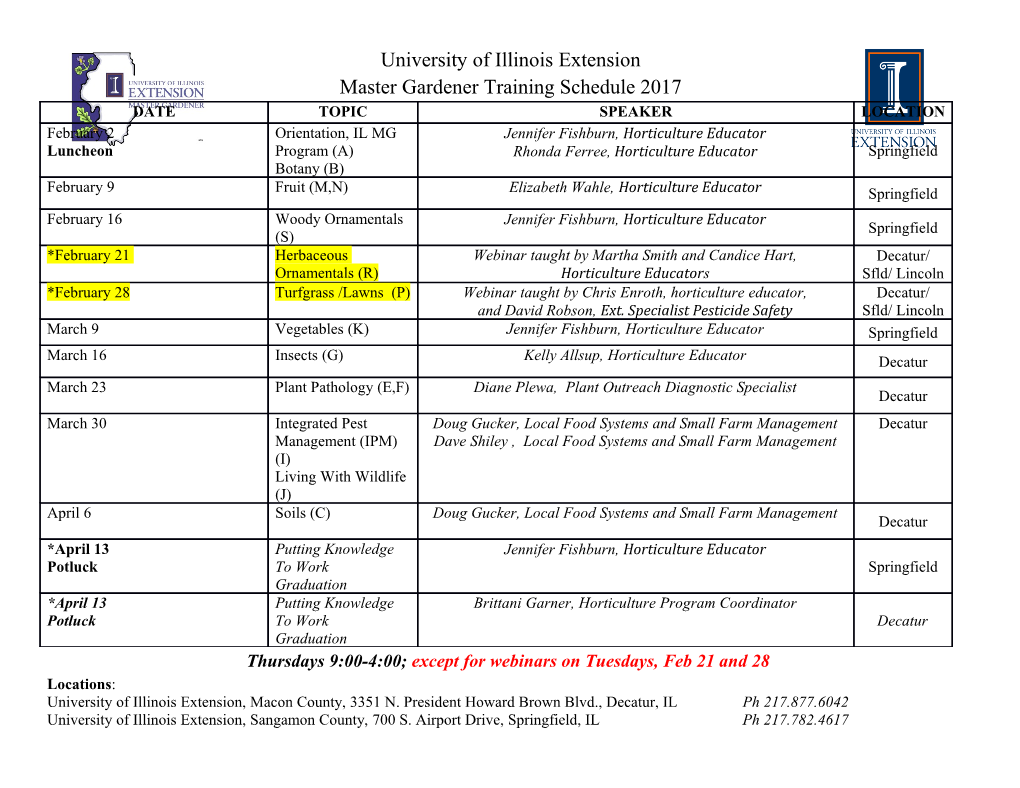
Supplementary Online Content Roussos P, Guennewig B, Kaczorowski DC, Barry G, Brennand KJ. Activity- dependent changes in gene expression in schizophrenia human-induced pluripotent stem cell neurons. JAMA Psychiatry. Published online October 12, 2016. doi:10.1001/jamapsychiatry.2016.2575 eAppendix. Methods eReferences. eFigure 1. Multidimensional scaling of RNA sequencing gene expression of hiPSC neurons eFigure 2. Box plots showing the distributions of normalized gene expression in schizophrenia vs controls eFigure 3. Quantitative real-time PCR (qRT-PCR) validation of DEGs for Disease status (schizophrenia vs control) eFigure 4. Binned density scatterplot eFigure 5. Pathway enrichment analysis for differential expressed genes between control and schizophrenia neurons across a group of gene sets for known molecular pathways and biological processes eFigure 6. Box plots showing the distributions of normalized gene expression in schizophrenia vs controls stratified by treatment with PBS and potassium chloride for SLC38A2 and THNSL1 genes eFigure 7. Binned density scatterplots comparing the log2 fold-change for PCID treatment eFigure 8. Pathway enrichment analysis for differential expressed genes eFigure 9. Quantitative real-time PCR (qRT-PCR) validation of DEGs for PCID alterations of gene expression eFigure 10. Percentage of variance explained by each experimental variable for the top 20 control signatures eFigure 11. Box plot showing the distributions of normalized gene expression in schizophrenia vs controls stratified by treatment with PBS and potassium chloride for KIF5B gene eFigure 12. Pathway enrichment analysis for the top 5% of control signatures across a group of gene sets for known molecular pathways and biological processes eFigure 13. Module eigengene patterns and enrichment scores of the top 5 enriched functional gene sets for modules that are significant at FDR ≤0.05 with diagnosis (brown and turquoise) or PCID (yellow and green) eFigure 14. Intramodular hub genes for modules that are significantly associated with disease and PCID eTable 1. Primers for real-time PCR © 2016 American Medical Association. All rights reserved. Downloaded From: https://jamanetwork.com/ on 09/30/2021 eTable 2. Demographic and sequencing data for the schizophrenia and control samples eTable 3. Differentially expressed genes for diagnosis (control vs schizophrenia) at FDR 5% eTable 4. Differentially expressed genes for PCID (untreated or treated with potassium chloride) at FDR 5% eTable 5. Differentially expressed genes for PCID in controls at FDR 5% eTable 6. Differentially expressed genes for PCID in schizophrenia at FDR 5% eTable 7. Summary of coexpression modules associated with diagnosis and PCID eTable 8. Summary of genetic enrichment with coexpression modules This supplementary material has been provided by the authors to give readers additional information about their work. © 2016 American Medical Association. All rights reserved. Downloaded From: https://jamanetwork.com/ on 09/30/2021 eAppendix Methods Generation of hiPSC neurons Neural progenitor cells (NPCs) were differentiated from hiPSCs as previously described1,2. We used hiPSCs from 4 cases with schizophrenia and 4 controls that have been reported in previous studies1,2. NPCs were maintained at high density, grown on Matrigel (BD)-coated plates in NPC media (DMEM/F12, 1x N2, 1x B27-RA (Invitrogen), 1 µg/ml Laminin and 20 ng/ml FGF2) and split approximately 1:4 every week with Accutase (Millipore) (for more information, see3). For neural differentiations, dissociated NPCs were plated in neural differentiation media (DMEM/F12, 1x N2, 1X B27-RA, 20 ng/ml BDNF (Peprotech), 20 ng/ml GDNF (Peprotech), 1 mM dibutyrl- cyclicAMP (Sigma), 200 nM ascorbic acid (Sigma) onto polyornithine/Laminin-coated plates at 200,000 cells per well of a 6-well plate. hiPSC derived-neurons were differentiated for 6 weeks; media was replaced every 5-7 days. Neurons were treated with 50mM KCl (or PBS vehicle control) for the final three hours prior to harvest. RNA preparation and sequencing Total RNA was isolated from approximately density-matched plates (initially seeded with ~200,000 NPCs per well) using TRIzol (Thermo Fisher), according to the manufacturer’s instructions. Briefly, RNA was chloroform extracted, pelleted with isopropanol, washed with 70% ethanol and resuspended in water. RNA was treated with RQ1 RNAse-free DNAse (Promega) for 30 minutes at 37C and then the reaction was inactivated by incubation with EGTA Stop buffer at 65C for 10 minutes. The RNA Integrity Number © 2016 American Medical Association. All rights reserved. Downloaded From: https://jamanetwork.com/ on 09/30/2021 (RIN) was determined using an RNA Nano chip (Agilent Technologies) on the Agilent 2100 Bioanalyzer. All samples have high RIN (mean ± standard deviation: 9.53 ± 0.22). 500 ng of total RNA was used as input material for library preparation using the TruSeq Stranded Total RNA Sample Prep Kit (LT) (Illumina, USA) according to manufacturer’s instructions. Individual libraries were indexed as recommended by Illumina. Mapping and Normalization of Gene Expression Mapping and quantification: We mapped reads to hg19 reference genome using TopHat (version 2.0.9) and Bowtie (version 2.1.0), with the default parameters. Known Ensembl gene levels (version 70) were quantified by HTSeq (version 0.6.0) in intersection-strict mode. A sample-by-gene read count matrix was generated for 16 samples by 56,632 Ensembl genes. Scaling normalization to remove composition biases in sequencing data was applied to log(CPM) (read Counts Per Million total reads) using the trimmed mean of M-values (TMM) method4. Covariates exploration: We selected covariates for inclusion to differential gene expression analysis by performing principal component analysis of the normalized read count matrix and examining which variables were significantly correlated with the high- variance components (explaining > 1% of the variance) of the data. This identified five covariates, which encompassed 5 statistical degrees of freedom (df): Diagnosis (control and schizophrenia), Treatment (PBS and KCl), Gender (Male and female), Age and RIN. © 2016 American Medical Association. All rights reserved. Downloaded From: https://jamanetwork.com/ on 09/30/2021 Normalized gene expression: We used the voomWithQualityWeights function from the limma package to model the normalized read counts. This function estimates weights by combining observational-level weights from voom with sample-specific weights estimated using the arrayWeights function. Briefly, in the first step voom estimates confidence weights for each normalized observed read count by residualizing on the covariates, fitting a mean-variance relationship function across all genes, using the fitted function to estimate the variance of a particular read count observation, and then setting the observation weight to be the inverse of the corresponding estimated variance. This is an important step to model the statistical sampling of gene expression level, since larger log(CPM) typically exhibit lower variance. In the second step, the estimated sample weights are used to obtain observational weights that take into account variations in sample quality. The variance model is then fitted a second time and a final set of modified weights is used in the linear modeling and differential expression analysis. Differential analysis: For each transcript, we fit weighted least-squares linear regression models for the effect on gene expression of each variable on the right-hand side: Gene expression ~ diagnosis + treatment + covariates Then, for each transcript, the diagnosis (control and schizophrenia) and treatment (PBS and KCl) coefficients were statistically tested for being non-vanishing, implying an estimated effect, above and beyond any other effect from the covariates (Gender, Age and RIN) on gene expression using linear regression utilities in the limma package. P- values were then adjusted for multiple hypotheses testing using false discovery rate © 2016 American Medical Association. All rights reserved. Downloaded From: https://jamanetwork.com/ on 09/30/2021 (FDR) estimation, and the differentially expressed genes were determined as those with an estimated FDR ≤0.05. Explore effect of 3-hour treatment of PBS or KCl on gene expression While our study is well controlled to detect differences between schizophrenia and controls and/or in response to depolarization, it is unclear if 3-hour exposure to either KCl or PBS changes gene expression, especially transcripts related to stress or starvation molecular processes. To explore this hypothesis, we leverage RNAseq data generated in the same samples without 3-hour exposure to 50mM KCl or PBS and explore for differences that exist in gene expression based on the following model: Gene expression ~ Cohort + Covariates Where cohort is Neurons with and without 3-hour exposure to 50mM KCl or PBS and covariates included Age, sex and RIN. We identified a set of 792 that are DEG among the 2 cohorts, but this is not surprising since the experiments were conducted independently at different time points (and were sequenced in different facilities) so that will include technical and experimental batch effect. We then used the 792 genes and performed pathway analysis to determine if pathways related to stress response are significantly enriched. The pathways that were enriched at FDR <20% are included below. Annotation Pval O FD ue R R go:CC|nucleosome 1.8E 13 2.5E -08 .0 -06 go:BP|nucleosome assembly 8.0E 6. 9.4E -07 4 -05 go:BP|cerebellar Purkinje cell layer development 5.9E 14 6.7E -05 .0 -03 go:MF|beta-catenin binding 1.6E 4. 1.7E -04 1 -02 © 2016 American Medical Association. All rights reserved. Downloaded From: https://jamanetwork.com/ on 09/30/2021 go:BP|positive regulation of DNA binding 4.0E 8. 3.9E -04 4 -02 go:BP|negative regulation of myoblast differentiation 4.4E 11 4.0E -04 .6 -02 go:BP|vocalization behavior 4.4E 11 4.0E -04 .6 -02 go:BP|negative regulation of transcription from RNA polymerase II 8.9E 1. 7.8E promoter -04 8 -02 go:BP|negative regulation of BMP signaling pathway 9.9E 5. 8.3E -04 4 -02 go:BP|regulation of alpha-amino-3-hydroxy-5-methyl-4-isoxazole 1.6E 7.
Details
-
File Typepdf
-
Upload Time-
-
Content LanguagesEnglish
-
Upload UserAnonymous/Not logged-in
-
File Pages155 Page
-
File Size-