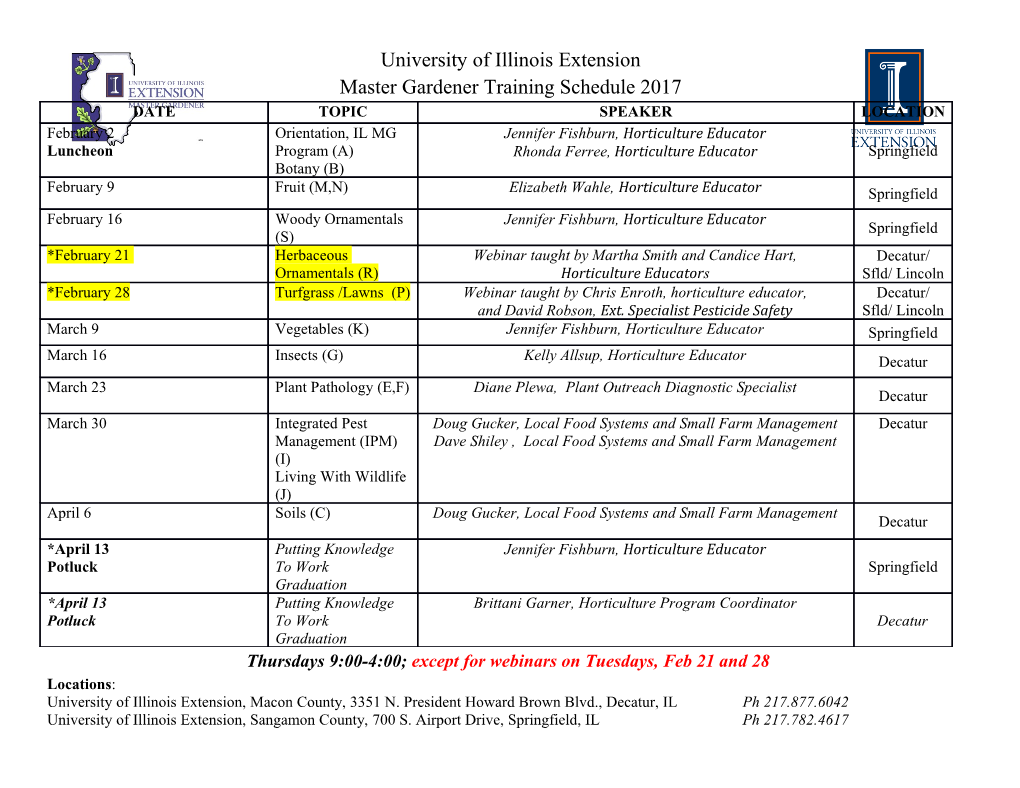
A Characterization of Mean Squared Error for Estimator with Bagging Martin Mihelich Charles Dognin Yan Shu Michael Blot Open Pricer Verisk | AI, Verisk Analytics Walnut Algorithms Walnut Algorithms École Normale Supérieure Abstract 1 Introduction Since the popular paper (Breiman, 1996), bootstrap ag- Bagging can significantly improve the gen- gregating (or bagging) has become prevalent in machine eralization performance of unstable machine learning applications. This method considers a number learning algorithms such as trees or neural net- N of samples from the dataset, drawn uniformly with works. Though bagging is now widely used in replacement (bootstrapping) and averages the different practice and many empirical studies have ex- estimations computed on the different sub-sets in order plored its behavior, we still know little about to obtain a better (bagged) estimator. In this article, the theoretical properties of bagged predic- we theoretically investigate the ability of bagging to tions. In this paper, we theoretically inves- reduce the Mean Squared Error (MSE) of a statistical tigate how the bagging method can reduce estimator with an additional focus on the MSE of the the Mean Squared Error (MSE) when applied variance estimator with bagging. on a statistical estimator. First, we prove In a machine learning context, bagging is an ensemble that for any estimator, increasing the number method that is effective at reducing the test error of pre- of bagged estimators N in the average can dictors. Several convincing empirical results highlight only reduce the MSE. This intuitive result, this positive effect (Webb and Zheng, 2004; Mao, 1998). observed empirically and discussed in the lit- However, those observations cannot be generalized to erature, has not yet been rigorously proved. every algorithm and dataset since bagging deteriorates Second, we focus on the standard estimator of the prediction performance in some cases (Grandvalet, variance called unbiased sample variance and 2004). Unfortunately, it is difficult to understand the we develop an exact analytical expression of reasons explaining why the behavior of bagging dif- the MSE for this estimator with bagging. This fers from one application to another. In fact, only a allows us to rigorously discuss the number of few articles give theoretical interpretations (Bühlmann iterations N and the batch size m of the bag- et al., 2002; Friedman and Hall, 2007; Buja and Stuet- ging method. From this expression, we state zle, 2016). The difficulty to obtain theoretical results that only if the kurtosis of the distribution is partially due to the huge number of bootstrap pos- 3 is greater than 2 , the MSE of the variance sibilities for the estimator with bagging. For instance, estimator can be reduced with bagging. This in (Buja and Stuetzle, 2006; Xi Chen and Hall, 2003; result is important because it demonstrates Buja and Stuetzle, 2016), authors studied properties that for distribution with low kurtosis, bag- of bagging-statistic, which considers all possible boot- ging can only deteriorate the performance of strap samples in bagging, and provided a theoretical a statistical prediction. Finally, we propose a understanding regarding the relationship between the novel general-purpose algorithm to estimate sample size and the batch size. However, in practice, with high precision the variance of a sample. one cannot consider all possible bootstrap samples. As a result, bagging estimators, which considers a number of randomly constructed samples in bagging are always used. The bagging-statistics studied in the literature (Buja and Stuetzle, 2006; Xi Chen and Hall, 2003; Buja Proceedings of the 23rdInternational Conference on Artificial and Stuetzle, 2016) considers the mean of bagging sam- Intelligence and Statistics (AISTATS) 2020, Palermo, Italy. ples and the bagging estimator considers the average of PMLR: Volume 108. Copyright 2020 by the author(s). randomly selected bagging samples, which have more A Characterization of Mean Squared Error for Estimator with Bagging randomness (We will detail the mathematical difference Stuetzle, 2006) on the bagging-statistics of biased in section 2.1). These insufficient theoretical guidelines variance estimator. We include this proof and a generally lead to arbitrary choices of the fundamental short comparison of (Buja and Stuetzle, 2006) in parameters of bagging, such as the batch size m and the supplementary material. the number of iterations N. • Finally, we provide various experiments illustrating In this paper, we investigate the theoretical proper- and supporting our theoretical results. ties of bagging applied to statistical estimators and specifically the impact of bagging on the MSE of those The rest of the paper is organized as follows. In Sec- estimators. We provide three core contributions: tion 2 we present the mathematical framework along with our derivation of the MSE for any bagged estima- • We give a rigorous mathematical demonstration tors. In Section 3, we focus on the particular case of (proof) that the bias of any estimator with bag- the bagged unbiased sample variance estimator and we ging is independent from the number of iterations provide a new criteria on the variable kurtosis. In Sec- N, while the variance linearly decreases in 1 . N tion 4, we support our theoretical findings with three This intuitive behavior, observed in several pa- experiments. pers (Breiman, 1996; Liu et al., 2018), has not yet been demonstrated. We also provide recommen- dations for the choice of the number of iterations 2 Bagged Estimators: The More The N and the batch size m. We further discuss the Better implication in a machine learning context. To demonstrate these points, we develop a mathemat- In this section we rigorously define the notations before ical framework which enables us, with a symmetric presenting the derivation of the different components argument, to obtain an exact analytical expression of the MSE of a bagged statistical estimator, namely for the bias and variance of any estimator with the bias and the variance. We subsequently deduce bagging. This symmetric technique can be used our first contribution: the more iterations N used in to calculate many other metrics on estimators, we the bagging algorithm, the smaller the MSE is, with a hope that our findings will enable more research linear dependency. in the area. 2.1 Notations, Definitions • We use our framework to further study bagging ap- plied to the standard estimator of variance called A dataset, denoted ` = (xi)i2f1;:::ng, is the realization unbiased sample variance. This estimator is widely of an independent and identically distributed sample used and a lot of work has been done on specific set, noted L = (X1; :::; Xn), of a variable X 2 X .A versions, such as the Jackknife variance estimator statistic of the variable X is noted θ(X) 2 R. An (Efron and Stein, 1981) or the variance estimator estimator of θ, computed from L, is noted θ^(L). with replacement (Cho and Cho, 2009). In this paper, we derive an exact analytical formula for By considering U : f1; ::; mg 7! f1; ::; ng, a random the MSE of the variance estimator with bagging. function, we denote LU = (XU(1); :::; XU(m)) a uniform It allows us to provide a simple criteria based on sampling with replacement from L of size m. The the kurtosis of the sample distribution, which char- random variable U is defined on U = f(uk)k=1···nm g, acterizes whether or not the bagging will have a the finite set of all m sized sampling with replacement, positive impact on the MSE of the variance esti- of cardinal nm. The bagging method considers N mator. We find that on average, applying bagging such sampling functions taken uniformly from U, noted to the variance estimator reduces the MSE if and B = (U 1; :::; U N ). We can now define the bagging only if the kurtosis of the sample distribution is estimator: above 3 and the number of bagging iterations N 2 N N is large enough. From this theorem, we are able to 1 X 1 X θ~(L; B) = θ^(L i ) = θ^(X i ; :::; X i ): propose a novel, more accurate variance estimation N U N U (1) U (m) i=1 i=1 algorithm. This result is particularly interesting (1) since it describes explicit configurations where the application of bagging cannot be beneficial. We Usually, the size of the sample sets is n. Here, we further discuss how this result fits into common ^ intuitions in machine learning. consider the general case where θ is a function of a set of any size m. The number N of iterations is often • As a byproduct, using our framework, we provide set to N 2 [j10; 100j] (where [ja; bj] represents all the an alternative proof to the results in (Buja and natural numbers between a and b) without rigorous Martin Mihelich, Charles Dognin, Yan Shu, Michael Blot ^ ^ justification (Breiman, 1996; Dietterich, 2000; Lemmens Remark 2.1 EL VarU (θ(LU )) , VarL EU (θ(LU )) and Croux, 2006). We discuss this parameter in the ^ 2 and EL(EU (θ(LU ))) − θ are positive and do not following section. depend on N. We deduce that: The average with respect to L is noted EL, and with respect to B; U is noted EB and EU . We assume that 1. The higher the N, the lower the MSE. m 2 8i 2 [j1; n j], E (θ^(L i ) ) < 1. Since B is defined L U 2. As N goes to 1, we have: on a finite set, we define E(L;B) which is the expected value taken with respect to the pair of random variables lim MSE(θ~) N!1 (L; B) and we have E(L;B) = EL(EB) = EB(EL). Us- ^ ^ 2 ing this notation, we remark that the bagging-statistics = VarL EU (θ(LU )) + EL(EU (θ(LU ))) − θ : studied in the literature (Buja and Stuetzle, 2006; Xi Chen and Hall, 2003; Buja and Stuetzle, 2016) is In this last expression we find the classical MSE decom- ^ ~ EU (θ(LU ), whereas θ(L; B) defined in equation (1) is position in terms of bias and variance.
Details
-
File Typepdf
-
Upload Time-
-
Content LanguagesEnglish
-
Upload UserAnonymous/Not logged-in
-
File Pages9 Page
-
File Size-