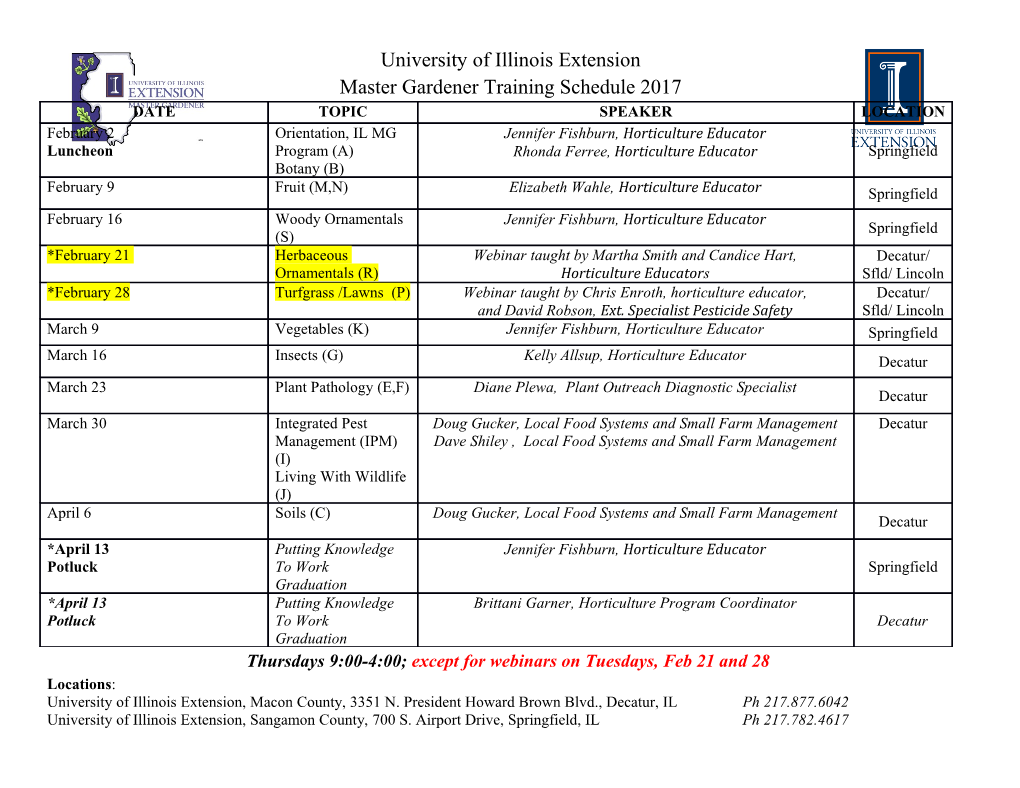
Robust Statistics Saragadam Introduction and overview Introduction Why robust statistics Robust Statistics Math primer Sensitivity curve Influence function Breakdown point Some robust estimation ideas Ad-hoc ideas The M-estimator Vishwanath Saragadam Raja Venkata ([email protected]) Robust estimation as the outcome of a April 29, 2015 distribution Visualizing some statistics Conclusion References 1/25 Robust Statistics Saragadam Introduction and overview Introduction Why robust statistics Math primer \A discordant small minority should never be able to Sensitivity curve Influence override the evidence of the majority of the observations." function Breakdown point { Huber (2011) Some robust estimation ideas Ad-hoc ideas The M-estimator Robust estimation as the outcome of a distribution Visualizing some statistics Conclusion References 2/25 Robust Statistics Introduction Saragadam Introduction and overview Introduction Why robust statistics Math primer I Modeling of data most likely will deviate from the actual Sensitivity curve model. Influence function Breakdown I Experimental errors might crop up into data. point Some robust I Inference might be grossly wrong in that case. estimation ideas I Can we come up with good statistics to capture these Ad-hoc ideas The uncertainties in model? M-estimator Robust I Is there a way to reduce the effect of outliers. estimation as the outcome of a distribution Visualizing some statistics Conclusion References 3/25 Robust Statistics Why robust statistics Saragadam Introduction and overview Introduction Why robust statistics Math primer Sensitivity I Find an inference method that describes majority of the curve Influence data. function Breakdown point I Identify outliers, i.e, data which does not fit the model. Some robust estimation I Talk about the influence of individual data points. ideas Ad-hoc ideas I Talk about how wrong the data has to be, to give a bad The M-estimator estimate.Ronchetti,hem,Tyler Robust estimation as the outcome of a distribution Visualizing some statistics Conclusion References 4/25 Robust Statistics What to expect from a robust statistic Saragadam Introduction and overview Introduction Why robust statistics Math primer Sensitivity I Efficiency: Reasonably good efficiency at the assumed curve Influence mode. function Breakdown point I Stability: A small deviation from the assumed model Some robust shouldn't return garbage statistics. estimation ideas Ad-hoc ideas I Breakdown: Large deviations shouldn't create a The M-estimator catastrophe. Robust estimation as the outcome of a distribution Visualizing some statistics Conclusion References 5/25 Robust Statistics Quantifying robustness { Sensitivity curve Saragadam Introduction and overview Introduction Why robust statistics Math primer n I Let T (fx g ) be a statistic. Then the sensitivity curve of Sensitivity n i i=1 curve Influence x, function Breakdown point SC(x; T ) = lim n[Tn(x1;:::; xn−1; x) − Tn−1(x1;:::; xn−1)] Some robust n!1 estimation ideas (1) Ad-hoc ideas The M-estimator I Quantifies the effect of an individual data point. Robust estimation as the outcome of a distribution Visualizing some statistics Conclusion References 6/25 Robust Statistics Quantifying robustness { Influence function Saragadam Introduction and overview Introduction Why robust statistics Let F be a distribution and F = (1 − )F + δ be the Math primer I x Sensitivity curve contaminated distribution. Influence function I Let T (F ) be a statistic. Then, Breakdown point Some robust estimation T (F) − T (F ) @ ideas IF (x; T ; F ) = lim = T (F) (2) !0 Ad-hoc ideas @ The =0 M-estimator Robust I For mean, IF (x; T ; F ) = x − µ¯ estimation as the outcome of a distribution Visualizing some statistics Conclusion References 7/25 Robust Statistics Quantifying robustness { Breakdown point Saragadam Introduction and overview Introduction Why robust I Define bias function: statistics Math primer 0 Sensitivity bias(m; Tn; X ) = sup kTn(X ) − Tn(X )k (3) curve x0 Influence function Breakdown 0 point Where X is X with m points replaced by corrupted points. Some robust estimation I Breakdown point, ideas Ad-hoc ideas m The ∗(T ; X ) = minf : bias(m; T ; X ) = 1g (4) M-estimator n n n n Robust estimation as the outcome I For mean, breakdown point is 0, because one rogue sample of a distribution is sufficient to take bias to 1 Visualizing some statistics Conclusion References 8/25 Robust Statistics Some Ad-hoc ideas Saragadam Introduction and overview Introduction Why robust statistics Math primer Sensitivity I Agree that data comes from a normal distribution. This curve Influence means, probability of outliers is very low. Solution? Drop function Breakdown point such data points! Called α-trimmed mean. Some robust I Another approach is to replace α proportion of tail data estimation ideas with it's closest observation. Called α-windsorized mean. Ad-hoc ideas The ∗ M-estimator I Break down point of both methods is = α Robust estimation as the outcome of a distribution Visualizing some statistics Conclusion References 9/25 Robust Statistics The M-estimator Saragadam Introduction and overview Introduction Why robust n statistics I Given data fxi gi=1 and statistic Tn. Math primer Sensitivity I Assume that we wish to minimize the following function: curve Influence function n Breakdown X point min ρ(xi ; Tn) (5) Some robust Tn estimation i=1 ideas Ad-hoc ideas The I Called an M-estimator, from Maximum likelihood type M-estimator estimator. Robust estimation as the outcome I If we wish to find location, then ρ(xi ; Tn) = ρ(xi − Tn) of a distribution Visualizing some statistics Conclusion References 10/25 Robust Statistics The M-estimator Saragadam Introduction and overview Introduction Why robust statistics I Differentiating eq. 5, Math primer Sensitivity curve n Influence X function (xi ; Tn) = 0 (6) Breakdown point i=1 Some robust estimation 1 2 ideas I Eg, if ρ(xi ; Tn) = 2 (xi − Tn) ; (xi ; Tn) = (xi − Tn). Ad-hoc ideas The Simple least squares solution. Give sample mean. M-estimator Robust I ρ(xi ; Tn) = jxi − Tnj; (xi ; Tn) = sign(xi − Tn). Gives estimation as the outcome median. of a distribution Visualizing some statistics Conclusion References 11/25 Robust Statistics The M-estimator Saragadam Introduction and overview Introduction Why robust statistics I Intuitively, we want to penalize a large number of points Math primer Sensitivity with small error, but relax on a few points with large error. curve Influence function I Huber loss function does this job. Breakdown point 1 2 Some robust 2 x jxj < k estimation hk (x) = 1 (7) ideas k(jxj − 2 k) jxj > k Ad-hoc ideas The M-estimator I Breakdown point is 0.5, meaning that, more than 50% of Robust estimation as the data has to be corrupt to give a bad estimate. the outcome of a distribution Visualizing some statistics Conclusion References 12/25 Robust Statistics Robust statistic as an outcome of a PDF Saragadam Introduction and overview Introduction Why robust statistics I All methods described previously are based on some kind of Math primer intuition to deal with error. Sensitivity curve Influence I Can a robust estimate be an outcome of a density function. function Breakdown point I Heavy tail distributions have higher probability for tail end Some robust samples. estimation ideas I Immediate distribution in mind: Cauchy distribution. Not Ad-hoc ideas The reliable if sampling is truly normal. M-estimator Robust I Can we get a control over the heaviness of the tail? Yes, estimation as the outcome student-t distribution Divgi (1990). of a distribution Visualizing some statistics Conclusion References 13/25 Robust Statistics Using Student-t distribution for robust estimation Saragadam Introduction and overview Introduction Why robust statistics Math primer Sensitivity curve Influence function Breakdown point Some robust estimation ideas Ad-hoc ideas The M-estimator Robust estimation as the outcome of a distribution Visualizing some statistics Figure: Image courtesy: Wikipedia. Conclusion References 14/25 Robust Statistics Using Student-t distribution for robust estimation Saragadam Introduction and overview I Let x come from a student-t distribution with center c, Introduction Why robust scale s and ν degrees of freedom. statistics x−c Math primer I Let u = s . Then, the density of x, Sensitivity curve Influence u − ν+1 function (1 + ) 2 Breakdown ν point fX (x) = p 1 ν (8) Some robust s νB( 2 ; 2 ) estimation ideas n Ad-hoc ideas I Log likelihood function for fxi gi=1, The M-estimator n 2 Robust ν + 1 X u p 1 ν estimation as L(c; s) = − log(1 + i ) − n log(s νB( ; )) the outcome 2 ν 2 2 of a i=1 distribution (9) Visualizing some statistics Conclusion References 15/25 Robust Statistics Using Student-t distribution for robust estimation Saragadam Introduction and overview Introduction I Differentiating with respect to c, Why robust statistics n Math primer @L ν + 1 X ui Sensitivity = 0 =) 2 = 0 (10) curve @c s ν + u Influence i=1 i function Breakdown n point X ≡ (x ; θ) = 0 Some robust i estimation i=1 ideas Ad-hoc ideas The M-estimator I Differentiating with respect to s, Robust n estimation as @L n ν + 1 X 2u2 the outcome = 0 =) − + i = 0 (11) of a @s s s ν + u2 distribution i=1 i Visualizing some statistics Conclusion References 16/25 Robust Statistics Using Student-t distribution for robust estimation Saragadam Introduction and overview Introduction Why robust statistics Math primer Sensitivity curve I c; s can be estimated with gradient descent or alternating Influence function maximization algorithm. Breakdown point I Tune ν for maximum log likelihood. Some robust estimation I Simple method, similar to M-estimator, and intuitive. ideas Ad-hoc ideas The I Free of parameters. M-estimator Robust estimation as the outcome of a distribution Visualizing some statistics Conclusion References 17/25 Robust Statistics Estimating mean using various methods Saragadam Introduction and overview Introduction Why robust statistics n Math primer I Consider the data fxi gi=1, of which, m data points are Sensitivity curve corrupted.
Details
-
File Typepdf
-
Upload Time-
-
Content LanguagesEnglish
-
Upload UserAnonymous/Not logged-in
-
File Pages25 Page
-
File Size-