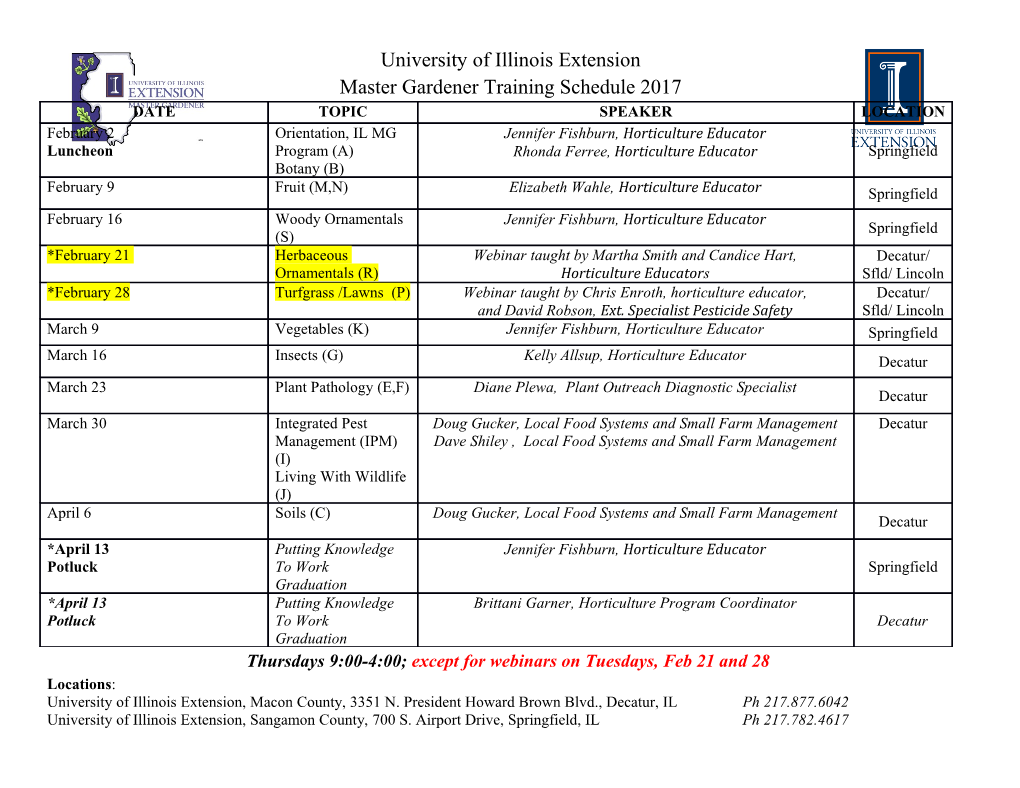
remote sensing Article Mapping Soil Organic Carbon for Airborne and Simulated EnMAP Imagery Using the LUCAS Soil Database and a Local PLSR Kathrin J. Ward 1,* , Sabine Chabrillat 1 , Maximilian Brell 1, Fabio Castaldi 2, Daniel Spengler 1 and Saskia Foerster 1 1 Helmholtz Center Potsdam, GFZ German Research Center for Geosciences, Section 1.4 Remote Sensing and Geoinformatics, Telegrafenberg A17, 14473 Potsdam, Germany; [email protected] (S.C.); [email protected] (M.B.); [email protected] (D.S.); [email protected] (S.F.) 2 Research Institute for Agriculture, Fisheries and Food—ILVO, Burgemeester Van Gansberghelaan 92 box 1, 9820 Merelbeke, Belgium; [email protected] * Correspondence: [email protected] Received: 27 August 2020; Accepted: 15 October 2020; Published: 20 October 2020 Abstract: Soil degradation is a major threat for European soils and therefore, the European Commission recommends intensifying research on soil monitoring to capture changes over time and space. Imaging spectroscopy is a promising technique to create spatially accurate topsoil maps based on hyperspectral remote sensing data. We tested the application of a local partial least squares regression (PLSR) to airborne HySpex and simulated satellite EnMAP (Environmental Mapping and Analysis Program) data acquired in north-eastern Germany to quantify the soil organic carbon (SOC) content. The approach consists of two steps: (i) the local PLSR uses the European LUCAS (land use/cover area frame statistical survey) Soil database to quantify the SOC content for soil samples from the study site in order to avoid the need for wet chemistry analyses, and subsequently (ii) a remote sensing model is calibrated based on the local PLSR SOC results and the corresponding image spectra. This two-step approach is compared to a traditional PLSR approach using measured SOC contents from local samples. The prediction accuracy is high for the laboratory model in the first step with R2 = 0.86 and RPD = 2.77. The HySpex airborne prediction accuracy of the traditional approach is high and slightly superior to the two-step approach (traditional: R2 = 0.78, RPD = 2.19; two-step: R2 = 0.67, RPD = 1.79). Applying the two-step approach to simulated EnMAP imagery leads to a lower but still reasonable prediction accuracy (traditional: R2 = 0.77, RPD = 2.15; two-step: R2 = 0.48, RPD = 1.41). The two-step models of both sensors were applied to all bare soils of the respective images to produce SOC maps. This local PLSR approach, based on large scale soil spectral libraries, demonstrates an alternative to SOC measurements from wet chemistry of local soil samples. It could allow for repeated inexpensive SOC mapping based on satellite remote sensing data as long as spectral measurements of a few local samples are available for model calibration. Keywords: soil organic carbon; mapping; hyperspectral; EnMAP; LUCAS; localPLSR 1. Introduction Soil degradation is a serious concern worldwide with implications for climate change and food security [1]. Soil organic carbon (SOC), especially, is an important soil property as its presence is a precondition for the formation of soils and it plays an essential role in soil fertility and soil water characteristics [2,3]. Soils are one of the largest carbon sinks and hence variations in SOC content have effects on the climate [1]. In the framework of the Sustainable Development Goals (SDGs) listed Remote Sens. 2020, 12, 3451; doi:10.3390/rs12203451 www.mdpi.com/journal/remotesensing Remote Sens. 2020, 12, 3451 2 of 20 by the United Nations, SOC is regarded as the most relevant soil property with regard to climate regulations [2] and as such is listed as one of the GCOS (Global Climate Observing System) ECV (Essential Climate Variables) for which monitoring of the SOC status should be improved [4]. In order to prevent further soil degradation, there is a recognized need for up-to-date, spatially accurate and inexpensive soil maps to monitor soils and their processes (e.g., [5–7]) As traditional soil mapping involves intense soil sampling and wet chemistry analyses, it is time and cost intensive and the spatial accuracy is dependent on the density of collected soil samples. An alternative to wet chemistry is soil spectroscopy which uses spectral information to derive key soil properties. It is a well-established method under laboratory conditions using point spectrometers [8] which measure the radiation reflected by an object at a single point. Therefore, soil properties need to be spectrally active to produce a specific spectral signature or alternatively they need to be correlated to a soil property that fulfils this requirement (e.g., [5]). SOC shows strong interactions with the electromagnetic radiation in the spectral region visible for human eyes and hence, soils rich in SOC appear darker as a general decrease in reflectance is observed with an increasing SOC. Furthermore, biochemical components of soil organic matter like chlorophyll, cellulose, starch, oil, pectin, lignin and humic acids [9] have impacts on the reflectance in from the visible (400–700 nm) to the near-/shortwave infrared (700–2500 nm) regions [10] of the electromagnetic spectrum. As a first effect, a general flattening of the reflectance spectral curve in the visible (VIS) is typical of SOC in agricultural soils, related to the decomposition of chlorophyll pigments which originally causes a wide spectral absorption feature around 664 nm [10,11]. Furthermore, there are strong and narrow absorptions caused by SOC in the short-wave infrared (SWIR) between 2100–2300 nm that can be observed in lignite-rich soils [10–12]. To handle the broad, weak and superimposing absorption features of soils, most studies use partial least squares regression (PLSR) or similar multivariate modelling techniques as they cope well with a high collinearity and a large number of predictor variables [13,14]. Additionally, imaging spectroscopy, primarily from airborne platforms, is already widely used in many local studies and leads to good prediction accuracies, with overviews provided in: [5,15]. Many studies have been conducted to estimate the SOC content mainly at a local farm or field scale using hyperspectral imagery and soil samples collected at the study site, which were later analyzed for their SOC content at a laboratory [13]. Using airborne hyperspectral data, good prediction accuracy can be achieved: [16] used the airborne HyMAP sensor to estimate the SOC content in northern Germany using PLSR at field scale and received an R2 = 0.9. Lower prediction accuracies were reported using the first generation of spaceborne hyperspectral imagers: [17] explain their SOC prediction accuracy using Hyperion data and PLSR models of R2 = 0.51 and RPD = 1.43 in Australia by a low signal-to-noise ratio (SNR) and a spatial resolution of 30 m. When moving from the laboratory to an airborne and spaceborne scale, new challenges arise for soil property quantification, e.g., weather conditions and atmospheric attenuation, soil conditions (mainly moisture content, roughness and crusts), internal pixel variations of the soil property or even fractions of other land cover types within the pixel [5,18]. There is ongoing research to meet these challenges and improve model accuracy (overview in [5]). Scale is also an important component influencing model accuracy. On larger scales, soils are more inhomogeneous leading to increasing variances of soil properties but also to non-linear relationships between soil property and spectrum which complicates model calibration [14,19,20]. Furthermore, the availability of local or regional soil databases for model calibration and validation is an issue. A reduced need for soil samples for model calibration would be desirable, as sampling campaigns and wet chemistry analyses are time and cost intensive, especially with regard to applications at a global/regional scale. With the recent and upcoming availability of hyperspectral satellite data with high signal to noise ratio (e.g., PRISMA (PRecursore IperSpettrale della Missione Applicativa) [21], EnMAP (Environmental Mapping and Analysis Program) [22]) research also focusses on the potential for the quantification of soil properties from these new platforms and for larger areas. Chabrillat et al. [23] demonstrated that no simple best-practice rule exists concerning the method to be used for certain soil properties and sensors. Remote Sens. 2020, 12, 3451 3 of 20 For each combination the best method needs to be defined by further studies. Steinberg et al. [24] obtained good PLSR prediction accuracies for selected soil properties using simulated EnMAP images for sites in Spain and Luxembourg. Generally, the prediction accuracy decreases from airborne to simulated satellite observations with decreasing spatial resolution [23,24]. Currently, efforts are being made to create large scale soil spectral libraries that can be used for model calibration to develop more robust models that are applicable for larger areas in order to create global maps with less need for local soil sampling and analyses [5]. Wetterlind et al. [25] found that using the national soil spectral library of Sweden spiked with local samples from their Swedish study site, can lead to comparable results as if PLSR models were calibrated at a farm-scale. Castaldi et al. [26] used the samples of pan-European topsoil database LUCAS (land use/cover area frame survey) located within a selected scene of the multispectral Sentinel-2 mission to fit exponential regression models based on band combinations. When applied to the Sentinel-2 bands, they received good validation results by using the red and red edge bands. Castaldi et al. [27] obtained good results by also using LUCAS in a bottom-up approach using PLSR to predict SOC for hyperspectral airborne data acquired in Belgium and Luxembourg without a spectral transfer function between laboratory and airborne data. Tziolas et al. [28] estimated the SOC content for a study site in Israel applying a spiked bottom-up approach with a local Gaussian regression on airborne imagery.
Details
-
File Typepdf
-
Upload Time-
-
Content LanguagesEnglish
-
Upload UserAnonymous/Not logged-in
-
File Pages20 Page
-
File Size-