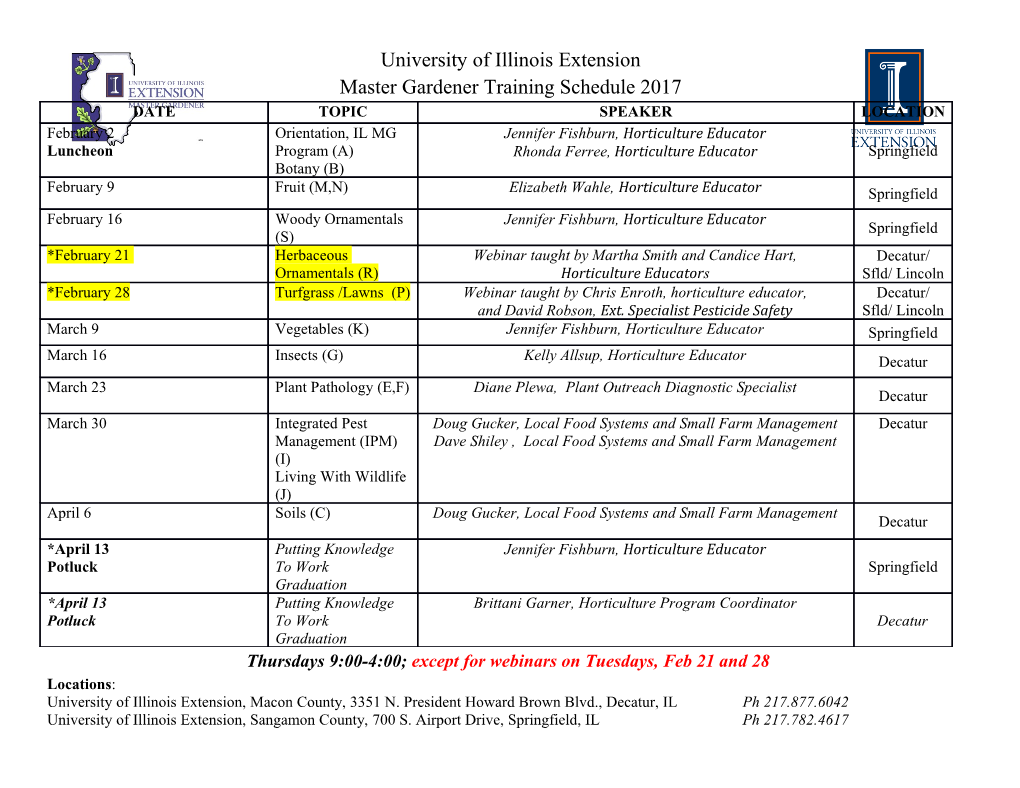
Stat 694 Lecture Notes -- Thurs., 3/2/06 Lecture – Thurs., 3/2/06 Today • Finish seeds example – Bayesian logistic regression • Overdispersed generalized linear models • PHDCN example – Bayesian hierarchical logistic regression model • Bayesian generalized linear model for count data – Poisson (log- linear) regression model • Salmonella example 1 Bayesian Generalized Linear Model GENERALIZED LINEAR MODEL Idea: extend the idea of linear modeling to cases where either 1. the relationship between X and E(y|X ) is not linear 2. assumption of a normal sampling distribution is inappropriate 2 1 Stat 694 Lecture Notes -- Thurs., 3/2/06 Bayesian Generalized Linear Model SEEDS EXAMPLE • We want to look at the relationship between germination and both seed type (73 or 75) and root extract (bean or cucumber). LOGISTIC REGRESSION MODEL p(r i|p i,n i) = Bin(p i,n i), where logit(p i) = α0+α1 xi1 + α2xi2 +α12 xi1 xi2 3 Bayesian Generalized Linear Model BAYESIAN LOGISTIC REGRESSION MODEL • Likelihood • Prior on the αs 4 2 Stat 694 Lecture Notes -- Thurs., 3/2/06 Bayesian Generalized Linear Model Definition We say that a generalized linear model is overdispersed if the model allows the possibility of variation beyond that of the assumed sampling distribution. Bayesian Overdispersed (Random Effects) Logistic Regression Model • p(r i|p i,n i) = Bin(p i,n i), where logit(p i) = α0+α1 x1 + α2x2 +α12 x1x2+b i 2 2 • p(b i|σ ) = N(0, σ ) 5 Bayesian Generalized Linear Model PROJECT ON HUMAN DEVELOPMENT (PHDCN) EXAMPLE 6 3 Stat 694 Lecture Notes -- Thurs., 3/2/06 Bayesian Generalized Linear Model RESPONSE VARIABLE • BMI INDIVIDAL COVARIATES • age1 • famsize • sescomp • gender • fams1 • latino • black 7 Bayesian Generalized Linear Model NC COVARIATES • pclnpov • pcstab3 • comstrt8 8 4 Stat 694 Lecture Notes -- Thurs., 3/2/06 Bayesian Generalized Linear Model HIERARCHICAL LOGISTIC REGRESSION MODEL For the ith student in the jth neighborhood, (I) • p(Y i,j |p i,j ) = Bern(p i,j ), where logit(p i,j ) = Xi,j β + µj (N) • µj = αj + Zjβ • p( αj) = • p( β(I))= • p( β(N))= 9 Bayesian Generalized Linear Model POSTERIOR SUMMARIES Variable Post. Mean (95% Credible Interval) intrcpt -4.106 (-5.112,-2.993) age1 0.149 (0.077,0.21) famsize -0.008 (-0.091,0.069) sescomp -0.128 (-0.257,-0.012) gender 0.123 (-0.209,0.445) fams1 -0.004 (-0.356,0.375) latino 0.418 (-0.052,0.931) black 0.792 (0.294,1.374) 10 5 Stat 694 Lecture Notes -- Thurs., 3/2/06 Bayesian Generalized Linear Model POSTERIOR SUMMARIES Variable Post. Mean (95% Credible Interval) pclnpov 0.017 (-0.171,0.212) pcstab3 0.193 (-0.035,0.415) cmstrt8 0.099 (-0.124,0.325) 11 Bayesian Generalized Linear Model POSTERIOR SUMMARIES 12 6 Stat 694 Lecture Notes -- Thurs., 3/2/06 Bayesian Generalized Linear Model GENERALIZED LINEAR MODEL – COUNT DATA Assumption: SALMONELLA EXAMPLE (Breslow, 1984) 13 Bayesian Generalized Linear Model OVERDISPERSED POISSON REGRESSION MODEL 14 7.
Details
-
File Typepdf
-
Upload Time-
-
Content LanguagesEnglish
-
Upload UserAnonymous/Not logged-in
-
File Pages7 Page
-
File Size-