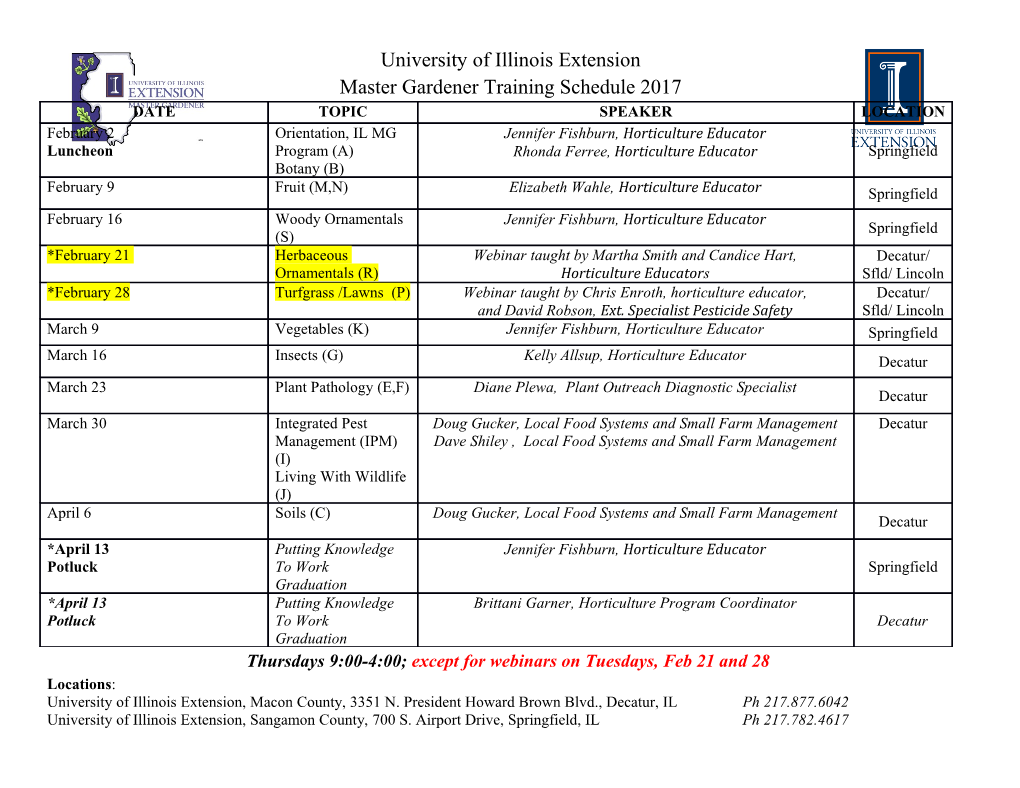
Gene Networks Hans J. Bohnert Shisong Ma (present address: Yale University) Introduction High-throughput transcript profiling platforms ± microarrays in a variety of forms - make use of the increasing amount of genomic DNA and EST sequences and generate a flood of data. Tools are urgently needed that allow for analyses that convert these data into models for the structures of any underlying pathways and causal networks (Brazhnik et al., 2002). From an organism's response to various developmental or externally manipulated conditions at the transcript level it should be possible to infer functional relations between genes based on co- expression pattern, essentially by assuming ªguilt by associationº. However, the use of these data in interpreting how genes respond to external or internal, i.e., hormonal or biochemical, stimuli has been fraught with uncertainties. Statistical models abound, yet there is no consensus about how biological context and significance are to be merged in these models. Common are notions that generate ªrelevance networksº by determining Pearson correlation coefficients (Markowetz and Spang, 2007), which indeed associate many genes with similar expression patterns. However, the method is encumbered by several problems because it cannot separate indirect from direct interactions and tends to recover far too many interactions, which then frustrate interpretation (Schäfer and Strimmer, 2005a). Also, results are dominated by a few heavily connected 1 pathways, for example genes associated with functions of the ribosome, while the effects of environmental factors are less faithfully represented. We have chosen the graphical Gaussian model, GGM, as a method for representing correlation. GGM is based on partial correlation (Schäfer and Strimmer, 2005a, b; Opgen-Rhein and Strimmer, 2007), placing the relationship between gene pairs in the context of the entire transcriptome, while the Pearson correlation approach considers each pair of genes separate. The partial correlation approach has superior ability in separating direct from indirect interactions. However, GGM is impeded by what may be called the problem of ªlarge p, small nº. This describes the fact that the number of microarray slides (n) in a dataset is invariably much smaller than the number of genes (p) in a genome. For the model plant Arabidopsis thaliana, whose genome is well known, some 3,000 microarray experiments with the Affymetrix ATH1 platform on which >22,000 gene probes are printed represent ~150 experimental conditions. A ªshrinkageº method (Schäfer and Strimmer, 2005a, b) makes it possible to infer partial correlations from among 2,000 genes from this dataset. We then proposed and implemented an iterative sampling routine, coupled with the shrinkage approach, thus expanding the partial correlation analysis to establish network coverage of the whole genome (Ma et al., 2007). Subsequent analyses revealed that GGM recovers interactions between seemingly unrelated biological pathways (developmental and biochemical) that cover diverse aspects, which provided particular advantages for the analysis of responses to external factors. Depending on the stringency of the settings, which may be adjusted based on biological knowledge, it is possible to recover 2 genome-wide networks of moderate sizes that cover many pathways, and which can be controlled by the degree with which they recount known gene interactions. Although this represents a heuristic, experimental approach many interactions with biological significance have been recovered (Ma et al., 2007; Ma and Bohnert, 2008; Li et al., 2008). Further meaningful enhancement of the GGM can be obtained using clustering methods (Gasch and Eisen, 2002; Ma et al., 2006; Ma and Bohnert, 2007). It seems that modified GGM approaches can provide novel understanding of transcript profiles, that the process can identify genes in biochemical pathways, in explaining hormonal, biotic and abiotic stress-relevant responses, and in placing genes into developmental pathways. The chosen model is particularly suitable placing various isoforms of genes in families into different context, and by assigning genes of unknown functions into networks that can then be experimentally interrogated. GGM would profit from increased experimentation, especially if the number of experimental conditions and the number of time course experiments were increased, and if more wild type to (knockout) mutant comparisons were included. The numbers of stringently controlled and annotated experiments is not yet sufficient to provide a sufficiently complex and nearly scale-free model of the Arabidopsis transcriptome. Also, we are still far from being able to integrate into an improved GGM and to see in context data established by different microarray platforms, or to profit from diverse datasets as they are provided by protein interaction or metabolite dynamics studies, via, e.g., Bayesian network approaches (Lee et al, 2004b), although robust models about 3 how to reconcile data from different microarray platforms are still to be in developed. Another plant database exists that organizes a network based on the Pearson correlation coefficient (Obayashi et al, 2007). For non-plant organisms, a co- expression network based on 1st order partial correlation exists for yeast (Magwene and Kim, 2004). Other networks, for yeast, C. elegans, or human tissues, typically combine co-expression data with other types of data, such as protein-protein interactions, CHIP-experimental data or across-species gene conservation studies (Ramani et al., 2008; Lee et al., 2008; Lee et al., 2004a; Lee et al., 2007). Owing to the fact that more protein interaction studies have been conducted for important other models, human cell cultures or yeast in particular, less emphasis has been placed in these models on gene networks, while integration of different datasets has been emphasized. A similar trajectory can be expected for plant datasets in the future to become incorporated into the TAIR database that strives o incorporate all information on Arabidopsis genetics, biochemistry and genomics (Rhee et al., 2003). References Brazhnik, P., A. de la Fuente, and P. Mendes. (2002) Gene networks: how to put the function in genomics. Trends Biotechnol 20: 467-472. Gasch, A.P., Eisen, M.B. (2002) Exploring the conditional co-regulation of yeast gene expression through fuzzy k-means clustering. Genome Biol. 3: R 0059. 4 Lee, I., Date, S.V., Adai, A.T., and Marcotte, E.M. (2004a) A probabilistic functional network of yeast genes. Science 306:155-1558. Lee, H.K., Hsu, A.K., Sajdak, J., Qin, J., Pavlidis, P. (2004b) Coexpression analysis of human genes across many microarray data sets. Genome Res. 14: 1085-1094. Lee, I., Li, Z, Marcotte, E.M. (2007) An Improved, Bias-Reduced Probabilistic Functional Gene Network of Baker©s Yeast, Saccharomyces cerevisiae. PLoS ONE 2: e988 Lee, I., Lehner, B., Crombie, C., Wong, W., Fraser, A.G., Marcotte, E.M. (2008) A single gene network accurately predicts phenotypic effects of gene perturbation in Caenorhabditis elegans. Nat Genet. 40:181-8 Lee, T.I., Rinaldi, N.J., Robert, Odom, R.D.T., Bar-Joseph, Z., Gerber, G.K., Hannett, N.M., Harbison, C.T., Thompson, C.M., Simon, I., Zeitlinger, J., Jennings, E.G., Murray, H.L., Gordon, D.B., Ren, B., Wyrick, J.J., Tagne, J.B., Volkert, T.L., Fraenkel, E., Gifford, D.K., Young, R.A. (2002) Transcriptional regulatory networks in Saccharomyces cerevisiae. Science 298: 799-804. Li, P., Ma, S., Bohnert, H.J. (2008) Co-expression characteristics of trehalose 6- phosphate phosphatase sub-family genes reveal different functions in a network context. Physiol. Plant. in press. Ma, S., Gong, Q., Bohnert, H.J. (2006) Dissecting Salt Stress Pathways. J. Exp. Bot. 57: 1097-1107. 5 Ma, S., Bohnert, H.J. (2007) Integration of Arabidopsis thaliana stress-related transcript profiles, promoter structures, and cell-specific expression. Genome Biol. 8: R49. Ma, S., Gong, Q., Bohnert, H.J. (2007) An Arabidopsis Gene Network based on the Graphical Gaussian Model. Genome Res. 17: 1614-1625. Ma, S., Bohnert, H.J. (2008) Genomics Data, Integration, Networks and Systems. Molec. BioSystems, epub: January 9, 2008. Magwene, P.M., Kim, J. (2004) Estimating genomic coexpression networks using first-order conditional independence. Genome Biol 5: R100. Markowetz, F., Spang, R. (2007) Inferring cellular networks - a review. BMC Bioinformatics 8 Suppl6: S5 Obayashi, T., Kinoshita, K., Nakai, K., Shibaoka, M., Hayashi, S., Saeki, M., Shibata, D., Saito, K., Ohta, H. (2007) ATTED-II: a database of co-expressed genes and cis elements for identifying co-regulated gene groups in Arabidopsis. Nucleic Acids Res. 35: D863±D869. Opgen-Rhein , R., Strimmer, K. (2007) From correlation to causation networks: a simple approximate learning algorithm and its application to high-dimensional plant gene expression data. BMC Syst. Biol. 1: 37. Ramani, A.K., Li, Z., Hart, G.T., Carlson, M.W., Boutz, D.R., Marcotte, E.M. (2008) A map of human protein interactions derived from co-expression of human mRNAs and their orthologs. Mol Syst Biol 4:180 Rhee, S.Y., Beavis, W., Berardini, T.Z., Chen, G., Dixon, D., Doyle, A., Garcia- Hernandez, M., Huala, E., Lander, G., Montoya, M. et al. (2003) The 6 Arabidopsis Information Resource (TAIR): a model organism database providing a centralized, curated gateway to Arabidopsis biology, research materials and community. Nucleic Acids Res. 31: 224-228. Schäfer , J., Strimmer, K. (2005a) An empirical Bayes approach to inferring
Details
-
File Typepdf
-
Upload Time-
-
Content LanguagesEnglish
-
Upload UserAnonymous/Not logged-in
-
File Pages7 Page
-
File Size-