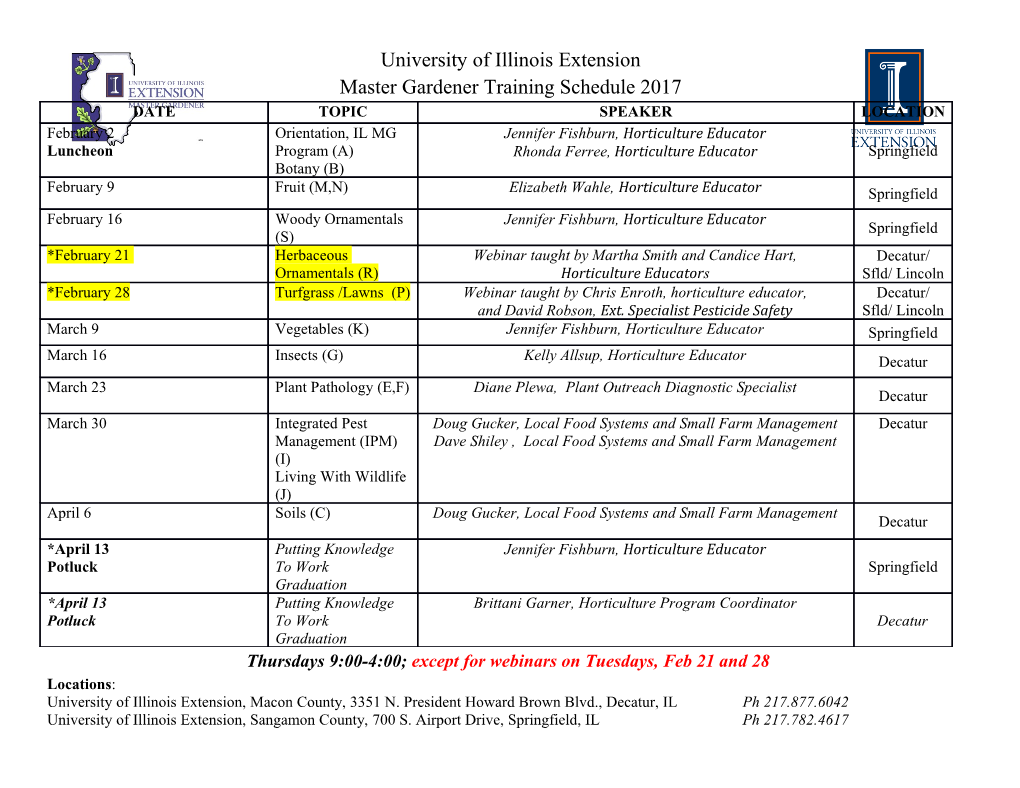
bioRxiv preprint doi: https://doi.org/10.1101/2020.09.11.293258; this version posted September 11, 2020. The copyright holder for this preprint (which was not certified by peer review) is the author/funder, who has granted bioRxiv a license to display the preprint in perpetuity. It is made available under aCC-BY-ND 4.0 International license. 1 1 2 A comparative survey of betacoronavirus strain molecular dynamics 3 identifies key ACE2 binding sites 4 5 P. X. Rynkiewicz1, G. A. Babbitt1*, F. Cui1, A. O. Hudson1, M. L. Lynch2 6 7 8 9 10 11 Running title – coronavirus binding molecular dynamics 12 13 14 15 16 17 18 bioRxiv preprint doi: https://doi.org/10.1101/2020.09.11.293258; this version posted September 11, 2020. The copyright holder for this preprint (which was not certified by peer review) is the author/funder, who has granted bioRxiv a license to display the preprint in perpetuity. It is made available under aCC-BY-ND 4.0 International license. 2 1 ABSTRACT 2 Comparative functional analysis of the binding interactions between various betacoronavirus 3 strains and their potential human target proteins, such as ACE1, ACE2 and CD26, is critical to 4 our future understanding and combating of COVID-19. Here, employing large replicate sets of 5 GPU accelerated molecular dynamics simulations, we statistically compare atom fluctuations of 6 the known human target proteins in both the presence and absence of different strains of the 7 viral receptor binding domain (RBD) of the S spike glycoprotein. We identify a common 8 interaction site between the N-terminal helices of ACE2 and the viral RBD in all strains (hCoV- 9 OC43, hCoV-HKU1, MERS-CoV, SARS-CoV1, and SARS-CoV-2) and a second more dynamically 10 complex RBD interaction site involving the ACE2 amino acid sites K353, Q325, and a novel 11 motif, AAQPFLL (386-392) in the more recent cross-species spillovers (i.e. absent in hCoV- 12 OC43). We use computational mutagenesis to further confirm the functional relevance of these 13 sites. We propose a “one touch/two touch” model of viral evolution potentially involved in 14 functionally facilitating binding interactions in zoonotic spillovers. We also observe these two 15 touch sites governing RBD binding activity in simulations on hybrid models of the suspected 16 viral progenitor, batCoV-HKU4, interacting with both the human SARS target, ACE2, and the 17 human MERS target, CD26. Lastly, we confirm that the presence of a common hypertension 18 drug (lisinopril) within the target site of SARS-CoV-2 bound models of ACE1 and ACE2 acts to 19 enhance the RBD interactions at the same key sites in our proposed model. In the near future, 20 we recommend that our comparative computational analysis identifying these key viral RBD- 21 ACE2 binding interactions be supplemented with comparative studies of site-directed 22 mutagenesis in order to screen for current and future coronavirus strains at high risk of 23 zoonotic transmission to humans. 24 KEYWORDS Molecular dynamics, molecular evolution, viral binding, COVID 19 25 26 STATEMENT OF SIGNIFICANCE 27 We generated structural models of the spike glycoprotein receptor binding domain from recent 28 and past betacoronavirus outbreak strains aligned to the angiotensin 1 converting enzyme 2 29 protein, the primary target protein of the SARS-CoV-2 virus causing COVID 19. We then 30 statistically compared computer simulated molecular dynamics of viral bound and unbound 31 versions of each model to identify locations where interactions with each viral strain have 32 dampened the atom fluctuations during viral binding. We demonstrate that all known strains 33 of betacoronavirus are strongly interactive with the N-terminal helix region of ACE2. We also 34 identify a more complex viral interaction with three novel sites that associates with more 35 recent and deadly SARS strains, and also a bat progenitor strain HKU4. 36 bioRxiv preprint doi: https://doi.org/10.1101/2020.09.11.293258; this version posted September 11, 2020. The copyright holder for this preprint (which was not certified by peer review) is the author/funder, who has granted bioRxiv a license to display the preprint in perpetuity. It is made available under aCC-BY-ND 4.0 International license. 3 1 INTRODUCTION 2 The SARS-CoV-2 virus belongs to the class of positive-strand RNA betacoronaviruses 3 structurally defined by a nucleocapsid interior surrounded by the spike, membrane, and 4 envelope proteins (1). Structural membrane and envelope proteins are known to play a key role 5 in virion assembly and budding from infected cells, making them potential targets for antiviral 6 treatments (2). Additionally, the unprecedented infectivity of SARS-CoV-2 brings into question 7 the structural, functional, and evolutionary similarities between it and other coronavirus 8 outbreaks, including those that have evolved into less pathologically severe strains. One 9 prominent candidate for comparative studies of the propensity for viral transmission is the 10 spike protein, which is responsible for initial viral contact with the target host receptor and 11 subsequent entry into host cells. 12 The spike protein consists of two homotrimeric functional subunits: the S1 subunit 13 consisting of the Receptor Binding Domains (RBDs) interacting directly with the host, and the S2 14 subunit which directly fuses the virion to the host cellular membrane (3). The S1 and S2 15 subunits of coronaviruses are cleaved and subsequently activated by type-II transmembrane 16 serine proteases and the subtilisin-like serine protease furin, the latter of which evidently 17 primes SARS-CoV-2 for cellular entry but not SARS-CoV-1 (4, 5). The boundary between the S1 18 and S2 subunits forms an exposed loop in human coronaviruses SARS-CoV-2,OC43, HKU1, and 19 MERS-CoV that is not found in SARS-CoV-1 (6). This suggests a complex evolutionary landscape 20 for SARS-CoV-related coronaviruses, the breadth of which cannot be fully understood through 21 amino acid or nucleotide sequence comparisons alone. 22 The spike protein of SARS-CoV-2 has been demonstrated to directly and specifically 23 target the human angiotensin converting enzyme 2 (ACE2) receptor, which has a role in the 24 renin–angiotensin system (RAS) critical for regulating cardiovascular and renal functions (7,8). 25 ACE2 is a glycoprotein consisting of 805 amino acid residues that is the primary target for 26 docking and viral entry in many coronavirus infections, including those belonging to SARS-CoV 27 and human alpha-coronavirus NL63 (9). ACE2 is classified as a zinc metallopeptidase 28 (carboxypeptidase) involved in cleaving a single amino acid residue from angiotensin I (AngI) to 29 generate Ang1-9 (10). In addition, ACE2 also cleaves a single amino acid residue from 30 angiotensin II (Ang II) to generate Ang1-7 (10). ACE2 receptors reside on the surfaces of 31 multiple epithelial cells, with tissue distribution predominantly in the lung and gut linings, but 32 are also found in heart, kidney, liver and blood vessel tissues. Given the dominant role ACE2 33 plays in SARS-CoV-2 viral entry, understanding how sequence and structural variations affect 34 the molecular dynamics of ACE2/RBD interactions can provide information to guide the 35 rationale design and development of therapeutics targeting this interaction. The comparative 36 dynamic modeling of present, past, and potential progenitor viral strain interactions to ACE2 37 and other known targets of past outbreaks, such as CD26, the target of MERS-CoV, can also bioRxiv preprint doi: https://doi.org/10.1101/2020.09.11.293258; this version posted September 11, 2020. The copyright holder for this preprint (which was not certified by peer review) is the author/funder, who has granted bioRxiv a license to display the preprint in perpetuity. It is made available under aCC-BY-ND 4.0 International license. 4 1 potentially illuminate critical functional aspects that facilitate zoonotic spillovers involving the 2 betacoronaviruses. 3 A powerful tool for in silico analysis of protein-protein interactions is all-atom all-atom 4 accelerated molecular dynamics (aMD) simulation, which attempts to accurately sample the 5 physical trajectories of molecular structures over time via traditional Newtonian mechanics 6 played out over a modified potential energy landscape (11). Protein structures determined via 7 x-ray crystallography have previously been used to provide insights into viral evolution by 8 comparing angstrom scale distances and conformational similarities. MD simulations are 9 increasingly broadening these methods of inquiry by allowing further analysis of shorter time 10 scale dynamics of protein-protein interactions (12). In the SARS-CoV-2 pathosystem, MD 11 simulations have already been employed to map the hydrogen bonding topology of the SARS- 12 CoV-2 RBD/ACE2 interaction, explore the merits of drug repurposing, characterize mutations, 13 and determine the stability of binding interactions between the SARS-CoV-2 RBD and several 14 contemporary antiviral drugs (13, 14, 15, 16). 15 While conventional and accelerated MD simulations can provide unprecedented 16 resolution on protein motion over time, interpretations based upon comparisons of single MD 17 trajectories are statistically problematic and difficult to compare. This makes the application of 18 MD to comparative questions regarding functional binding or impacts of mutation challenging. 19 Given recent increases in GPU processor power, statistically comparing large replicates of aMD 20 simulations is now an available solution to this problem. Because MD trajectories of complex 21 structures like proteins are inherently complex and chaotic, even if they are adequately 22 resampled, they do not often fit traditional model assumptions of many parametric statistical 23 tests. So reliable comparisons of dynamics need to further be based upon statistical methods 24 that can fully capture the multi-modal distributional space of protein motion and assign 25 probability scores in the absence of any distributional model that would attempt to describe 26 protein motion.
Details
-
File Typepdf
-
Upload Time-
-
Content LanguagesEnglish
-
Upload UserAnonymous/Not logged-in
-
File Pages27 Page
-
File Size-