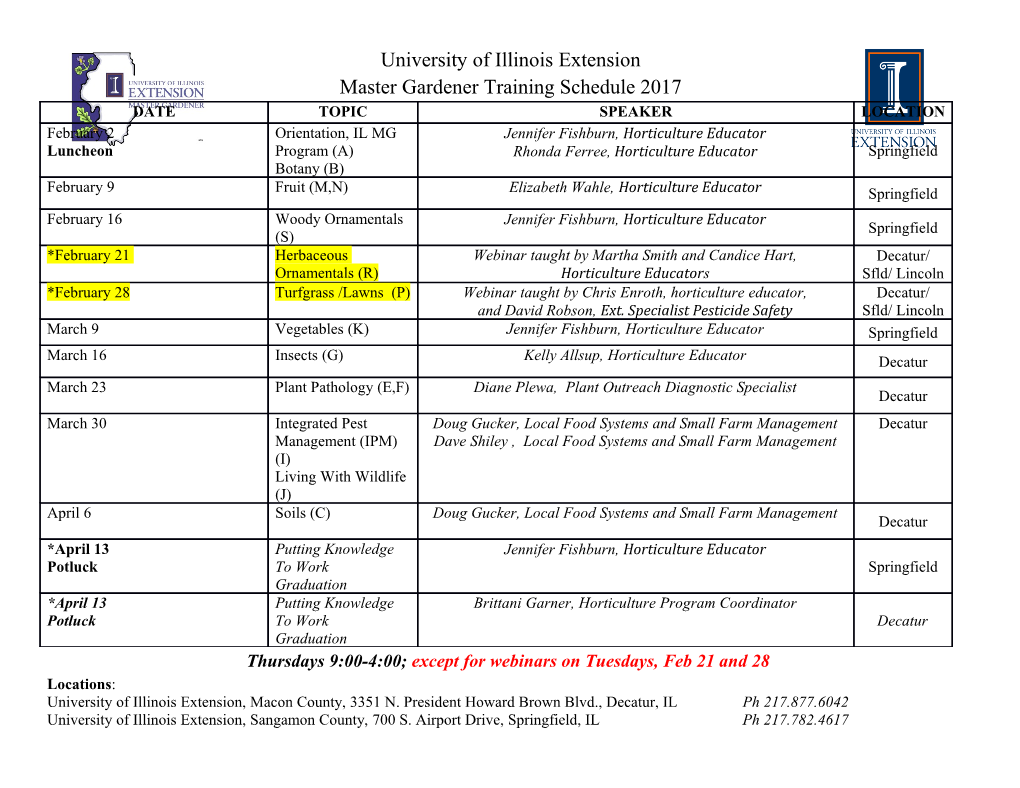
Behavior-oriented Modeling of Electric Vehicle Load Profiles: A Stochastic Simulation Model Considering Different Household Characteristics, Charging Decisions and Locations Alexander Harbrecht, Russell McKenna, David Fischer, Wolf Fichtner No. 29 | April 2018 WORKING PAPER SERIES IN PRODUCTION AND ENERGY KIT – The Research University in the Helmholtz Association www.iip.kit.edu Behavior-oriented Modeling of Electric Vehicle Load Profiles: A Stochastic Simulation Model Considering Different Household Characteristics, Charging Decisions and Locations Alexander Harbrecht, Russell McKenna*, David Fischer, Wolf Fichtner *Corresponding author: [email protected], +49 721 6084 4582, IIP, Building 06.33, Hertzstr. 16, 76187 Karlsruhe, Germany. This paper presents a stochastic bottom-up model to assess electric vehicles' (EV) impact on load profiles at different parking locations as well as their load management potential assuming different charging strategies. The central innovation lies in the consideration of socio-economic, technical and spatial factors, all of which influence charging behavior and location. Based on a detailed statistical analysis of a large dataset on German mobility, the most statistically significant influencing factors on residential charging behavior could be identified. Whilst household type and economic status are the most important factors for the number of cars per household, the driver's occupation has the strongest influence on the first departure time and parking time whilst at work. An inhomogeneous Markov-chain is used to sample a sequence of destinations of each car trip, depending (amongst other factors) on the occupation of the driver, the weekday and the time of the day. Probability distributions for the driven kilometres, driving durations and parking durations are used to derive times and electricity demand. The probability distributions are retrieved from a national mobility dataset of 70,000 car trips and filtered for a set of socio-economic and demographic factors. Individual charging behaviour is included in the model using a logistic function accounting for the sensitivity of the driver towards (low) battery SOC. The presented model is validated with this mobility dataset and shown to have a deviation in key household mobility characteristics of just a few percentage points. The model is then employed to analyse the impact of uncontrolled charging of BEV on the residential load profile. It is found that the absolute load peaks will increase by up to factor 8.5 depending on the loading infrastructure, the load in high load hours will increase by approx. a factor of 3 and annual electricity demand will approximately double. Contents Table of Contents II List of Figures IV List of Tables VIII List of Abbreviations XI 1 Introduction 1 2 Fundamentals 3 2.1 Mobilityandtransportation . 3 2.2 Electricvehicles(EV). 5 2.3 ImpactsofEVchargingonthepowersystem. 8 2.4 InfluencingfactorsonEVcharging . 9 3 Differentiation of households’ driving behavior 13 3.1 Dataused .................................... 13 3.1.1 MobilityinGermany(MiD) . 13 3.1.2 Comparison with other German mobility studies . 16 3.1.3 Datapreparation ............................ 17 3.1.4 Selection of dependent variables and MiD datasets . 22 3.2 Statisticalmethodsused . 27 3.2.1 Categoricaldata ............................ 27 3.2.2 Numericaldata ............................. 33 3.3 Resultsanddiscussion ............................. 44 3.3.1 Householdlevel ............................. 48 3.3.2 Vehiclelevel............................... 52 3.3.3 Triplevel ................................ 63 3.3.4 Criticalsummary ............................ 80 4 Implementation of synPRO-emobility 85 4.1 Modeloverview ................................. 85 4.1.1 Goalandapproach ........................... 85 4.1.2 Generalstructure ............................ 87 4.1.3 Inputdata................................ 88 4.1.4 Outputdata............................... 96 4.2 FromdatatoBEVchargingprofiles. 99 4.2.1 Generationofdrivingprofiles . 99 4.2.2 Representation of charging decisions, stations and quantities . 103 4.3 Validationandexemplaryresults . 109 4.3.1 Validationrunsanddiscussion. 115 4.3.2 Criticalappraisal . .. .125 4.3.3 Impact of different household types and charging preferences . 127 5 Conclusion and outlook 128 6 References 131 II A Appendix for Chapter 3 138 A.1 AdditionalinformationonMiD . 138 A.1.1 Surveymaterial .............................138 A.1.2 Importantderivedsurveyvariables . 141 A.2 Brown-Forsythetesttables. 147 A.2.1 Householdlevel .............................147 A.2.2 Vehiclelevel...............................148 A.2.3 Triplevel ................................149 A.3 Specified research questions and statistical hypotheses . ...........153 A.3.1 Householdlevel .............................153 A.3.2 Vehiclelevel...............................155 A.3.3 Triplevel ................................159 A.4 TesttablesforCliff’sMethod . 169 A.4.1 Householdlevel .............................169 A.4.2 Vehiclelevel...............................182 A.4.3 Triplevel ................................194 A.5 Additional χ2-testresultsandmosaicplots . 208 A.5.1 Householdlevel .............................208 A.5.2 Vehiclelevel...............................210 A.5.3 Triplevel ................................212 A.6 Summarytablesofmaineffects . 216 B Appendix for Chapter 4 222 B.1 Selectedcorrelationmatrices . 222 B.2 Syntheticloadprofiles . 227 III List of Figures 1 Overview of influencing factors on electric vehicle charging ......... 11 2 Determinable spatial information from the MiD trip dataset used to create logically consistent sequences of departure and arrival places ........ 20 3 Unshaded mosaic (left) and association plots (right) for example data (Zeileis etal.,2007,p.511) ............................... 29 4 Examples of probability density functions of chi-squared distributions (Agresti,2007,p.35) .............................. 30 5 Shaded mosaic plot for example data (Zeileis et al., 2007, p.516)...... 32 6 Distributional situation where Cliff’s δ isclosetozero. 41 7 Exemplary overview of interpreted results from test tables of Cliff’s method 46 8 Household level: overview of main effects on number of cars per household (cf.Table18) .................................. 48 9 Household level (χ2-test and mosaic plot): main effect of place of residence onhouseholdtype................................ 50 10 Histogram of driven trips distributed over the number of distinct drivers perhouseholdandcar ............................. 52 11 Vehicle level: overview of main effects on number of trips per (use) day (cf. Table38and39)(I) .............................. 53 12 Vehicle level: overview of main effects on number of trips per (use) day (cf. Table38and39)(II) .............................. 54 13 Vehicle level (χ2-test and mosaic plot): main effect of household type on mainuseroccupation.............................. 56 14 Vehicle level (χ2-test and mosaic plot): main effect of household type on mainuser(vehicle)usefrequency . 57 15 Vehicle level (χ2-test and mosaic plot): main effect of main user occupation onmainuser(vehicle)usefrequency . 58 16 Vehicle level (χ2-test and mosaic plot): main effect of place of residence on mainuser(vehicle)usefrequency . 59 17 Vehicle level (χ2-test and mosaic plot): main effect of main user (vehicle) usefrequencyonmainuser(dailyvehicle)use . 60 18 Vehicle level (χ2-test and mosaic plot): main effect of place of residence on mainuser(dailyvehicle)use . 61 19 Vehicle level (χ2-test and mosaic plot): main effect of weekday on main user(dailyvehicle)use ............................. 61 20 Trip level: overview of main effects on driven distance for H-W or W-H trips(cf.Table50) ............................... 63 21 Trip level: overview of main effects on driven distance for all trips except H-WorW-Htrips(cf.Table51,52,53) . 65 22 Trip level: overview of main effects on departure time for first H-W trips perday(cf.Table54) ............................. 67 23 Trip level: overview of main effects on departure time for first trips per day exceptH-Wtrips(cf.Table55) . 68 24 Trip level: overview of main effects on driving time for all H-W or W-H trips(cf.Table56) ............................... 70 25 Trip level: overview of main effects on driving time for all trips except H-W orW-Htrips(cf.Table57,58,59) . 72 IV 26 Trip level: overview of main effects on parking time for all H-W trips (cf. Table60) .................................... 73 27 Trip level: overview of main effects on parking time for all trips except H-W orW-Htrips(cf.Table61,62,63) . 74 28 Trip level (χ2-test and mosaic plot): main effect of main user occupation ondeparturearrivalplaces . 76 29 Trip level (χ2-test and mosaic plot): main effect of trip index on departure arrivalplaces .................................. 77 30 Trip level (χ2-test and mosaic plot): main effect of weekday on departure arrivalplaces .................................. 78 31 Trip level (χ2-test and mosaic plot): main effect of departure arrival places ontrippurpose ................................. 79 32 GeneralstructureofsynPRO-emobility . 87 33 Example overview of household/BEV configurations for a simulation in synPRO-emobility ............................... 89 34 Exemplary Battery Self-discharge Curve for a Parking Duration of 30 Days 93 35 Exemplary Active Power Charging Curve at 3.7 kW for a Parking Duration of2HoursandaSaturationChargeLevelof90% . 95 36 Overview of Input Parameters Categorized by Stochastic
Details
-
File Typepdf
-
Upload Time-
-
Content LanguagesEnglish
-
Upload UserAnonymous/Not logged-in
-
File Pages257 Page
-
File Size-