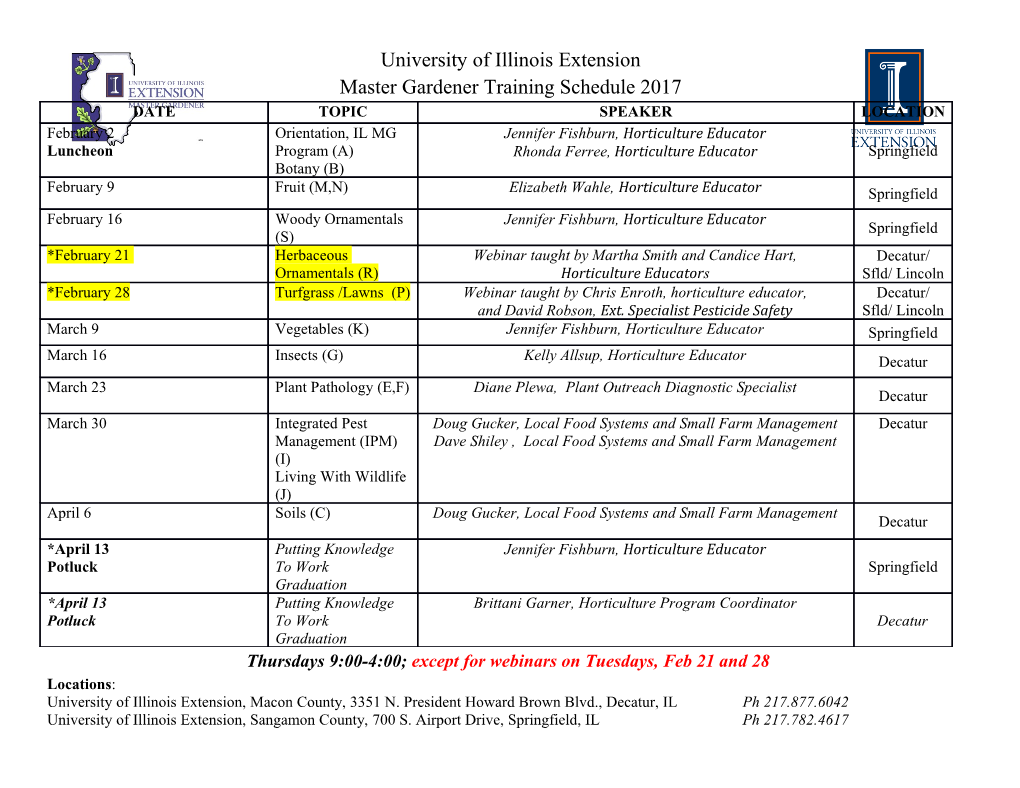
Fuzzy Relations, Rules and Inferences Debasis Samanta IIT Kharagpur [email protected] 06.02.2018 Debasis Samanta (IIT Kharagpur) Soft Computing Applications 06.02.2018 1 / 64 Fuzzy Relations Debasis Samanta (IIT Kharagpur) Soft Computing Applications 06.02.2018 2 / 64 Crisp relations To understand the fuzzy relations, it is better to discuss first crisp relation. Suppose, A and B are two (crisp) sets. Then Cartesian product denoted as A × B is a collection of order pairs, such that A × B = f(a; b)ja 2 A and b 2 Bg Note : (1) A × B 6= B × A (2) jA × Bj = jAj × jBj (3)A × B provides a mapping from a 2 A to b 2 B. The mapping so mentioned is called a relation. Debasis Samanta (IIT Kharagpur) Soft Computing Applications 06.02.2018 3 / 64 Crisp relations Example 1: Consider the two crisp sets A and B as given below. A =f 1, 2, 3, 4g B = f3, 5, 7 g. Then, A × B = f(1; 3); (1; 5); (1; 7); (2; 3); (2; 5); (2; 7); (3; 3); (3; 5); (3; 7); (4; 3); (4; 5); (4; 7)g Let us define a relation R as R = f(a; b)jb = a + 1; (a; b) 2 A × Bg Then, R = f(2; 3); (4; 5)g in this case. We can represent the relation R in a matrix form as follows. 3 5 7 1 2 0 0 0 3 2 6 1 0 0 7 R = 6 7 3 4 0 0 0 5 4 0 1 0 Debasis Samanta (IIT Kharagpur) Soft Computing Applications 06.02.2018 4 / 64 Operations on crisp relations Suppose, R(x; y) and S(x; y) are the two relations define over two crisp sets x 2 A and y 2 B Union: R(x; y) [ S(x; y) = max(R(x; y); S(x; y)); Intersection: R(x; y) \ S(x; y) = min(R(x; y); S(x; y)); Complement: R(x; y) = 1 − R(x; y) Debasis Samanta (IIT Kharagpur) Soft Computing Applications 06.02.2018 5 / 64 Example: Operations on crisp relations Example: Suppose, R(x; y) and S(x; y) are the two relations define over two crisp sets x 2 A and y 2 B 20 1 0 03 21 0 0 03 60 0 1 07 60 1 0 07 R = 6 7 and S = 6 7 ; 40 0 0 15 40 0 1 05 0 0 0 0 0 0 0 1 Find the following: 1 R [ S 2 R \ S 3 R Debasis Samanta (IIT Kharagpur) Soft Computing Applications 06.02.2018 6 / 64 Composition of two crisp relations Given R is a relation on X,Y and S is another relation on Y ,Z . Then R ◦ S is called a composition of relation on X and Z which is defined as follows. R ◦ S = f(x; z)j(x; y) 2 R and (y; z) 2 S and 8y 2 Y g Max-Min Composition Given the two relation matrices R and S, the max-min composition is defined as T = R ◦ S ; T (x; z) = maxfminfR(x; y); S(y; z) and 8y 2 Y gg Debasis Samanta (IIT Kharagpur) Soft Computing Applications 06.02.2018 7 / 64 Composition: Composition Example: Given X = f1; 3; 5g; Y = f1; 3; 5g; R = f(x; y)jy = x + 2g; S = f(x; y)jx < yg Here, R and S is on X × Y . Thus, we have R = f(1; 3); (3; 5)g S = f(1; 3); (1; 5); (3; 5)g 1 3 5 1 3 5 1 2 0 1 0 3 1 2 0 1 1 3 R= 3 4 0 0 1 5 and S= 3 4 0 0 1 5 5 0 0 0 5 0 0 0 1 3 5 1 2 0 0 1 3 Using max-min composition R ◦ S= 3 4 0 0 0 5 5 0 0 0 Debasis Samanta (IIT Kharagpur) Soft Computing Applications 06.02.2018 8 / 64 Fuzzy relations Fuzzy relation is a fuzzy set defined on the Cartesian product of crisp set X1; X2; :::; Xn Here, n-tuples (x1; x2; :::; xn) may have varying degree of memberships within the relationship. The membership values indicate the strength of the relation between the tuples. Example: X = f typhoid, viral, cold g and Y = f running nose, high temp, shivering g The fuzzy relation R is defined as runningnose hightemperature shivering typhoid 2 0:1 0:9 0:8 3 viral 4 0:2 0:9 0:7 5 cold 0:9 0:4 0:6 Debasis Samanta (IIT Kharagpur) Soft Computing Applications 06.02.2018 9 / 64 Fuzzy Cartesian product Suppose A is a fuzzy set on the universe of discourse X with µA(x)jx 2 X B is a fuzzy set on the universe of discourse Y with µB(y)jy 2 Y Then R = A × B ⊂ X × Y ; where R has its membership function given by µR(x; y) = µA×B(x; y) = minfµA(x); µB(y)g Example : A = f(a1; 0:2); (a2; 0:7); (a3; 0:4)gand B = f(b1; 0:5); (b2; 0:6)g b1 b2 2 3 a1 0:2 0:2 R = A × B = a2 4 0:5 0:6 5 a3 0:4 0:4 Debasis Samanta (IIT Kharagpur) Soft Computing Applications 06.02.2018 10 / 64 Operations on Fuzzy relations Let R and S be two fuzzy relations on A × B. Union: µR[S(a; b) = maxfµR(a; b); µS(a; b)g Intersection: µR\S(a; b) = minfµR(a; b); µS(a; b)g Complement: µR(a; b) = 1 − µR(a; b) Composition T = R ◦ S µR◦S = maxy2Y fmin(µR(x; y); µS(y; z))g Debasis Samanta (IIT Kharagpur) Soft Computing Applications 06.02.2018 11 / 64 Operations on Fuzzy relations: Examples Example: X = (x1; x2; x3); Y = (y1; y2); Z = (z1; z2; z3); y1 y2 2 3 x1 0:5 0:1 R = x2 4 0:2 0:9 5 x3 0:8 0:6 z1 z2 z3 y 0:6 0:4 0:7 S = 1 y2 0:5 0:8 0:9 z1 z2 z3 2 3 x1 0:5 0:4 0:5 R ◦ S = x2 4 0:5 0:8 0:9 5 x3 0:6 0:6 0:7 µR◦S(x1; y1) = maxfmin(x1; y1); min(y1; z1); min(x1; y2); min(y2; z1)g = maxfmin(0:5; 0:6); min(0:1; 0:5)g = maxf0:5; 0:1g = 0:5 and so on. Debasis Samanta (IIT Kharagpur) Soft Computing Applications 06.02.2018 12 / 64 Fuzzy relation : An example Consider the following two sets P and D, which represent a set of paddy plants and a set of plant diseases. More precisely P = fP1; P2; P3; P4g a set of four varieties of paddy plants D = fD1; D2; D3; D4g of the four various diseases affecting the plants In addition to these, also consider another set S = fS1; S2; S3; S4g be the common symptoms of the diseases. Let, R be a relation on P × D, representing which plant is susceptible to which diseases, then R can be stated as D1 D2 D3 D4 2 3 P1 0:6 0:6 0:9 0:8 P2 6 0:1 0:2 0:9 0:8 7 R = 6 7 P3 4 0:9 0:3 0:4 0:8 5 P4 0:9 0:8 0:4 0:2 Debasis Samanta (IIT Kharagpur) Soft Computing Applications 06.02.2018 13 / 64 Fuzzy relation : An example Also, consider T be the another relation on D × S, which is given by S1 S2 S3 S4 2 3 D1 0:1 0:2 0:7 0:9 D2 6 1:0 1:0 0:4 0:6 7 S = 6 7 D3 4 0:0 0:0 0:5 0:9 5 D4 0:9 1:0 0:8 0:2 Obtain the association of plants with the different symptoms of the disease using max-min composition. Hint: Find R ◦ T , and verify that S1 S2 S3 S4 2 3 P1 0:8 0:8 0:8 0:9 P2 6 0:8 0:8 0:8 0:9 7 R ◦ S = 6 7 P3 4 0:8 0:8 0:8 0:9 5 P4 0:8 0:8 0:7 0:9 Debasis Samanta (IIT Kharagpur) Soft Computing Applications 06.02.2018 14 / 64 Fuzzy relation : Another example Let, R = x is relevant to y and S = y is relevant to z be two fuzzy relations defined on X × Y and Y × Z, respectively, where X = f1; 2; 3g ,Y = fα; β; γ; δg and Z = fa; bg. Assume that R and S can be expressed with the following relation matrices : α β γ δ 1 2 0:1 0:3 0:5 0:7 3 R = 2 4 0:4 0:2 0:8 0:9 5 and 3 0:6 0:8 0:3 0:2 a b α 2 0:9 0:1 3 β 6 0:2 0:3 7 S = 6 7 γ 4 0:5 0:6 5 δ 0:7 0:2 Debasis Samanta (IIT Kharagpur) Soft Computing Applications 06.02.2018 15 / 64 Fuzzy relation : Another example Now, we want to find R ◦ S, which can be interpreted as a derived fuzzy relation x is relevant to z. Suppose, we are only interested in the degree of relevance between 2 2 X and a 2 Z .
Details
-
File Typepdf
-
Upload Time-
-
Content LanguagesEnglish
-
Upload UserAnonymous/Not logged-in
-
File Pages64 Page
-
File Size-