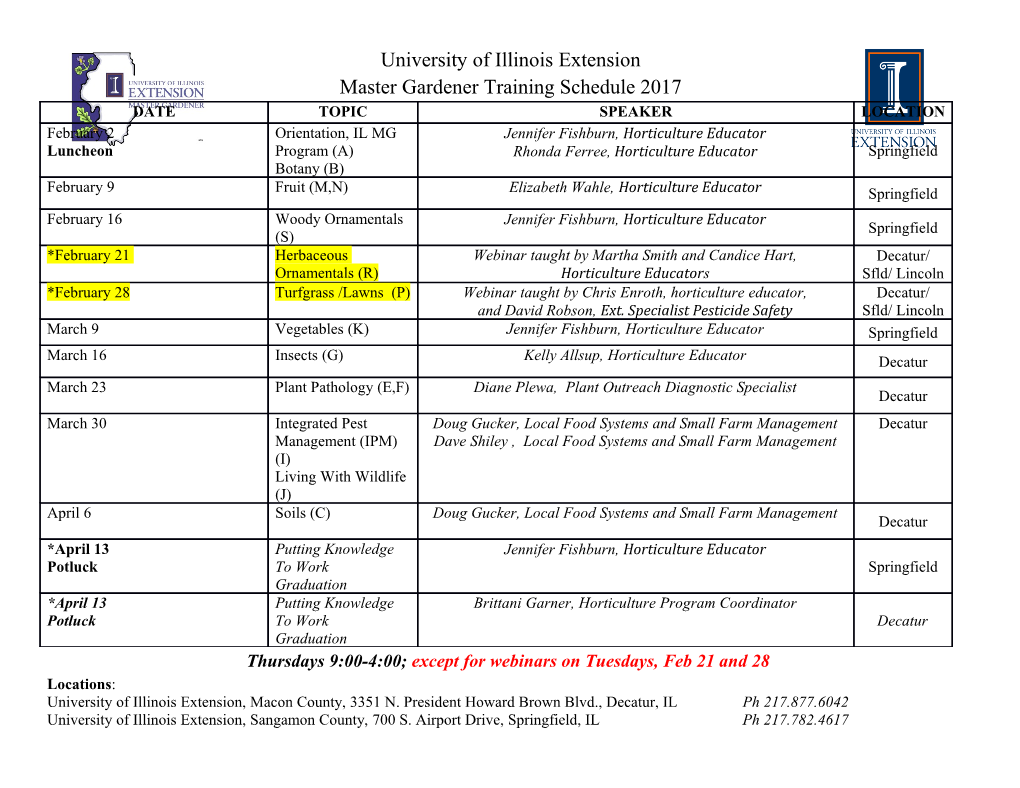
Purdue University Purdue e-Pubs Open Access Dissertations Theses and Dissertations January 2016 Cell Type-specific Analysis of Human Interactome and Transcriptome Shahin Mohammadi Purdue University Follow this and additional works at: https://docs.lib.purdue.edu/open_access_dissertations Recommended Citation Mohammadi, Shahin, "Cell Type-specific Analysis of Human Interactome and Transcriptome" (2016). Open Access Dissertations. 1371. https://docs.lib.purdue.edu/open_access_dissertations/1371 This document has been made available through Purdue e-Pubs, a service of the Purdue University Libraries. Please contact [email protected] for additional information. Graduate School Form 30 Updated 12/26/2015 PURDUE UNIVERSITY GRADUATE SCHOOL Thesis/Dissertation Acceptance This is to certify that the thesis/dissertation prepared By Shahin Mohammadi Entitled CELL TYPE-SPECIFIC ANALYSIS OF HUMAN INTERACTOME AND TRANSCRIPTOME For the degree of Doctor of Philosophy Is approved by the final examining committee: Ananth Grama Wojciech Szpankowski Chair David Gleich Jennifer Neville Markus Lill To the best of my knowledge and as understood by the student in the Thesis/Dissertation Agreement, Publication Delay, and Certification Disclaimer (Graduate School Form 32), this thesis/dissertation adheres to the provisions of Purdue University’s “Policy of Integrity in Research” and the use of copyright material. Approved by Major Professor(s): Ananth Grama William Gorman, Assistant to the Department Head 11/1/2016 Approved by: Head of the Departmental Graduate Program Date CELL TYPE-SPECIFIC ANALYSIS OF HUMAN INTERACTOME AND TRANSCRIPTOME A Dissertation Submitted to the Faculty of Purdue University by Shahin Mohammadi In Partial Fulfillment of the Requirements for the Degree of Doctor of Philosophy December 2016 Purdue University West Lafayette, Indiana ii I dedicate this thesis to my mom, whose role in my life I can not even begin to describe. She made my past, present, and future possible. There is no word that can express the sacrifices she made in her life to make sure I will have the best life I can. I am, and will always be, in debt to her. iii ACKNOWLEDGMENTS This journey would have not been possible if not for the great help from my major advisor, Ananth Grama, who not only mentored me, but also believed in me, trusted me, and encouraged me to explore my interests and curiosity. I am also grateful to my co-advisor, Wojciech Szpankowski, who continuously supported me and provided valuable guidance throughout my PhD. During these years, I had the honor and the pleasure of collaborating with great colleagues and made wonderful connections which contributed greatly to my success, my growth, and my achievements. I would like to give special thanks to Shankar Subramaniam who have provided great support throughout a few of our works, all of which resulted in wonderful contributions. I am also in debt to David Gleich who have always been there when I need someone to help me understand the problem, formulate it, or otherwise provide invaluable help. Working with him has been a pleasure and I am hoping to continue this relationship throughout my academic career. Last but not least I would like to give special thanks to Andrea Goldsmith and her postdoc Neta Zuckerman who have helped me form a big part of my current direction of research and provided insight on our joint work. I would also like to thank the members of my thesis committee, Markus Lill and Jennifer Neville, for reading this dissertation and providing helpful comments and feedback. iv TABLE OF CONTENTS Page LIST OF TABLES :::::::::::::::::::::::::::::::: viii LIST OF FIGURES ::::::::::::::::::::::::::::::: ix ABSTRACT ::::::::::::::::::::::::::::::::::: xii 1 INTRODUCTION :::::::::::::::::::::::::::::: 1 2 BIOLOGICAL BACKGROUND ::::::::::::::::::::::: 5 2.1 Genes, RNAs, Proteins { And How We Measure Them in Bulk Tissues 5 2.2 From Bulk Tissue Measurements to Single Cell RNA-Seq (scRNA-Seq) 6 2.3 Cell Type Specificity { Ubiquitously Expressed Versus Highly Selective Genes :::::::::::::::::::::::::::::::::: 7 2.4 Adding Cell Type-specificity to Biological Networks ::::::::: 8 3 A BIOLOGICALLY-INSPIRED KERNEL TO MEASURE SIMILARITY OF CELLS :::::::::::::::::::::::::::::::::: 9 3.1 Background ::::::::::::::::::::::::::::::: 9 3.2 Materials and Methods ::::::::::::::::::::::::: 11 3.2.1 Datasets ::::::::::::::::::::::::::::: 11 3.2.2 Identifying the Shared Subspace among a Group of Tissues : 12 3.2.3 Adjusting Transcriptional Signatures to Control for the Effect of Shared Subspace ::::::::::::::::::::::: 15 3.2.4 Computing Signal-to-Noise Ratio (SNR) ::::::::::: 16 3.2.5 Assessing the Significance of Marker Detection Methods :: 17 3.2.6 Combining Individual p-values to Compute a Meta p-value : 18 3.3 Results and Discussion ::::::::::::::::::::::::: 18 3.3.1 Adjusting for the Effect of Housekeeping Genes Enhances Signal- to-Noise Ratio (SNR) for the Known Marker Genes ::::: 18 3.3.2 Iterative Application of Adjustment Process Identifies Markers That are Comparable or Better Than the t-test ::::::: 19 3.3.3 Adjusted Cell Type Signatures Identify Groups of Similar Tis- sues/Cell Types ::::::::::::::::::::::::: 22 3.3.4 Putting the Pieces Together: Automated Identification of Cell Types and Their Characteristic Markers ::::::::::: 25 3.3.5 Adjusted Signatures Predict Tissue-specific Transcriptional Reg- ulatory Networks :::::::::::::::::::::::: 26 v Page 4 DE NOVO IDENTIFICATION OF CELL TYPES FROM SINGLE-CELL TRANSCRIPTOME ::::::::::::::::::::::::::::: 32 4.1 Background ::::::::::::::::::::::::::::::: 32 4.2 Materials and Methods ::::::::::::::::::::::::: 34 4.2.1 Datasets ::::::::::::::::::::::::::::: 34 4.2.2 Overview of Prior Methods for Cell-type Identification ::: 35 4.2.3 Overview and Justification for ACTION 's Components ::: 36 4.2.4 Step 1: A Biologically-inspired Metric for Similarity of Cells 37 4.2.5 Steps 2 and 3: A Geometric Approach to Identify Principal Functions (Representing Pure Cell Types) :::::::::: 39 4.2.6 Estimating the Total Number of Archetypes Needed to Repre- sent All Cell Types ::::::::::::::::::::::: 42 4.2.7 Steps 4 and 5: Constructing the Transcriptional Regulatory Network Corresponding to Each Archetype :::::::::: 44 4.3 Results and Discussion ::::::::::::::::::::::::: 46 4.3.1 Component 1: Measuring Cell-to-cell Similarity ::::::: 47 4.3.2 Component 2: A Geometric View to Identify Discrete Cell Types 50 4.3.3 Component 3: Constructing Subclass-specific Transcription Reg- ulatory Network of MITF-associated Patients :::::::: 53 5 SEPARATING CELL TYPES AND THEIR RELATIVE PERCENTAGES FROM COMPLEX TISSUES :::::::::::::::::::::::: 57 5.1 Background ::::::::::::::::::::::::::::::: 57 5.2 Materials and Methods ::::::::::::::::::::::::: 60 5.2.1 Datasets ::::::::::::::::::::::::::::: 60 5.2.2 Deconvolution: Formal Definition ::::::::::::::: 61 5.2.3 Overview of Prior In Silico Deconvolution Methods ::::: 73 5.2.4 Evaluation Measures :::::::::::::::::::::: 76 5.2.5 Implementation ::::::::::::::::::::::::: 77 5.3 Results and Discussion ::::::::::::::::::::::::: 78 5.3.1 Effect of Loss Function and Constraint Enforcement on Decon- volution Performance :::::::::::::::::::::: 78 5.3.2 Agreement of Gene Expressions With Sum-to-One (STO) Con- straint :::::::::::::::::::::::::::::: 83 5.3.3 Range Filtering { Finding an Optimal Threshold :::::: 86 5.3.4 Selection of Marker Genes { The Good, Bad, and Ugly ::: 95 5.3.5 To Regularize or Not to Regularize :::::::::::::: 100 5.3.6 Putting it All Together ::::::::::::::::::::: 101 5.3.7 Summary :::::::::::::::::::::::::::: 104 6 CONSTRUCTING CELL TYPE SPECIFIC INTERACTOMES ::::: 107 6.1 Background ::::::::::::::::::::::::::::::: 107 6.2 Materials and Methods ::::::::::::::::::::::::: 109 vi Page 6.2.1 Datasets ::::::::::::::::::::::::::::: 109 6.2.2 Constructing Human Tissue-specific Interactome ::::::: 111 6.2.3 Implementation Details ::::::::::::::::::::: 114 6.3 Results and Discussion ::::::::::::::::::::::::: 114 6.3.1 Transcriptional Activity Scores Predict Tissue-specificity of Genes 114 6.3.2 Constructing Tissue-specific Interactomes ::::::::::: 116 6.3.3 Qualitative Characterization of Tissue-specific Interactomes 117 6.3.4 Tissue-specific Interactome Predicts Context-sensitive Interac- tions in Known Functional Pathways ::::::::::::: 118 6.3.5 Tissue-specific Interactions are Enriched among Proteins with Shared Tissue-specific Annotations :::::::::::::: 120 6.3.6 Tissue-specific Interactions Densely Connect Genes Correspond- ing to Tissue-specific Disorders :::::::::::::::: 120 6.3.7 Tissue-specific Interactome Identifies Novel Disease-related Path- ways { Case Study in Neurodegenerative Disorders ::::: 122 7 CONSERVATION OF CELL TYPE-SPECIFIC NETWORKS ACROSS SPECIES :::::::::::::::::::::::::::::: 132 7.1 Background ::::::::::::::::::::::::::::::: 132 7.2 Materials and Methods ::::::::::::::::::::::::: 136 7.2.1 Datasets ::::::::::::::::::::::::::::: 136 7.2.2 Sparse Network Alignment Using Belief Propagation :::: 138 7.2.3 Tissue-specific Random Model (TRAM) for Generating Pseudo- random Tissues ::::::::::::::::::::::::: 139 7.2.4 Significance of Network Alignments :::::::::::::: 141 7.2.5 Differential Expression of Genes with Respect to a Group of Tissues :::::::::::::::::::::::::::::: 142 7.2.6 Conservation of Genesets Based on the Majority Voting Rule 143 7.3 Results and Discussion ::::::::::::::::::::::::: 144 7.3.1 Aligning Yeast Interactome with Human Tissue-specific Net- works :::::::::::::::::::::::::::::: 146 7.3.2 Investigating Roles of Housekeeping
Details
-
File Typepdf
-
Upload Time-
-
Content LanguagesEnglish
-
Upload UserAnonymous/Not logged-in
-
File Pages201 Page
-
File Size-