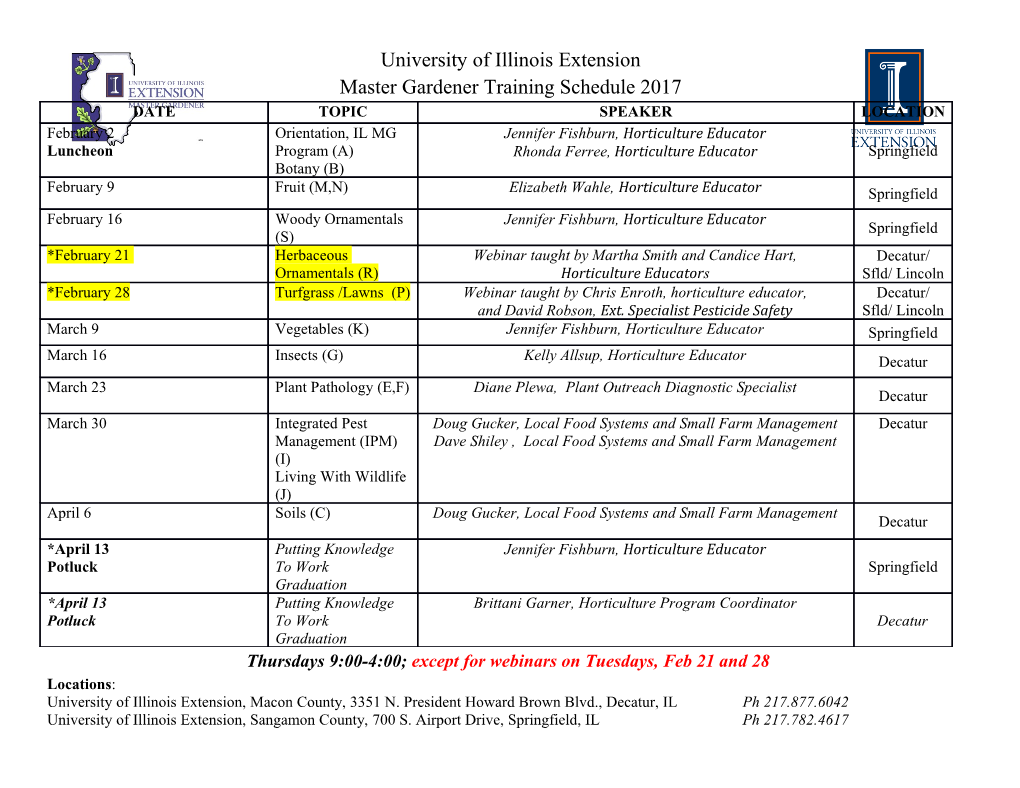
Machine learning for Monte-Carlo integration Valentin Hirschi ACAT 11th January 2019 Plan • Type of integrals appearing in HEP • Standard phase-space integration techniques • Current numerical methods for loop integrals • Machine learning assisted MC-integration • Prospects Valentin Hirschi, ETHZ Machine-learning for MC HEP simulations ACAT 11.01.2019 Plan • Type of integrals appearing in HEP • Standard phase-space integration techniques • Current numerical methods for loop integrals • Machine learning assisted MC-integration • Prospects Valentin Hirschi, ETHZ Machine-learning for MC HEP simulations ACAT 11.01.2019 Cross-section computation + ` `− x1E x2E µF µF p p long distance long distance dx1dx2dΦFS fa(x1,µF )fb(x2,µF ) ⇥ˆab X (ˆs, µF ,µR) a,b → Phase-space Parton density Parton-level cross integral functions section Dim[Φ(n)] ∼ 3n Peaked function Valentin Hirschi, ETHZ Machine-learning for MC HEP simulations ACAT 11.01.2019 Phase-space integrals 4 4 2 2 2 4 d p1 ...d pN ( p ) δp ...δp δ (p + ...p ) |M { i} | 1 N 1 N Z • Need a parametrisation to solve the deltas, e.g. N=2 : N 2 2 2 2 s =(p1 + p2) t =(p + p ) u =(p + p ) = M s t 1 3 1 4 i − − i X • Neural networks may help to cope with the high PS dim ( d =3 N 3 ) and peak structure of PDF & matrix elements. − • Timings Tree-level One-loop Two-loop MG5aMC MadLoop VVamp [arXiv:1405.0301] [arXiv:1103.0621] [arXiv:1503.08835] dd¯ ZZ 7 µs x 102 0.6 ms x 104 (1 min) ! O dd¯ ZZg 35 µs x 103 38 ms N/A ! dd¯ ZZgg 220 µs x 104 1200 ms N/A ! Valentin Hirschi, ETHZ Machine-learning for MC HEP simulations ACAT 11.01.2019 Loop integrals d d N( ki ) d k1 ...d kNl { } Nd Z ⇧1 Di • Dimensionality fixed by number of loops, not ext. kinematics • Features mass threshold singularities and IR+UV divergences • Polynomial integrand, fast evaluation time for all topologies • Advanced analytic integration techniques developed, but purely numerical methods are now (re-)surfacing. • Machine learning can help cope with the large variance of the resulting integrands. Valentin Hirschi, ETHZ Machine-learning for MC HEP simulations ACAT 11.01.2019 Plan • Type of integrals appearing in HEP • Standard phase-space integration techniques • Current numerical methods for loop integrals • Machine learning assisted MC-integration • Prospects Valentin Hirschi, ETHZ Machine-learning for MC HEP simulations ACAT 11.01.2019 Plan • Type of integrals appearing in HEP • Standard phase-space integration techniques • Current numerical methods for loop integrals • Machine learning assisted MC-integration • Prospects Valentin Hirschi, ETHZ Machine-learning for MC HEP simulations ACAT 11.01.2019 Importance sampling 1.0 1.0 0.8 0.8 0.6 0.6 0.4 0.4 0.2 0.2 IN =0.637 0.307/√N ± 0.0 0.0 0.0 0.1 0.2 0.3 0.4 1 π 0.0 0.2 0.4 0.6 0.8 1.0 0.5 1 ⇡ 1 π ⇡ I = dx cos x 2 cos 2 x ξ2 cos2 cos2 x[ξ2] x 2 I = dx(1 cx ) I = = !dx(1dξcx ) 2 !0 − (1 cx2) ξ1 − 1−(1x[ξ] cx2) Z0 − Z0 − ≃ 1 IN =0.637 0.307/√N IN =0.637 0.031/√N ± ± Phase-Space parametrisation is key to tame the variance Valentin Hirschi, ETHZ Machine-learning for MC HEP simulations ACAT 11.01.2019 Importance sampling dq2 (q2 M 2 + iMΓ)2 Z − q2 M 2 ⇠ = arctan − Γ M ✓ ◆ Why Importance Sampling? Probability of using that point p(x) The change of variable ensures that the evaluation of the integrand is done where it is largest Valentin Hirschi, ETHZ Machine-learning for MC HEP simulations ACAT 11.01.2019 Importance sampling Adaptative Monte-Carlo • Create a piece-wise approximation of the function on the fly Essence of the implementation 1. Setup bins such that each of them contain the same contribution. ➡ Many bins where integrand is large 2. Use the resulting approximation for importance sampling. Valentin Hirschi, ETHZ Machine-learning for MC HEP simulations ACAT 11.01.2019 VEGAS More than one Dimension • VEGAS works only with 1(few) dimension ➡ Otherwise number of “bins” scales like Nd :( Valentin Hirschi, ETHZ Machine-learning for MC HEP simulations ACAT 11.01.2019 VEGAS More than one Dimension • VEGAS works only with 1(few) dimension ➡ Otherwise number of “bins” scales like Nd :( Solution • Factorisation ansatz: projection on the axis → p(x)= p(x)•p(y)•p(z)… Valentin Hirschi, ETHZ Machine-learning for MC HEP simulations ACAT 11.01.2019 VEGAS More than one Dimension • VEGAS works only with 1(few) dimension ➡ Otherwise number of “bins” scales like Nd :( Solution • Factorisation ansatz: projection on the axis → p(x)= p(x)•p(y)•p(z)… Valentin Hirschi, ETHZ Machine-learning for MC HEP simulations ACAT 11.01.2019 VEGAS More than one Dimension • VEGAS works only with 1(few) dimension ➡ Otherwise number of “bins” scales like Nd :( Solution • Factorisation ansatz: projection on the axis → p(x)= p(x)•p(y)•p(z)… • Factorisation breaks down ➡Additional change of variable needed Valentin Hirschi, ETHZ Machine-learning for MC HEP simulations ACAT 11.01.2019 Monte Carlo integration Monte CarloVEGAS technics choice of the phase-space parametrization has a strong impact on the efficiencyefficiency of of the anMC adaptative integration MC: integration : •The choice of the parameterisation has a anycase peakstrong 1 : is any aligned peakimpact is alongaligned on athe singlealong efficiency direction a single direction of the P-S of the P-S parametrizationparametrization y2 y2 y2 y2 Grid y1 y1 y1 y1 thetheadaptiveadaptative Monte-Carlo Monte-Carlo P-S P-S integration integrationisis veryvery efficient efficient →→ Valentin Hirschi, ETHZ Machine-learning for MC HEP simulations ACAT 11.01.2019 MadWeight – p. 12/29 MadWeight – p. 7/17 MonteMonte Carlo Carlo integration integration Monte Carlo integration Monte Carlo integrationVEGAS choicechoice of the of thephase-spacephase-space parametrization parametrizationhashas a strong a strong impact impact on the on the choice of the phase-space parametrization has a strong impact on the choiceefficiencyefficiency of the ofphase-space the of theMCMC integration parametrization integration: : has a strong impact on the efficiency of the MC integration : efficiency of• theTheMC choice integration of :the parameterisation has a solutionsomesolutionstrong to peaks the to the previous impact are previous not case aligned caseon the : : perform perform along efficiency a a a change single change of direction ofvariables variables ofin the orderin P-S order some peaks are not aligned along a single direction of the P-S to alignparametrizationto align the the peaks peaks along along a a single single direction of of the the P-S P-S paramet parametrizationrization parametrization yy11++y2y2 y2 y2 y2 y2 y2 y2 Grid y1 y2 y1 − y1 y1 y2 y1 y1 1 y1 y1 − the adaptive Monte-Carlo P-S integration is very efficient the adaptive Monte-Carlo P-S integration converges slowly → the→ theadaptiveadaptive Monte-Carlo Monte-Carlo P-S P-S integration integrationis veryconverges efficient slowly → → MadWeight – p. 12/29 Valentin Hirschi, ETHZ Machine-learning for MC HEP simulations MadWeightACAT – p. 12/29 11.01.2019 MadWeight – p. 12/29 MadWeight – p. 12/29 Monte Carlo integration MonteMonte Carlo Carlo integration integration Monte Carlo integrationVEGAS has a strong impact on the choice of the phase-space parametrization has a strong impact on the choicechoice of the ofphase-space the phase-space parametrization parametrizationhas a stronghas a strongimpact onimpact the on the choiceefficiency of the ofphase-space the MC integration parametrization: has a strong impact on the efficiencyefficiency of the ofMC the integrationMC integration: : efficiency of• theTheMC choice integration of :the parameterisation: has a solution to the previous case : perform a change of variables in order y 1 solutionsolutionstrong to the to impact previous the previous caseon the case: perform efficiency : perform a change a change of variables of variablesy 2 in orderin order some peaks are not aligned along a single direction of the P-S to alignto align the the peaks peaks along along a a single single direction of of the the P-S P-S paramet parametrizationrization MadWeight – p. 12/29 to align the peaks along aphase-space single direction parametrization of the P-S parametrization parametrization MC integration yy11+ y2y2 y2 y2 + y1 + y2 converges slowly y2 y2 y2 Monte Carlo integrationRotation choice of the efficiency of the y 2 y 1 someGrid peaks are not aligned along a single direction of the P-S parametrization y1 y2 y1 − y1 y1 y2y1 y2 y1 y1 y1 − − the adaptive Monte-Carlo P-S integration is very efficient thethe→adaptiveadaptivethe Monte-Carloadaptive Monte-Carlo Monte-Carlo P-S integration P-S integration P-Sconverges integrationis very slowlyis very efficient efficient →→ → adaptive Monte-Carlo P-S integration the MadWeight – p. 12/29 MadWeight – p. 12/29 Valentin Hirschi, ETHZ Machine-learning for MC HEP simulations ACAT 11.01.2019MadWeight – p. 12/29 → MadWeight – p. 12/29 MULTI-CHANNEL It is often the case that no transformation can align all integrand peaks to the chosen axes. Valentin Hirschi, ETHZ Machine-learning for MC HEP simulations ACAT 11.01.2019 MULTI-CHANNEL It is often the case that no transformation can align all integrand peaks to the chosen axes. Solution: combine different transformations = channels n n p(x)=! αipi(x) with ! αi =1 i=1 i=1 with each pi(x) taking care of one “peak” at the time Valentin Hirschi, ETHZ Machine-learning for MC HEP simulations ACAT 11.01.2019 MULTI-CHANNEL n p(x)=! αipi(x) i=1 with n ! αi =1 i=1 p1(x) p2(x) Valentin Hirschi, ETHZ Machine-learning for MC HEP simulations ACAT 11.01.2019 MULTI-CHANNEL n p(x)=! αipi(x) i=1 with n ! αi =1 i=1 Then, f(x) n f(x) I
Details
-
File Typepdf
-
Upload Time-
-
Content LanguagesEnglish
-
Upload UserAnonymous/Not logged-in
-
File Pages52 Page
-
File Size-