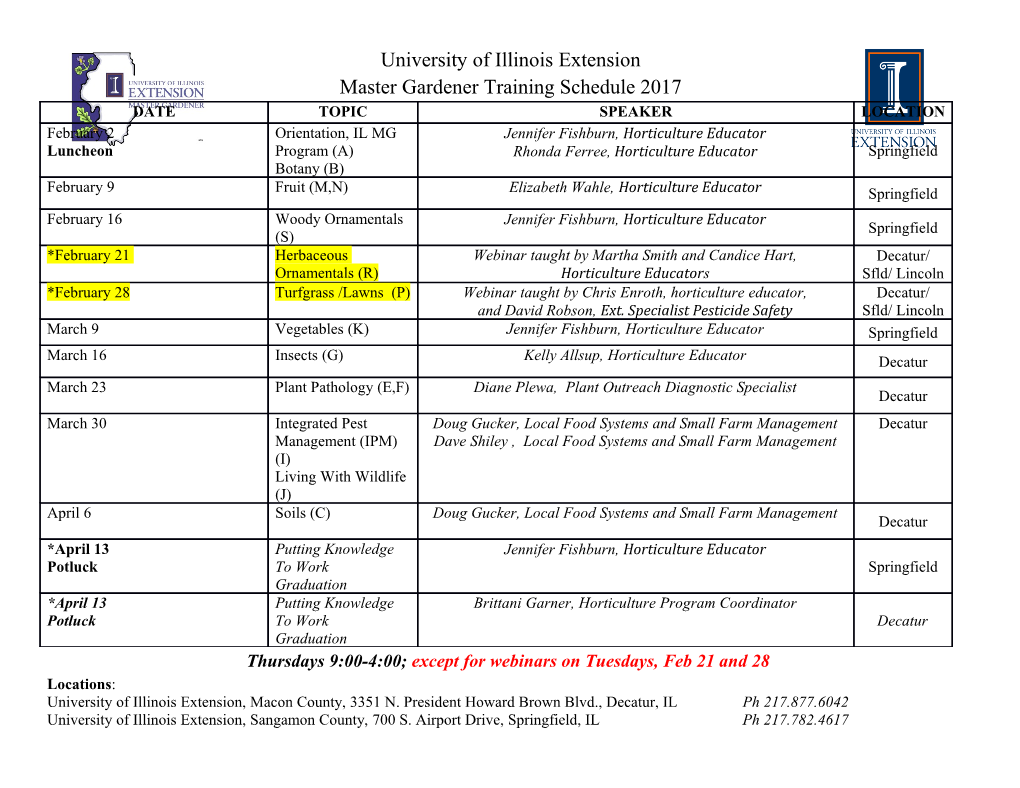
What is Compressed Sensing? Main Results Construction of Measurement Matrices Some Topics Not Covered An Overview of Compressed sensing M. Vidyasagar FRS Cecil & Ida Green Chair, The University of Texas at Dallas [email protected], www.utdallas.edu/∼m.vidyasagar Distinguished Professor, IIT Hyderabad [email protected] Ba;a;ga;va;tua;l .=+a;ma mUa; a;tRa and Ba;a;ga;va;tua;l Za;a:=+d;a;}ba Memorial Lecture University of Hyderabad, 16 March 2015 M. Vidyasagar FRS Overview of Compressed Sensing What is Compressed Sensing? Main Results Construction of Measurement Matrices Some Topics Not Covered Outline 1 What is Compressed Sensing? 2 Main Results 3 Construction of Measurement Matrices Deterministic Approaches Probabilistic Approaches A Case Study 4 Some Topics Not Covered M. Vidyasagar FRS Overview of Compressed Sensing What is Compressed Sensing? Main Results Construction of Measurement Matrices Some Topics Not Covered Outline 1 What is Compressed Sensing? 2 Main Results 3 Construction of Measurement Matrices Deterministic Approaches Probabilistic Approaches A Case Study 4 Some Topics Not Covered M. Vidyasagar FRS Overview of Compressed Sensing What is Compressed Sensing? Main Results Construction of Measurement Matrices Some Topics Not Covered Compressed Sensing: Basic Problem Formulation n Suppose x 2 R is known to be k-sparse, where k n. That is, jsupp(x)j ≤ k n, where supp denotes the support of a vector. However, it is not known which k components are nonzero. Can we recover x exactly by taking m n linear measurements of x? M. Vidyasagar FRS Overview of Compressed Sensing What is Compressed Sensing? Main Results Construction of Measurement Matrices Some Topics Not Covered Precise Problem Statement: Basic Define the set of k-sparse vectors n Σk = fx 2 R : jsupp(x)j ≤ kg: m×n Do there exist an integer m, a \measurement matrix" A 2 R , m n and a \demodulation map" ∆ : R ! R , such that ∆(Ax) = x; 8x 2 Σk? Note: Measurements are linear but demodulation map could be nonlinear. The algorithm is universal { the same A and ∆ need to work for every vector x, and nonadaptive { one has to choose all m rows of A at the outset. M. Vidyasagar FRS Overview of Compressed Sensing What is Compressed Sensing? Main Results Construction of Measurement Matrices Some Topics Not Covered Further Considerations What if: The vector x is not exactly sparse, but only nearly sparse? The measurement is corrupted by noise, and equals Ax + η The vector x is (nearly) sparse in some other basis, and not the canonical basis (e.g., time and frequency)? M. Vidyasagar FRS Overview of Compressed Sensing What is Compressed Sensing? Main Results Construction of Measurement Matrices Some Topics Not Covered Some Definitions n Given a norm k · k on R , for each integer k < n, define σk(x; k · k) := min kx − zk; z2Σk the k-sparsity index of the vector x. x 2 Σk (x is k-sparse) if and only if σk(x; k · k) = 0 for every norm. If x 62 Σk, then σk(x; k · k) depends on the specific norm. The k-sparsity index w.r.t. to an `p-norm is easy to compute. Let Λ0 denote the index set of the k largest components by magnitude of x. Then σ (x; k · k ) = kx c k : k p Λ0 p M. Vidyasagar FRS Overview of Compressed Sensing What is Compressed Sensing? Main Results Construction of Measurement Matrices Some Topics Not Covered Precise Problem Statement: Final Given integers n; k n and a real number > 0, do there exist an m×n m n integer m, a matrix A 2 R , and a map ∆ : R ! R such that k∆(Ax + η) − xk2 ≤ C1σk(x; k · kp) + C2, m whenever η 2 R satisfies kηk2 ≤ ? Here C1;C2 are \universal" constants that do not depend on x or η. If so the pair (A; ∆) is said to display near-ideal signal recovery. This formulation combines several desirable features into one. M. Vidyasagar FRS Overview of Compressed Sensing What is Compressed Sensing? Main Results Construction of Measurement Matrices Some Topics Not Covered Interpretation Suppose x is k-sparse, and suppose y = Ax + η. If an \oracle" knows the support set of x, call it J, then the standard least-squares estimate is t −1 t t −1 t x^ = (AJ AJ ) AJ y = x + (AJ AJ ) AJ η; t −1 t kx^ − xk2 = k(AJ AJ ) AJ ηk2 ≤ C0kηk2; t −1 t where C0 is the induced norm of (AJ AJ ) AJ . With a pair (A; ∆) that achieves near-ideal signal recovery, we have kx^ − xk2 ≤ C2kηk2: So, if (A; ∆) achieves near-ideal signal recovery, then the estimation error is a constant multiple of that achievable by an \oracle," but without knowing the support set of x. M. Vidyasagar FRS Overview of Compressed Sensing What is Compressed Sensing? Main Results Construction of Measurement Matrices Some Topics Not Covered Interpretation (Cont'd) If x is not sparse, but measurements are noise-free (η = 0), then the estimate x^ = ∆(Ax) satisfies kx^ − xk2 ≤ C1σk(x; k · kp): So the estimate is within a \universal constant" times the k-sparsity index of x. If x is k-sparse and measurements are noise-free, we get exact signal recovery. M. Vidyasagar FRS Overview of Compressed Sensing What is Compressed Sensing? Main Results Construction of Measurement Matrices Some Topics Not Covered Outline 1 What is Compressed Sensing? 2 Main Results 3 Construction of Measurement Matrices Deterministic Approaches Probabilistic Approaches A Case Study 4 Some Topics Not Covered M. Vidyasagar FRS Overview of Compressed Sensing What is Compressed Sensing? Main Results Construction of Measurement Matrices Some Topics Not Covered Restricted Isometry Property Definition m×n A matrix A 2 R is said to satisfy the restricted isometry property (RIP) or order k with constant δk if 2 2 2 (1 − δk)kuk2 ≤ kAuk2 ≤ (1 + δk)kuk2; 8u 2 Σk: t If J ⊆ f1; : : : ; ng and jJj ≤ k, then the spectrum of AJ AJ lies in the interval [1 − δk; 1 + δk]. M. Vidyasagar FRS Overview of Compressed Sensing What is Compressed Sensing? Main Results Construction of Measurement Matrices Some Topics Not Covered Cande`es-Tao Result on `1-Norm Minimization Theorem Supposep A satisfies the RIP of order 2k with constant n δ2k < 2 − 1. Given x 2 R , define the demodulation map ∆ by ∆(y) =x ^ := arg min kzk1 s.t. Az = y: z Then ∆(Ax) = x; 8x 2 Σk: In plain English, any k-sparse vector can be recovered exactly by −1 minimizing kzk1 for z in A (Ax). Note that this is a linear programming problem. M. Vidyasagar FRS Overview of Compressed Sensing What is Compressed Sensing? Main Results Construction of Measurement Matrices Some Topics Not Covered A More General Result Theorem m×n Supposep A 2 R satisfies the RIP of order 2k with constant δ2k < 2 − 1, and that y = Ax + η where kηk2 ≤ . Define x^ = arg min kzk1 s.t. ky − Azk2 ≤ . z Then σk(x; k · k1) kx^ − xk2 ≤ C0 p + C2, k where p p 1 + ( 2 − 1)δ2k 4 1 + δ2k C0 = 2 p ;C2 = p : 1 − ( 2 + 1)δ2k 1 − ( 2 + 1)δ2k M. Vidyasagar FRS Overview of Compressed Sensing What is Compressed Sensing? Main Results Construction of Measurement Matrices Some Topics Not Covered Some Observations n Bounds are valid for all vectors x 2 R { no assumptions of sparsity. The smaller we can make the RIP constant δ, the tighter the bounds. We suspect that making δ smaller requires larger values of m (more measurements). Indeed this is so, as we shall discover next. M. Vidyasagar FRS Overview of Compressed Sensing What is Compressed Sensing? Deterministic Approaches Main Results Probabilistic Approaches Construction of Measurement Matrices A Case Study Some Topics Not Covered Outline 1 What is Compressed Sensing? 2 Main Results 3 Construction of Measurement Matrices Deterministic Approaches Probabilistic Approaches A Case Study 4 Some Topics Not Covered M. Vidyasagar FRS Overview of Compressed Sensing What is Compressed Sensing? Deterministic Approaches Main Results Probabilistic Approaches Construction of Measurement Matrices A Case Study Some Topics Not Covered Construction of Matrices with the RIP Challenge: Given integers n (dimension ofp the vector), k (desired level of sparsity), and real number δ 2 (0; 2 − 1), choose an m×n integer m and a matrix A 2 R such that A has the RIP of order 2k with constant δ. m×n Refresher: A matrix A 2 R is said to satisfy the restricted isometry property (RIP) or order k with constant δk if 2 2 2 (1 − δk)kuk2 ≤ kAuk2 ≤ (1 + δk)kuk2; 8u 2 Σk: Two distinct classes of approaches: deterministic and probabilistic. M. Vidyasagar FRS Overview of Compressed Sensing What is Compressed Sensing? Deterministic Approaches Main Results Probabilistic Approaches Construction of Measurement Matrices A Case Study Some Topics Not Covered Outline 1 What is Compressed Sensing? 2 Main Results 3 Construction of Measurement Matrices Deterministic Approaches Probabilistic Approaches A Case Study 4 Some Topics Not Covered M. Vidyasagar FRS Overview of Compressed Sensing What is Compressed Sensing? Deterministic Approaches Main Results Probabilistic Approaches Construction of Measurement Matrices A Case Study Some Topics Not Covered Coherence of a Matrix m×n Given a matrix A 2 R , assume w.l.o.g. that it is column-normalized, that is each column has `2-norm of one. Definition The one-column coherence µ1(A) is defined as µ1(A) := max max jhai; ajij: i2[n] j2[n]nfig The k-column coherence µk(A) is defined as X µk(A) := max max jhai; ajij: i2[n] S⊆[n]nfig;jS|≤k j2S Coherence quantifies how \nearly orthonormal" the columns are.
Details
-
File Typepdf
-
Upload Time-
-
Content LanguagesEnglish
-
Upload UserAnonymous/Not logged-in
-
File Pages49 Page
-
File Size-