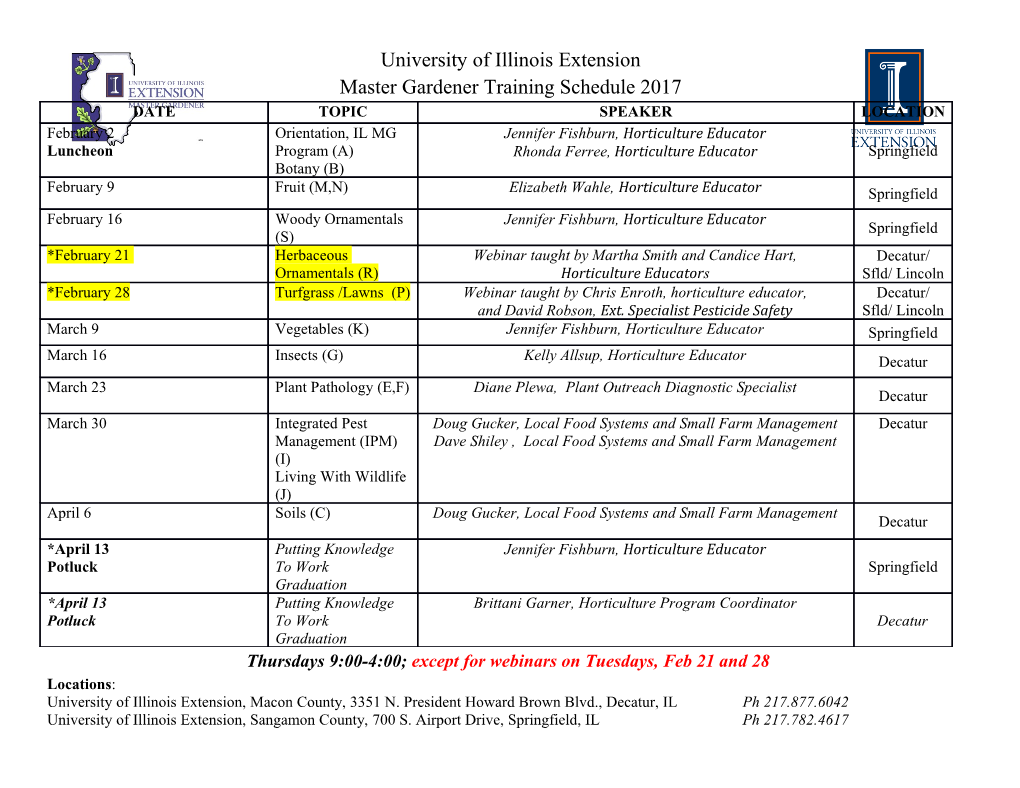
Wednesday 24th October 09:00 - 18:30 Location: 40.033 Nursing Room available 13:00 - 17:30 Location: 40.035 S. Graus Green City Hackathon Thursday 25th October 08:00 - 18:30 Location: 30.SV01 HALL Arrival and Registration 08:30 - 09:00 BCN Supercomputing Centre tour 09:00 - 20:30 Location: 40.033 Nursing Room available 09:00 - 12:00 Location: 40.035 S. Graus Green City Hackathon 12:00 - 13:00 Lunch (on your own) 13:00 - 13:30 BCN Supercomputing Centre tour 13:30 - 14:00 BCN Supercomputing Centre tour 13:00 - 16:30 Location: 40.S03 Big Data Processing for Social Science: An Introduction to Apache Spark 13:00 - 16:30 Location: 40.S14 Adaptive Survey Design 13:00 - 16:30 Location: 40.047A Biases and Their Consequences: Learning From the Total Survey Error Framework 13:00 - 16:30 Location: 40.047C Introduction to Computational Text Analysis 17:00 - 18:45 Location: 30.S01 Auditori Welcome and Opening Keynotes by Julia Lane (Professor, Wagner School of Public Policy at New York University; Center for Urban Science and Progress; Provostial Fellow) and Tom Smith (Managing Director, Data Science Campus of the Office for National Statistics, UK) Download Julia Lane's Keynote Download Tom Smith's Keynote 18:45 - 20:30 Welcome Reception at UPF (Indoor Courtyard, Roger de Llúria building). Weather permitting, the reception will be held outside (Outdoor Courtyard, Jaume I building) Friday 26th October 08:00 - 18:30 Location: 30.SV01 HALL Registration and Information Desk Fri 26th October, 08:30 - 17:30 Location: 30.S02 S. Expo Session: Posters 1 (actively presented from 11.30 to 13.00) Dr Antje Kirchner (RTI) These posters are the result of the Barcelona Dades Obertes Data Challenge organized by the city of Barcelona. For more information on the institutions and the data challenge please see: ● http://opendata-ajuntament.barcelona.cat/ca/esdeveniment-projectes ● http://opendata-ajuntament.barcelona.cat/ca/centres-participants Poster Title High School Investigating Complaints in Gracia Institut Vila de Gràcia Social Cohesion and Type of Neighborhood Institut Ferran Tallada Free WI-FI Points in Barcelona Institut Juan Manuel Zafra Access to Housing in Barcelona Institut Joan Brossa A Study of Traffic Accidents in Barcelona Institut J. Serrat i Bonastre WI-FI Points Institut Josep Comas i Solà Count Regression Modelling on Number of Migrants in Households Tsedeke Lambore Gemecho (PhD Student) - Presenting Author Ayele Taye Goshu (Associate Professor of Statistics ) The main objective of this study is to identify determinants of the number of international migrants in a household, and to compare regression models for count response. A total of 2288 data are collected from sixteen randomly sampled districts in Hadiya and Kembata-Tembaro zonal areas, Southern Ethiopia. The Poisson mixed models, as special cases of the generalized linear mixed model, is explored to determine effects of the predictors: age of household head, farm land size, and household size. Two ethnicities, Hadiya and Kembata, are included in the final model as dummy variables. Stepwise variable selection has indentified four predictors: age of head, farm land size, family size and dummy variable ethnic2 (0=other, 1=Kembata). These predictors are significant at 5% significance level with count response number of migrant. The Poisson mixed model consisting of the four predictors with random effects districts. Area specific random effects are significant with variance of about 0.5105 and standard deviation of 0.7145. The results show that the number of migrant increases with heads age, family size and farm land size. In conclusion, there is significantly high number of international migration per household in the area. Age of household head, family size, and farm land size are determinants that increase the number of international migrant in households. Community based intervention is needed so as to monitor and regulate the international migration for the benefits of the society. Testing Analytical Methods Related With the Unstructured Data Analysis From Perspective of ‘Data Scientists and Methodologists’ Piotr Tarka (Poznan University of Economics and Business, Department of Market Research) - Presenting Author In the market, opinion, survey, and social science researches, there arises a growing amount of data that in majority is based on unstructured formats derived from: customer feedbacks, social media conversations, blogs, news, articles, voice, and photos. Such data continues to grow in volume, variety, velocity, but also in the overall value. For the data scientists and classical methodologists, this trend offers new opportunities but also challenges. There has even been evidenced a slow shift in the analytical approaches that some data experts in practice use to derive values, in particular from the unstructured data analysis. In this presentation, having based on the conducted empirical research, we try to diagnose to what extent and which respective groups of experts relate, in their analytical work, to: textual, audio (spoken language), and picture data formats, assuming there may appear differences in context of intensity of application of these formats in practical data analysis. In article, by investigating experts' experience, we compare their opinions regarding unstructured data methods by implementing analytical approach based on the confirmatory factor analysis as well as multiple group analysis. With this assumption in mind, we constructed CFA model which allowed us testing the equivalence level of the data experts' views. The data was collected in the course of international online survey (through the agency of LinkedIn social network) among the data experts with three various educational backgrounds as: 1) economics/business, 2) sociology/psychology, and 3) mathematics/statistics/computer science. A Joint Modelling Approach in SAS to Assess Association Between Adult and Child HIV Infections in Kenya Elvis Muchene (University of Nairobi) - Presenting Author Recent studies have adopted a joint modelling approach as a more stout technique in studying outcomes of interest simultaneously, especially when the interest is in the association between two dependent variables. This has been necessitated by the fact that modelling such outcomes separately often leads to biased inferences due to existing possible correlations, especially in medical studies. This paper demonstrates the application of linear mixed modelling approach using SAS analysis software to evaluate the correlation between adult and child HIV infections for each county in Kenya, while adjusting for several predictors of interest. Using HIV data extracted from the Kenya open data website for the year 2014, we visualize in each county the HIV prevalence on the Kenyan map. High infection incidences are observed for counties located in Nyanza province. We further fit a joint model for the two outcomes of interest using the linear mixed models approach to capture possible correlation between the two outcomes for each county. Results indicate that there is a correlation between infections in adults and children. Further, there is a significant effect of ART coverage, adults and children in need of ART and number of people undergoing testing voluntarily. Researchers or students who have little understanding in application of linear mixed models, both theoretical understanding and practical analysis in SAS, as well as application on real datasets, will find this article useful. Findings from this article would interest the health sector, practitioners and other institutions working in HIV related interventions. Comparison of Artificial Neural Networks and Generalized Linear Models for NBA Outcomes Shan Wang (Northeastern Illinois University) - Presenting Author William Johnson (Northeastern Illinois University) Artificial neural networks are statistical learning models, inspired by biological neural networks, that are used in machine learning. It is a nonlinear regression method that provides alternative ways to logistic modeling, or more generally, generalized linear modeling. Comparing to the traditional regression method, such as the generalized linear model, the well-known advantages of ANN include the ability to detect more complex relationships between dependent and independent variables, fewer requirements of statistical training and the ability to analyze big data. This article presents an overview of the artificial neural networks method and the generalized linear modeling method and compares the predictive accuracy and computational burden using the National Basketball Association dataset from 2010 to 2017. From Data Points to Data Dan: Combining Log Analysis: Survey Analysis and Interviews to Segment Google Analytics Customers Laura Eidem (N/A) - Presenting Author Yinni Guo (N/A) Sundar Sdorairaj (N/A) Google Analytics has a wide user base, from hobbyist bloggers to employees of Fortune 100 corporations. In order to better understand our users, and to get more precision around the proportion of each user type that make up our customer base, we embarked on a customer segmentation project. This long-term research project used both qualitative and quantitative methods to scope and define customer “use cases,” or particular tasks that directed the front-end interactions of a user’s session. Our quantitative approach consisted of collecting all front-end user interactions, and performing Latent Dirichlet Analysis to arrive at groupings of 25 use cases, as well as conducting a survey to investigate
Details
-
File Typepdf
-
Upload Time-
-
Content LanguagesEnglish
-
Upload UserAnonymous/Not logged-in
-
File Pages101 Page
-
File Size-