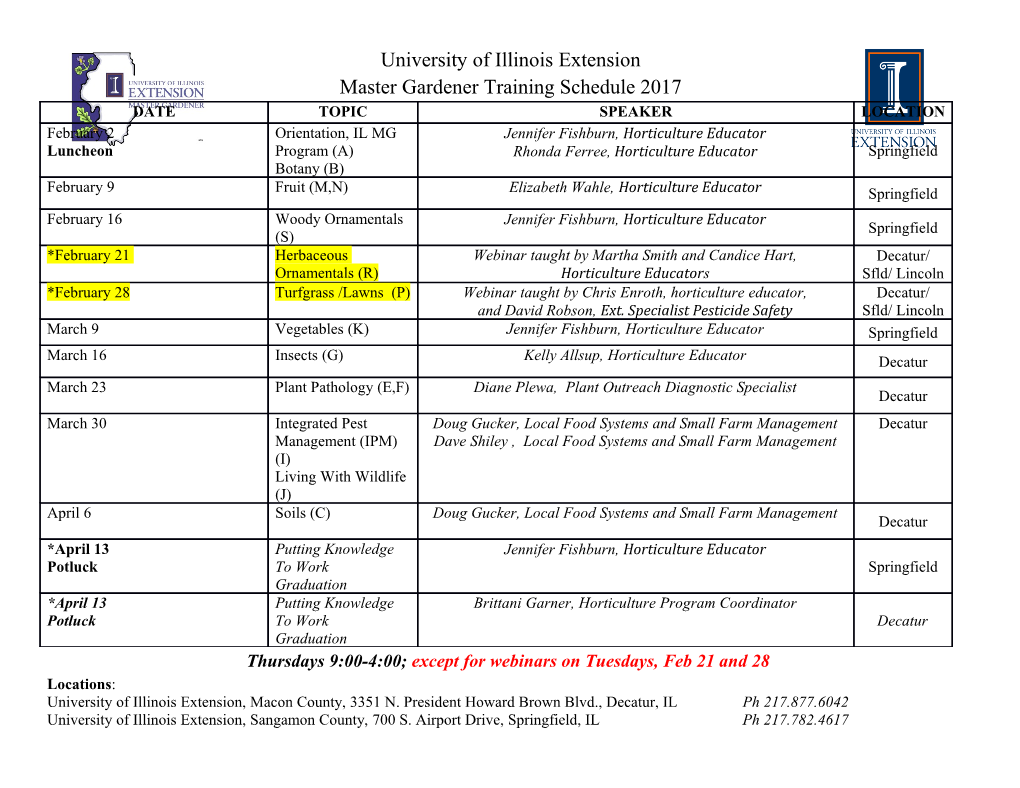
Supplementary Online Content Teschendorff AE, Yang Z, Wong A, et al. Correlation of smoking-associated DNA methylation changes in buccal cells with DNA methylation changes in epithelial cancer. JAMA Oncol. Published online May 14, 2015. doi:10.1001/jamaoncol.2015.1053 eMethods eFigure 1. Flowchart Figure eFigure 2. Correlation between smoking pack years and the time of last quit before sample collection eFigure 3. DNA methylation reversal for AHRR eFigure 4. Singular Value Decomposition analysis of the discovery set DNA methylation data matrix of 400 buccal samples and 479,491 CpGs eFigure 5. Correction for cellular heterogeneity using RefFreeEWAS in the discovery buccal sample set (n=400) eFigure 6. Comparison of Buccal and Whole Blood smoking DNA methylation signatures eFigure 7. Linear correlation between smoking index and smoking pack years eFigure 8. The smoking index is aggravated in cancer eFigure 9. The smoking index across normal/cancer sets (part-1), as evaluated by restricting to four different CpG subsets from the full 1501 smoking-associated DNAme signature eFigure 10. The smoking index across normal/cancer sets (part-2), as evaluated by restricting to four different CpG subsets from the full 1501 smoking-associated DNAme signature eFigure 11. The smoking index as evaluated in endometrial carcinogenesis eFigure 12. The smoking index evaluated in a series of 152 cytologically normal cervical smear samples eFigure 13. Functional significance of smoking DNAme signature eFigure 14. The smoking index from three GSEA-enriched DNAme subsignatures in the normal cancer data sets (part-1) eFigure 15. The smoking index from three GSEA-enriched DNAme subsignatures in the normal cancer data sets (part-2) eFigure 16. Prediction of smoking status using DNA methylation profiles based on an elastic net classifier eFigure 17. Prediction of smoking status from buccal DNA methylation profiles using an elastic net classifier, and using a different training/test set partition of the 790 buccal samples eTable 1. Statistics of association of the 1501 smoking-associated CpGs eTable 2. RefFreeEWAS selected CpGs eTable 3. Gene Set Enrichment Analysis summary table of the hypermethylated smoking-associated CpGs eTable 4. Gene Set Enrichment Analysis summary table of the hypomethylated smoking-associated CpGs eTable 5. Enrichment Analysis Table of Transcription Factor (TF) Binding Sites eTable 6. Smoking associated fold-expression changes in non-tumour lung tissue of smoking associated CpGs eReferences This supplementary material has been provided by the authors to give readers additional information about their work. © 2015 American Medical Association. All rights reserved. Downloaded From: https://jamanetwork.com/ on 10/02/2021 eMethods Data Set and Ethical Approval: We analysed buccal cells from 790 women enrolled in the MRC National Survey of Health and Development (NSHD) study, a birth cohort study of men and women all born in Britain in March 1946 [1]. These 790 women were selected from those who provided a buccal and blood sample at the age of 53 in 1999, who had not previously developed any cancer, and who had complete information on epidemiological variables of interest and follow up. For 152 of these women we also analysed a matched blood sample. The study was approved by the Central Manchester Ethics Committee (07/H1008/168). Experimental Protocol for DNA methylation data and data availability: DNA from 790 buccal and 152 blood samples was extracted at Gen-Probe (www.gen-probe.com). Methylation analysis was performed using the Illumina Infinium Human Methylation450 BeadChip array [2]. The NSHD data are made available to researchers who submit data requests to [email protected]; see full policy documents at http://www.nshd.mrc.ac.uk/data.aspx. Managed access is in place for this 69 year old study to ensure that use of the data are within the bounds of consent given previously by participants, and to safeguard any potential threat to anonymity since the participants are all born in the same week. Quality Control and Normalization Analysis: Quality control and intra-sample normalization was performed on each of the 790 buccal and then separately on the 152 matched whole blood samples. In each case, raw .idat data files were processed using the minfi package [3], using the Illumina definition of beta-values and extracting P-values of detection for each sample. The Illumina methylation beta-value of a specific CpG site is calculated from the intensity of the methylated (M) and unmethylated (U) alleles, as the ratio of fluorescent signals β=Max(M,0)/[Max(M,0)+Max(U,0)+100]. On this scale, 0<β<1, with β values close to 1 (0) indicating 100% methylation (no methylation). Probes with more than 5% values not passing the detection P-value threshold were removed from further analysis, and the rest of NA’s were imputed using the k-nearest neighbors imputation procedure [4]. In the case of the 790 sample set, this resulted in 479,491 probes. To correct for the well-known bias of type-2 probes, we used the SWAN package [5]. To check robustness of this correction procedure, we verified that results were largely unchanged using BMIQ [6]. This completed the intra-sample normalization. Next, the 790 unmatched buccal samples were divided into two sets, one set defining a discovery set of 400 samples, with the remaining 390 defining a replication set. Sample selection was performed randomly (large sample size ensured that proportions of epidemiological factors, e.g. never-smokers, ex-smokers and current smokers, was similar between the two sets -see Table-1). To assess inter-sample variability within the discovery set, we first centered the intra-sample normalized beta-valued data matrix so that each probe had a mean zero across all samples. We then used Singular Value Decomposition (SVD) on this centered matrix to identify the components of maximal variation [7]. Random Matrix Theory was used to predict the number of significant components of variation [8]. In order to assess the relative contributions of biological and technical variables to data variability, significant components of variation were correlated to phenotypic and technical factors and results rendered in a P-value heatmap, a procedure previously implemented by us [7,9]. The SVD analysis revealed that the top component of variation correlated with Smoking Pack Years (SPY), an epidemiological indicator of an individual’s smoking history. Technical factors, notably, beadchip effects and variations in bisulfite conversion (BSC) efficiency were associated with the 2nd largest component of variation. Similar results were obtained in the replication cohort of 390 buccal samples. Supervised Analysis: Using the discovery set of 400 buccal samples, we next performed linear regressions between smoking pack years (SPY) and the beta methylation profiles. In detail, for each CpG, we ran a © 2015 American Medical Association. All rights reserved. Downloaded From: https://jamanetwork.com/ on 10/02/2021 multivariate linear regression using the estimated bisulfite conversion (BSC) efficiency (BSC) as a covariate to ensure that results would not be confounded by variations in BSC efficiency. Because there were only a maximum of 12 samples per beadchip, robustness against beadchip effects was tested at the very end of our analyses, by repeating all analyses with a different choice of discovery and replication sets. Specifically, instead of randomly picking samples, we randomly picked beadchips, thus ensuring that all samples from the discovery set were done on one set of beadchips, and all samples from the replication set done on a mutually exclusive set of chips. CpGs from the supervised regressions in the discovery set were ranked according to P-value, histograms of P-values was generated and the False Discovery Rate (FDR) estimated using the q-value procedure [10]. Given the observed strong association, we used a very stringent Bonferroni threshold (1.04e-7=0.05/479,491) to define smoking associated differentially methylated CpGs (DMCs). A total of 1501 CpGs passed this threshold, defining our buccal DNA methylation signature. Linear regression with adjustment for BSC efficiency were also used in the replication set, i.e. the 390 buccal set, to derive t-statistics of association between probe’s DNA methylation profiles and smoking pack years. In the case of the matched 152 whole blood set, we observed that the histogram of P-values exhibited a shape indicating the presence of a confounding factor [11]. SVD analysis over the 152 whole blood set revealed that the top component of variation did not correlate with any known biological, epidemiological or technical factor. Hence, for this data set, we applied Independent Surrogate Variable Analysis (ISVA) [8], to derive statistics of association and P-values, resulting in an improved FDR (q-values were used as FDR estimates). After application of ISVA, the resulting histogram of P-values exhibited a shape that was consistent with statistical theory. The fact that the top ranked CpGs derived from ISVA mapped to genes previously reported to undergo significant DNAme changes in independent blood EWAS (e.g. genes like AHRR, CYP1A1, PTK2, GFI1) attests to the quality of our normalized blood DNAme data. Correction for cellular heterogeneity: Although confounding variation by cell-type has been known to inflate signals in blood tissue [12], buccal tissue is more homogeneous and no deconvolution method has yet been properly validated on this type of tissue [13]. Nevertheless, we applied a reference-free deconvolution algorithm [13], which resulted in 897 of the 1501 CpGs retaining significance at a false discovery rate (FDR) threshold of 0.05 (FDR<0.05). This supports the view that putative changes in sample composition only has a moderate effect in buccal cells. Because the algorithm has been not been extensively tested on a tissue like buccal, results in this manuscript are based on the full set of 1501 CpGs.
Details
-
File Typepdf
-
Upload Time-
-
Content LanguagesEnglish
-
Upload UserAnonymous/Not logged-in
-
File Pages212 Page
-
File Size-