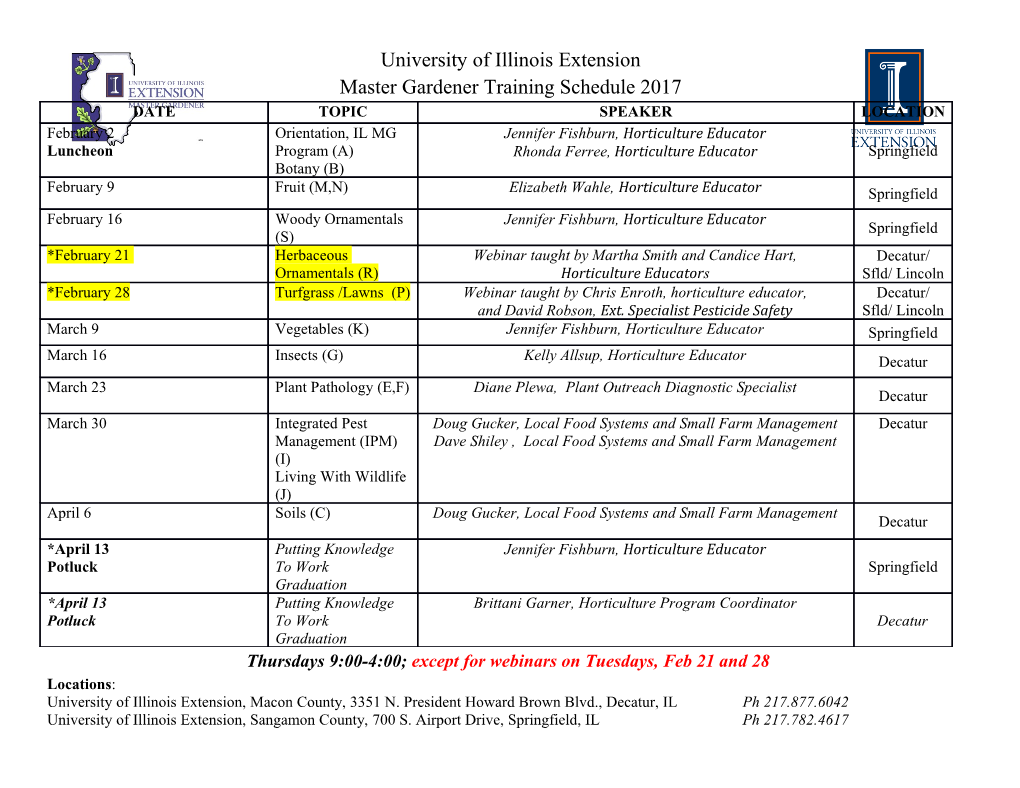
Rob J Hyndman Forecasting using 3. Autocorrelation and seasonality OTexts.com/fpp/2/ OTexts.com/fpp/6/1 Forecasting using R 1 Outline 1 Time series graphics 2 Seasonal or cyclic? 3 Autocorrelation Forecasting using R Time series graphics 2 Time series graphics Time plots R command: plot or plot.ts Seasonal plots R command: seasonplot Seasonal subseries plots R command: monthplot Lag plots R command: lag.plot ACF plots R command: Acf Forecasting using R Time series graphics 3 Time series graphics Economy class passengers: Melbourne−Sydney plot(melsyd[,"Economy.Class"]) 30 25 20 15 Thousands 10 5 0 1988 1989 1990 1991 1992 1993 Year Forecasting using R Time series graphics 4 Time series graphics Antidiabetic drug sales 30 > plot(a10) 25 20 15 $ million 10 5 1995 2000 2005 Year Forecasting using R Time series graphics 5 Time series graphics Seasonal plot: antidiabetic drug sales 30 2008 ● 2007 ● ● 2007 ● 25 ● ● 2006 ● ● ● ● ● ● 2006 ● ● ● ● 2005 ● ● ● ● 2005 20 ● ● ● ● 2004 ● ● 2004 ● ● ● ● ● ● ● ● ● 2003 ● ● ● ● ● ● 2003 2002 ● ● ● ● ● ● $ million ● 2002 15 ● ● 2001 ● ● ● ● ● ● ● ● ● ● ● ● ● ● ● ● ● ● 2001 2000 ● ● ● ● ● ● ● 2000 ● ● ● 1999 ● ● ● ● ● ● ● ● ● ● 1998 1999 ● ● ● ● ● ● ● 1997 10 ● ● ● ● ● ● ● ● ● ● ● 1998 ● ● 1996 19961997 ● ● ● ● ● ● ● ● ● ● ● ● ● ● ● ● ● ● 19931995 ● ● ● ● ● 19941995 ● ● ● ● ● ● ● ● ● ● ● ● 1993 ● ● ● 1994 ● ● ● ● ● ● ● 1992 ● ● ● ● ● ● ● ● ● 5 1992 ● ● ● ● ● ● ● ● ● ● ● ● ● ● ● 1991 ● ● ● ● ● ● ● ● ● ● ● ● ● ● ● ● ● ● ● Jan Feb Mar Apr May Jun Jul Aug Sep Oct Nov Dec Year Forecasting using R Time series graphics 6 Seasonal plots Data plotted against the individual “seasons” in which the data were observed. (In this case a “season” is a month.) Something like a time plot except that the data from each season are overlapped. Enables the underlying seasonal pattern to be seen more clearly, and also allows any substantial departures from the seasonal pattern to be easily identified. In R: seasonplot Forecasting using R Time series graphics 7 Seasonal plots Data plotted against the individual “seasons” in which the data were observed. (In this case a “season” is a month.) Something like a time plot except that the data from each season are overlapped. Enables the underlying seasonal pattern to be seen more clearly, and also allows any substantial departures from the seasonal pattern to be easily identified. In R: seasonplot Forecasting using R Time series graphics 7 Seasonal plots Data plotted against the individual “seasons” in which the data were observed. (In this case a “season” is a month.) Something like a time plot except that the data from each season are overlapped. Enables the underlying seasonal pattern to be seen more clearly, and also allows any substantial departures from the seasonal pattern to be easily identified. In R: seasonplot Forecasting using R Time series graphics 7 Seasonal plots Data plotted against the individual “seasons” in which the data were observed. (In this case a “season” is a month.) Something like a time plot except that the data from each season are overlapped. Enables the underlying seasonal pattern to be seen more clearly, and also allows any substantial departures from the seasonal pattern to be easily identified. In R: seasonplot Forecasting using R Time series graphics 7 Seasonal subseries plots Seasonal subseries plot: antidiabetic drug sales 30 > monthplot(a10) 25 20 15 $ million 10 5 Jan Feb Mar Apr May Jun Jul Aug Sep Oct Nov Dec Month Forecasting using R Time series graphics 8 Seasonal subseries plots Data for each season collected together in time plot as separate time series. Enables the underlying seasonal pattern to be seen clearly, and changes in seasonality over time to be visualized. In R: monthplot Forecasting using R Time series graphics 9 Seasonal subseries plots Data for each season collected together in time plot as separate time series. Enables the underlying seasonal pattern to be seen clearly, and changes in seasonality over time to be visualized. In R: monthplot Forecasting using R Time series graphics 9 Seasonal subseries plots Data for each season collected together in time plot as separate time series. Enables the underlying seasonal pattern to be seen clearly, and changes in seasonality over time to be visualized. In R: monthplot Forecasting using R Time series graphics 9 Quarterly Australian Beer Production beer <- window(ausbeer,start=1992) plot(beer) seasonplot(beer,year.labels=TRUE) monthplot(beer) Forecasting using R Time series graphics 10 Time series graphics Australian quarterly beer production 500 450 megaliters 400 1995 2000 2005 Forecasting using R Time series graphics 11 Time series graphics Seasonal plot: quarterly beer production ● 19941992 ● 1997 ● 1999 ● 19981995 ● 1993 ● 20021996 ● 2000 500 ● 200320062001 ● 2005 ● 2007 ● 2004 19942001 ● 450 1992 ● megalitres ● 2006 ● 19971993199920032004 ● 19982002 ● 19952007 ● 2000 ● ● ● 2008 ● ● 2005 ● ● ● ● 1996 ● ● ● ● ● 400 ● ● ● ● ● Q1 Q2 Q3 Q4 Quarter Forecasting using R Time series graphics 12 Time series graphics Seasonal subseries plot: quarterly beer production 500 450 Megalitres 400 Jan Apr Jul Oct Quarter Forecasting using R Time series graphics 13 Outline 1 Time series graphics 2 Seasonal or cyclic? 3 Autocorrelation Forecasting using R Seasonal or cyclic? 14 Time series patterns Trend pattern exists when there is a long-term increase or decrease in the data. Seasonal pattern exists when a series is influenced by seasonal factors (e.g., the quarter of the year, the month, or day of the week). Cyclic pattern exists when data exhibit rises and falls that are not of fixed period (duration usually of at least 2 years). Forecasting using R Seasonal or cyclic? 15 Time series patterns Australian electricity production 14000 12000 GWh 10000 8000 1980 1985 1990 1995 Year Forecasting using R Seasonal or cyclic? 16 Time series patterns Australian clay brick production 600 500 400 million units 300 200 1960 1970 1980 1990 Year Forecasting using R Seasonal or cyclic? 17 Time series patterns Sales of new one−family houses, USA 90 80 70 60 Total sales Total 50 40 30 1975 1980 1985 1990 1995 Forecasting using R Seasonal or cyclic? 18 Time series patterns US Treasury bill contracts 91 90 89 88 price 87 86 85 0 20 40 60 80 100 Day Forecasting using R Seasonal or cyclic? 19 Time series patterns Annual Canadian Lynx trappings 7000 6000 5000 4000 3000 Number trapped 2000 1000 0 1820 1840 1860 1880 1900 1920 Time Forecasting using R Seasonal or cyclic? 20 Seasonal or cyclic? Differences between seasonal and cyclic patterns: seasonal pattern constant length; cyclic pattern variable length average length of cycle longer than length of seasonal pattern magnitude of cycle more variable than magnitude of seasonal pattern The timing of peaks and troughs is predictable with seasonal data, but unpredictable in the long term with cyclic data. Forecasting using R Seasonal or cyclic? 21 Seasonal or cyclic? Differences between seasonal and cyclic patterns: seasonal pattern constant length; cyclic pattern variable length average length of cycle longer than length of seasonal pattern magnitude of cycle more variable than magnitude of seasonal pattern The timing of peaks and troughs is predictable with seasonal data, but unpredictable in the long term with cyclic data. Forecasting using R Seasonal or cyclic? 21 Seasonal or cyclic? Differences between seasonal and cyclic patterns: seasonal pattern constant length; cyclic pattern variable length average length of cycle longer than length of seasonal pattern magnitude of cycle more variable than magnitude of seasonal pattern The timing of peaks and troughs is predictable with seasonal data, but unpredictable in the long term with cyclic data. Forecasting using R Seasonal or cyclic? 21 Seasonal or cyclic? Differences between seasonal and cyclic patterns: seasonal pattern constant length; cyclic pattern variable length average length of cycle longer than length of seasonal pattern magnitude of cycle more variable than magnitude of seasonal pattern The timing of peaks and troughs is predictable with seasonal data, but unpredictable in the long term with cyclic data. Forecasting using R Seasonal or cyclic? 21 Seasonal or cyclic? Differences between seasonal and cyclic patterns: seasonal pattern constant length; cyclic pattern variable length average length of cycle longer than length of seasonal pattern magnitude of cycle more variable than magnitude of seasonal pattern The timing of peaks and troughs is predictable with seasonal data, but unpredictable in the long term with cyclic data. Forecasting using R Seasonal or cyclic? 21 Outline 1 Time series graphics 2 Seasonal or cyclic? 3 Autocorrelation Forecasting using R Autocorrelation 22 Autocorrelation Covariance and correlation: measure extent of linear relationship between two variables (y and X). Autocovariance and autocorrelation: measure linear relationship between lagged values of a time series y. We measure the relationship between: yt and yt−1 yt and yt−2 yt and yt−3 etc. Forecasting using R Autocorrelation 23 Autocorrelation Covariance and correlation: measure extent of linear relationship between two variables (y and X). Autocovariance and autocorrelation: measure linear relationship between lagged values of a time series y. We measure the relationship between: yt and yt−1 yt and yt−2 yt and yt−3 etc. Forecasting using R Autocorrelation 23 Autocorrelation Covariance and correlation: measure extent of linear relationship between two variables (y and X). Autocovariance and autocorrelation: measure linear
Details
-
File Typepdf
-
Upload Time-
-
Content LanguagesEnglish
-
Upload UserAnonymous/Not logged-in
-
File Pages58 Page
-
File Size-