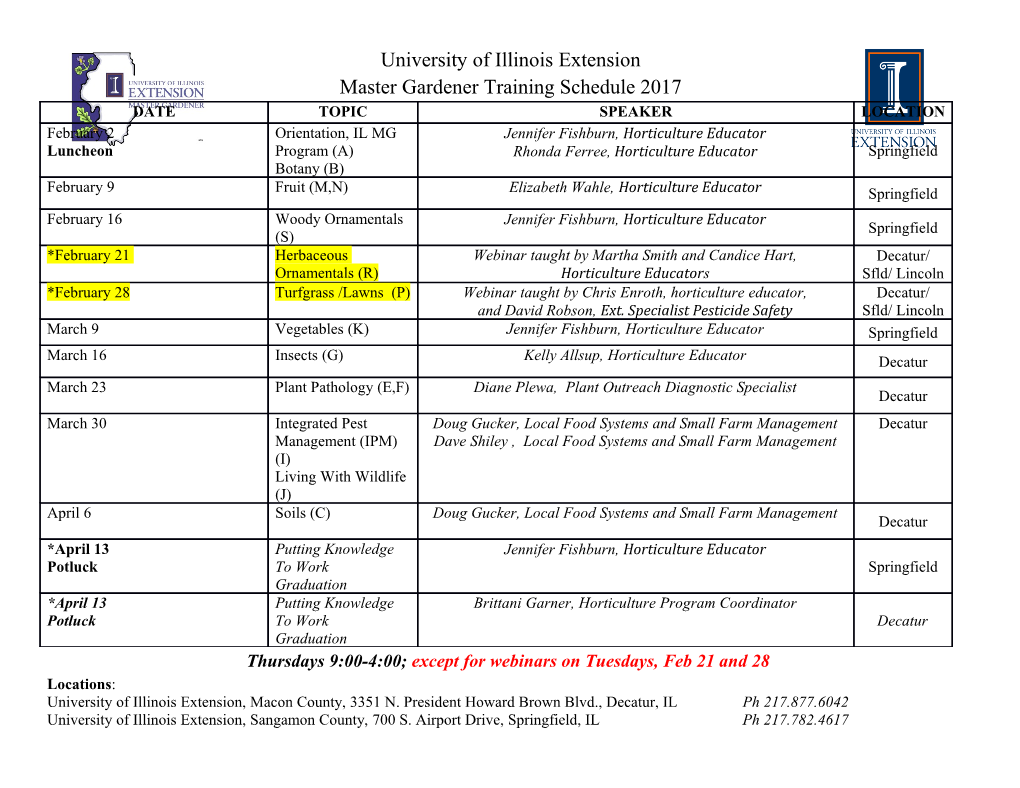
Lecture Notes for Econometrics 2002 (first year PhD course in Stockholm) Paul Söderlind1 June 2002 (some typos corrected and some material added later) 1University of St. Gallen. Address: s/bf-HSG, Rosenbergstrasse 52, CH-9000 St. Gallen, Switzerland. E-mail: [email protected]. Document name: EcmAll.TeX. Contents 1 Introduction 5 1.1 Means and Standard Deviation . 5 1.2 Testing Sample Means . 6 1.3 Covariance and Correlation . 8 1.4 Least Squares . 10 1.5 Maximum Likelihood . 11 1.6 The Distribution of ˇO .......................... 12 1.7 Diagnostic Tests . 14 1.8 Testing Hypotheses about ˇO ...................... 14 A Practical Matters 16 B A CLT in Action 17 2 Univariate Time Series Analysis 21 2.1 Theoretical Background to Time Series Processes . 21 2.2 Estimation of Autocovariances . 22 2.3 White Noise . 25 2.4 Moving Average . 25 2.5 Autoregression . 28 2.6 ARMA Models . 35 2.7 Non-stationary Processes . 36 3 The Distribution of a Sample Average 44 3.1 Variance of a Sample Average . 44 3.2 The Newey-West Estimator . 48 1 3.3 Summary . 50 4 Least Squares 53 4.1 Definition of the LS Estimator . 53 2 4.2 LS and R ............................... 55 4.3 Finite Sample Properties of LS . 57 4.4 Consistency of LS . 58 4.5 Asymptotic Normality of LS . 60 4.6 Inference . 63 4.7 Diagnostic Tests of Autocorrelation, Heteroskedasticity, and Normality 66 5 Instrumental Variable Method 74 5.1 Consistency of Least Squares or Not? . 74 5.2 Reason 1 for IV: Measurement Errors . 74 5.3 Reason 2 for IV: Simultaneous Equations Bias (and Inconsistency) . 76 5.4 Definition of the IV Estimator—Consistency of IV . 80 5.5 Hausman’s Specification Test ..................... 86 5.6 Tests of Overidentifying Restrictions in 2SLS . 87 6 Simulating the Finite Sample Properties 89 6.1 Monte Carlo Simulations . 89 6.2 Bootstrapping . 95 7 GMM 100 7.1 Method of Moments . 100 7.2 Generalized Method of Moments . 101 7.3 Moment Conditions in GMM . 101 7.4 The Optimization Problem in GMM . 104 7.5 Asymptotic Properties of GMM . 108 7.6 Summary of GMM . 113 7.7 Efficient GMM and Its Feasible Implementation . 114 7.8 Testing in GMM . 115 7.9 GMM with Sub-Optimal Weighting Matrix . 117 7.10 GMM without a Loss Function . 118 2 7.11 Simulated Moments Estimator . 119 8 Examples and Applications of GMM 122 8.1 GMM and Classical Econometrics: Examples . 122 8.2 Identification of Systems of Simultaneous Equations . 132 8.3 Testing for Autocorrelation . 134 8.4 Estimating and Testing a Normal Distribution . 138 8.5 Testing the Implications of an RBC Model . 142 8.6 IV on a System of Equations . 143 12 Vector Autoregression (VAR) 145 12.1 Canonical Form . 145 12.2 Moving Average Form and Stability . 146 12.3 Estimation . 149 12.4 Granger Causality . 149 12.5 Forecasts Forecast Error Variance . 150 12.6 Forecast Error Variance Decompositions . 151 12.7 Structural VARs . 152 12.8 Cointegration and Identification via Long-Run Restrictions . 165 12 Kalman filter 172 12.1 Conditional Expectations in a Multivariate Normal Distribution . 172 12.2 Kalman Recursions . 173 13 Outliers and Robust Estimators 179 13.1 Influential Observations and Standardized Residuals . 179 13.2 Recursive Residuals . 180 13.3 Robust Estimation . 182 13.4 Multicollinearity ............................184 14 Generalized Least Squares 187 14.1 Introduction . 187 14.2 GLS as Maximum Likelihood . 188 14.3 GLS as a Transformed LS . 191 14.4 Feasible GLS . 191 3 15 Nonparametric Regressions and Tests 193 15.1 Nonparametric Regressions . 193 15.2 Estimating and Testing Distributions . 201 16 Alphas /Betas and Investor Characteristics 208 16.1 Basic Setup . 208 16.2 Calendar Time and Cross Sectional Regression . 208 16.3 Panel Regressions, Driscoll-Kraay and Cluster Methods . 209 16.4 From CalTime To a Panel Regression . 216 16.5 The Results in Hoechle, Schmid and Zimmermann . 217 16.6 Monte Carlo Experiment . 219 16.7 An Empirical Illustration . 222 21 Some Statistics 226 21.1 Distributions and Moment Generating Functions . 226 21.2 Joint and Conditional Distributions and Moments . 228 21.3 Convergence in Probability, Mean Square, and Distribution . 231 21.4 Laws of Large Numbers and Central Limit Theorems . 233 21.5 Stationarity . 234 21.6 Martingales . 234 21.7 Special Distributions . 235 21.8 Inference . 246 22 Some Facts about Matrices 248 22.1 Rank . 248 22.2 Vector Norms . 248 22.3 Systems of Linear Equations and Matrix Inverses . 248 22.4 Complex matrices . 251 22.5 Eigenvalues and Eigenvectors . 252 22.6 Special Forms of Matrices . 252 22.7 Matrix Decompositions . 254 22.8 Matrix Calculus . 260 22.9 Miscellaneous . 263 4 1 Introduction 1.1 Means and Standard Deviation The mean and variance of a series are estimated as PT 2 PT 2 x t 1xt =T and t 1 .xt x/ =T: (1.1) N D D O D D N The standard deviation (here denoted Std.xt /), the square root of the variance, is the most common measure of volatility. The mean and standard deviation are often estimated on rolling data windows (for in- stance, a “Bollinger band” is 2 standard deviations from a moving data window around ˙ a moving average—sometimes used in analysis of financial prices.) If xt is iid (independently and identically distributed), then it is straightforward to find the variance of the sample average. Then, note that PT Á PT Var t 1xt =T t 1 Var .xt =T / D D D 2 T Var .xt / =T D Var .xt / =T: (1.2) D The first equality follows from the assumption that xt and xs are independently distributed (so the covariance is zero). The second equality follows from the assumption that xt and xs are identically distributed (so their variances are the same). The third equality is a trivial simplification. A sample average is (typically) unbiased, that is, the expected value of the sample average equals the population mean. To illustrate that, consider the expected value of the sample average of the iid xt PT PT E t 1xt =T t 1 E xt =T D D D E xt : (1.3) D The first equality is always true (the expectation of a sum is the sum of expectations), and 5 a. Distribution of sample average b. Distribution of √T times sample average 3 0.4 T=5 T=5 T T 2 =25 =25 T=50 T=50 T=100 0.2 T=100 1 0 0 −2 0 2 −5 0 5 Sample average √T times sample average Figure 1.1: Sampling distributions. This figure shows the distribution of the sample mean 2 and of pT times the sample mean of the random variable zt 1 where zt .1/. the second equality follows from the assumption of identical distributions which implies identical expectations. 1.2 Testing Sample Means The law of large numbers (LLN) says that the sample mean converges to the true popula- tion mean as the sample size goes to infinity. This holds for a very large class of random variables, but there are exceptions. A sufficient (but not necessary) condition for this con- vergence is that the sample average is unbiased (as in (1.3)) and that the variance goes to zero as the sample size goes to infinity (as in (1.2)). (This is also called convergence in mean square.) To see the LLN in action, see Figure 1.1. The central limit theorem (CLT) says that pT x converges in distribution to a normal N distribution as the sample size increases. See Figure 1.1 for an illustration. This also holds for a large class of random variables—and it is a very useful result since it allows us to test hypothesis. Most estimators (including LS and other methods) are effectively some kind of sample average, so the CLT can be applied. The basic approach in testing a hypothesis (the “null hypothesis”), is to compare the test statistics (the sample average, say) with how the distribution of that statistics (which is a random number since the sample is finite) would look like if the null hypothesis is true. For instance, suppose the null hypothesis is that the population mean is Suppose also that we know that distribution of the sample mean is normal with a known variance h2 (which will typically be estimated and then treated as if it was known). Under the null hypothesis, the sample average should then be N.; h2/. We would then reject the null 6 hypothesis if the sample average is far out in one the tails of the distribution. A traditional two-tailed test amounts to rejecting the null hypothesis at the 10% significance level if the test statistics is so far out that there is only 5% probability mass further out in that tail (and another 5% in the other tail). The interpretation is that if the null hypothesis is actually true, then there would only be a 10% chance of getting such an extreme (positive or negative) sample average—and these 10% are considered so low that we say that the null is probably wrong. Density function of N(0.5,2) Density function of N(0,2) 0.4 0.4 Pr(x ≤ −1.83) = 0.05 Pr(y ≤ −2.33) = 0.05 0.3 0.3 0.2 0.2 0.1 0.1 0 0 −4 −3 −2 −1 0 1 2 3 4 −4 −3 −2 −1 0 1 2 3 4 x y = x−0.5 Density function of N(0,1) 0.4 Pr(z ≤ −1.65) = 0.05 0.3 0.2 0.1 0 −4 −3 −2 −1 0 1 2 3 4 z = (x−0.5)/√2 Figure 1.2: Density function of normal distribution with shaded 5% tails.
Details
-
File Typepdf
-
Upload Time-
-
Content LanguagesEnglish
-
Upload UserAnonymous/Not logged-in
-
File Pages266 Page
-
File Size-