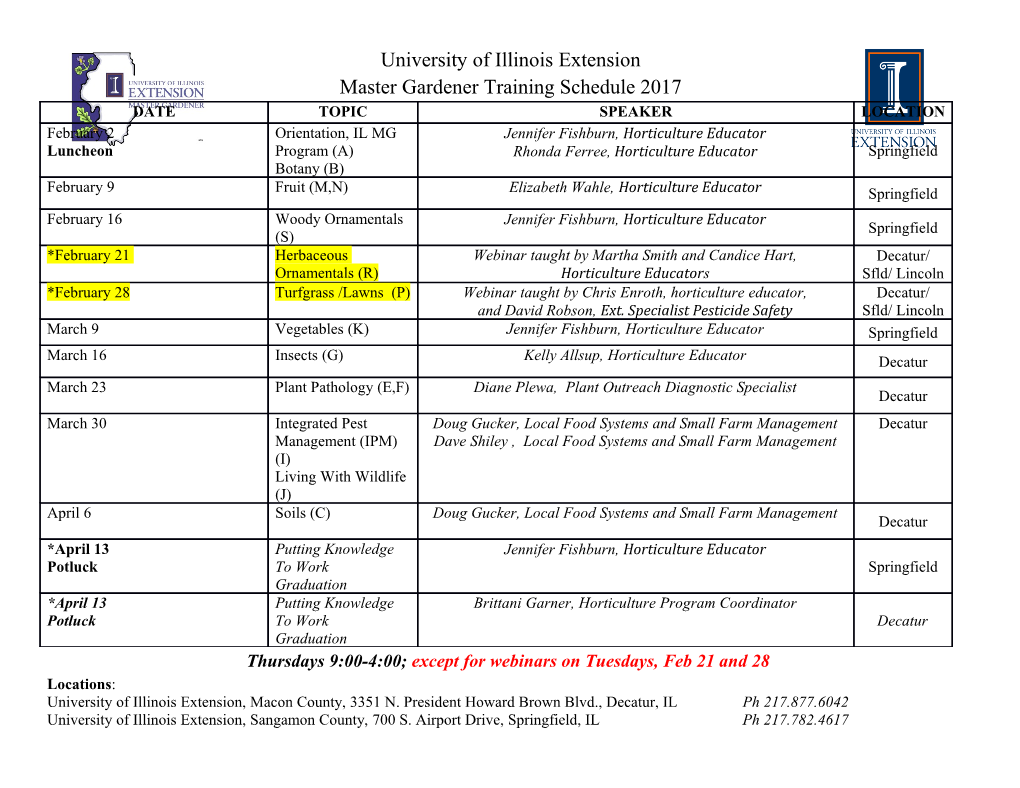
Effectiveness of Machine Learning for Intrusion Detection Systems. Heuristic-based Network Intrusion Detection System over Supervised Learning Valiente Sanchez, Joel Curs 2019-2020 Director: Vanesa Daza GRAU EN ENGINYERIA INFORMÀTICA Treball de Fi de Grau ACKNOWLEDGEMENTS To my family, for supporting me as much as they can for getting this university degree and completing this project. To my supervisor, Vanesa Daza, for encouraging me to develop this project. All the advice and corrections provided were necessary for completing this project. To all of you, thanks for providing me all the love and opportunities I needed. iii ABSTRACT Network Intrusion Detection Systems (NIDS) are software applications monitoring a network and the systems using this network for detecting malicious activities. These activities are reported to the administrator in the form of alarms. No further actions are taken to prevent these attacks, the creation of these alarms is the desired output of any NIDS. The administrator, using these alarms, realise what attacks are being performed. In front of successful attacks the source of the problem (vulnerability) is described by the alarms and, therefore, the administrator knows what needs to be fixed indeed. It is currently an expanding area and many companies provide software compatible with most used OS (Windows, Linux and Mac OS), for instance, Snort is the most used NIDS worldwide (owned and maintained by Cisco from 2013) which supports both Windows and Linux. Most used NIDSs are rule-based: administrators defines rules that match corresponding attacks. These rules can be provided by some NIDS vendors to detect well known attacks. The amount of rules is considerable and usually the management of them becomes a full-time work. As a result, researches are currently developing new NIDS using the power of Machine Learning in order to automatize this task. However, open source and free options are scarce which leads to performing this final degree project. RESUM Network Intrusion Detection Systems (NIDS) són aplicacions software monitorant una xarxa i els sistemes utilitzant-la per detectar activitats malicioses. Aquestes activitats són enviades a l’administrador en forma d’alarmes. Cap acció extra es pren per evitar aquests atacs, la creació d’aquestes alarmes és la resposta esperada de qualsevol NIDS. L’administrador, utilitzant les alarmes, s’adona de quins atacs s’estan executant. Davant d’atacs realitzats amb èxit, la font del problema (vulnerabilitat) es descriu en les alarmes i, per tant, l’administrador comprèn què necessita ser fixat. Actualment és una àrea en expansió i diferents empreses proporcionen software compatible amb els SO més utilitzats (Windows, Linux i Mac OS), per exemple, Snort és el NIDS més utilitzat internacionalment (apropiat i mantingut per Cisco des del 2013). el qual suporta Windows i Linux. Els NIDS més utilitzats es basen en regles: administrador defineixen regles les quals coincideixen amb els atacs corresponents. Aquestes regles poden ser proporcionades per alguns venedors de NIDS per detectar atacs ben coneguts. La quantitat de regles és considerable i sovint la seva gestió es converteix en treball a temps complet. Com a resultat, els investigadors estan actualment desenvolupant nous NIDS utilitzant la potència del Machine Learning per automatitzar aquesta tasca. Malgrat això, les opcions open source i gratuïtes són escasses la qual cosa va donar lloc a la creació d’aquest treball final de grau. v PROLOG Cybersecurity concerns with protecting users information against attackers. Cybercriminals are constantly trying to steal as much information as possible for malicious purposes. Normally, the targeted victims are enterprises offering services for users. For protecting their systems, enterprises make use of Network Intrusion Detection Systems. These systems are responsible for detecting and notifying enterprises that cyberattacks are being performed. Normally, these systems detect attacks by means of rules. Thus, these systems are known as rule-based Network Intrusion Detection Systems. Alerts are triggered for attacks matching rules defined by administrators. Moreover, administrators spend a lot of hours maintaining the rules used to detect attacks. It becomes a full-time job in most enterprises as each day new attacks emerge. As a result, a new type of those systems detecting and alerting from attacks appears for solving the management problem. The name of this new type is heuristic-based Network Intrusion Detection Systems. These systems does not require administrators procedures as it is managed by itself. In other words, these systems are intelligent. Machine Learning, a subset of Artificial intelligence, is used in these systems. The systems are somehow trained for detecting if attacks are being performed. No rules are needed and administrators are not involved in it. Researchers are improving these intelligent systems for detecting as many attacks as possible. Also, as new attacks constantly appear, researchers are trying to supply enough intelligence to systems for learning how to detect new attacks. Most of the Network Intrusion Detection Systems used worldwide are not intelligent. Administrators are involved in the process as rules are used. This systems are tools installed in machines. The tools are publicly available on the Internet in most cases. Users and enterprises can use them for free. These tools are known as open-source as its code is also available. However, heuristic-based detection systems are less common that rule-based. There are a few tools available on the Internet for free but enterprises are not using them indeed. openNIDS is a heuristic-based Network Intrusion Detection System provided for solving this problem. It is completely free to use and the code is publicly available as well. The purpose of this tool is bringing users and enterprises a tool using Machine Learning for detecting attacks. Moreover, the results are shown in a Graphical User Interface for making them understandable by a large audience. Systems intelligence provided by Machine Learning algorithms needs data from where to lean. Dataset is the technical name given to data used by these algorithms. Security data from enterprises is very scarce on the Internet or too old. This is a problem for developing that kind of systems as the correct behaviour of them strictly depends on the data used. openNIDS is not worried about this problem as it is trained with open-source data. vii CONTENTS ABSTRACT............................................................................................ vii PROLOG................................................................................................. ix FIGURES LIST....................................................................................... xii TABLES LIST........................................................................................ xiii 1. NIDS.................................................................................................... 1 1.1. Background...................................................................................... 1 a) Anatomy.......................................................................................... 1 b) Location.......................................................................................... 2 1.2. Types of NIDS................................................................................. 4 a) Rule-based....................................................................................... 4 b) Heuristic-based............................................................................... 5 1.3 State-of-the-art software.................................................................... 7 a) Snort................................................................................................ 7 b) Suricata........................................................................................... 9 c) Zeek................................................................................................. 11 d) Hogzilla........................................................................................... 12 e) Closing comments…....................................................................... 15 2. SOFTWARE DEVELOPED............................................................... 17 2.1. Machine Learning............................................................................ 18 a) Random forest................................................................................. 18 b) Dataset............................................................................................. 22 c) HPC................................................................................................. 30 d) ML models...................................................................................... 32 e) Evaluation metrics........................................................................... 33 2.2. GUI................................................................................................... 40 a) Design............................................................................................. 40 b) Implementation............................................................................... 42 c) Real-time demo............................................................................... 45 2.3. License............................................................................................. 46 3. CONCLUSIONS................................................................................
Details
-
File Typepdf
-
Upload Time-
-
Content LanguagesEnglish
-
Upload UserAnonymous/Not logged-in
-
File Pages71 Page
-
File Size-