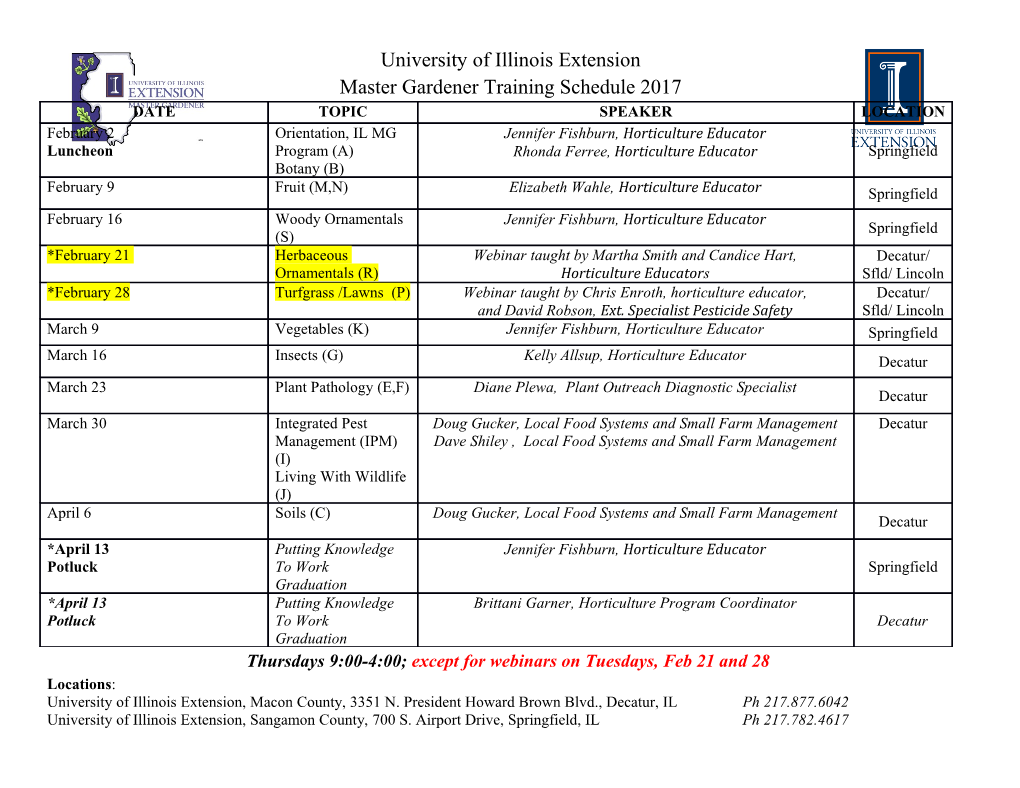
[P4] Agonists of G protein coupled-odorant receptors are predicted from chemical features Caroline Bushdid1, Claire Alice de March2, Sébastien Fiorucci 1, Hiroaki Matsunami2,3, Jérôme Golebiowski1,4 1 ChemoSim, Institut de Chimie de Nice, Université Nice Sophia Antipolis, 28 avenue Valrose, 06108, Nice Cedex 2, France. 2 Department of Molecular Genetics and Microbiology, Duke University Medical Center, Durham, NC 27710, USA 3 Department of neurobiology and Duke Institute for Brain Sciences, Duke University, Durham, NC 27710, USA 4 Department of Brain & Cognitive Sciences, DGIST, Daegu, Republic of Korea G protein-coupled receptors (GPCRs), the targets of nearly 30% of all modern therapeutic drugs, are seven transmembrane proteins converting extracellular stimuli into intracellular signals in a wide variety of cell types.[1] In the human nose, odor detection by olfactory sensory neurons (OSNs) depends on a large family of G protein-coupled odorant receptors (ORs) which represent 4% of our proteome.[2] Recent studies also report the presence of such receptors in many non-olfactory tissues.[3] Understanding the characteristics of the compounds these receptors get activated by becomes increasingly relevant.[4] Here, the activity of 258 chemicals on the human G protein-coupled odorant receptor (OR)51E1, also known as prostate-specific G protein-coupled receptor 2 (PSGR2) [5] was virtually screened by machine learning using 4884 chemical descriptors as input. A systematic control by functional in vitro assays revealed that a support vector machine algorithm accurately predicted the activity of a screened library. It allowed identifying two novel agonists in vitro for OR51E1. The transferability of the protocol was assessed on OR1A1, OR2W1, and MOR256-3 odorant receptors and in each case, novel agonists were identified with a hit rate ranging from 39% to 50%. We further show how ligands’ efficacy is encoded into residues within OR51E1 cavity using a molecular modelling protocol. Our approach allows widening the chemical spaces associated with odorant receptors.[6] This machine learning protocol based on chemical features thus represents an efficient tool for screening ligands for G protein-coupled odorant receptors that modulate non-olfactory functions, or upon combinatorial activation, give rise to our sense of smell. Bibliography: [1] Rask-Andersen M, Almén M.S., Schiöth H.B. Nat. Rev. Drug Discov. 10 (2011) 579-590 [2] Niimura Y. Curr. Genomics 13 (2012) 103-114 [3] Kang N., Koo J. BMB Rep. 45 (2012) 612-622 [4] Wacker D., Stevens R.C., Roth B.L. Cell 170 (2017) 414-427 [5] Flegel C., Manteniotis S., Osthold S., Hatt H. GIsselmann G. PLoS One 8 (2013) e55368 [6] Bushdid C., de March C.A., Fiorucci S., Matsunami H., Golebiowski J. J. Phys. Chem. Lett. (2018) accepted 42 .
Details
-
File Typepdf
-
Upload Time-
-
Content LanguagesEnglish
-
Upload UserAnonymous/Not logged-in
-
File Pages1 Page
-
File Size-