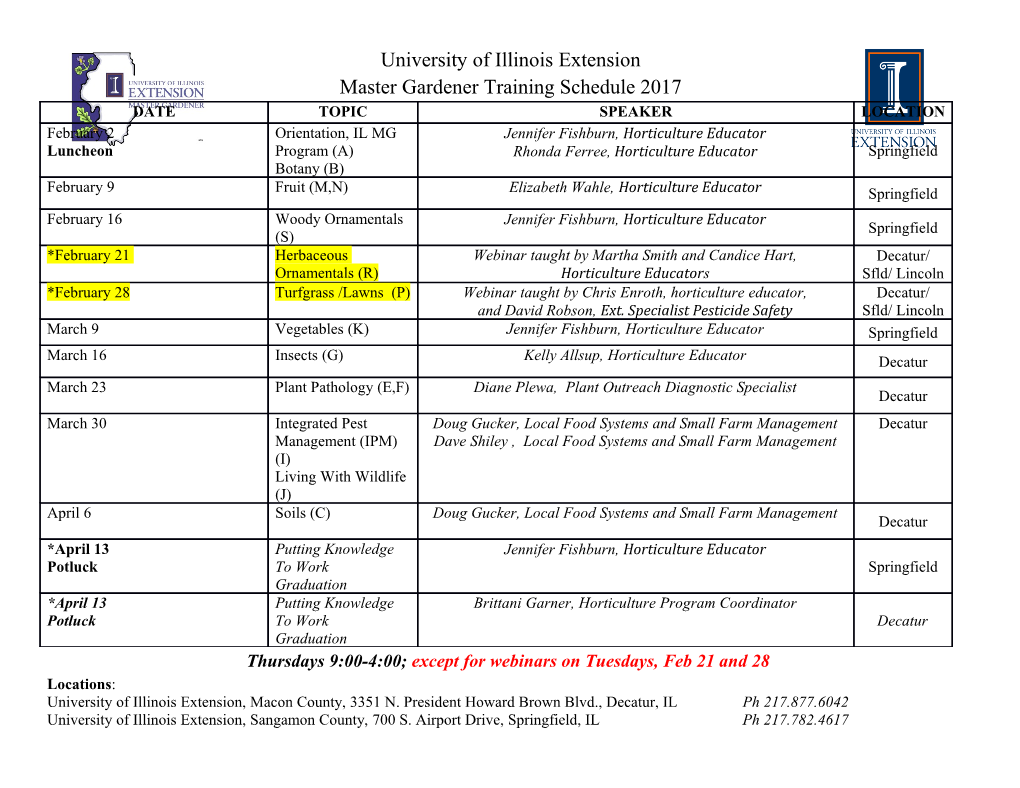
Feature Mining: A Novel Training Strategy for Convolutional Neural Network Tianshu Xie1 Xuan Cheng1 Xiaomin Wang1 [email protected] [email protected] [email protected] Minghui Liu1 Jiali Deng1 Ming Liu1* [email protected] [email protected] [email protected] Abstract In this paper, we propose a novel training strategy for convo- lutional neural network(CNN) named Feature Mining, that aims to strengthen the network’s learning of the local feature. Through experiments, we find that semantic contained in different parts of the feature is different, while the network will inevitably lose the local information during feedforward propagation. In order to enhance the learning of local feature, Feature Mining divides the complete feature into two com- plementary parts and reuse these divided feature to make the network learn more local information, we call the two steps as feature segmentation and feature reusing. Feature Mining is a parameter-free method and has plug-and-play nature, and can be applied to any CNN models. Extensive experiments demonstrate the wide applicability, versatility, and compatibility of our method. 1 Introduction Figure 1. Illustration of feature segmentation used in our method. In each iteration, we use a random binary mask to Convolution neural network (CNN) has made significant divide the feature into two parts. progress on various computer vision tasks, 4.6, image classi- fication10 [ , 17, 23, 25], object detection [7, 9, 22], and seg- mentation [3, 19]. However, the large scale and tremendous parameters of CNN may incur overfitting and reduce gener- training strategy for CNN for strengthening the network’s alizations, that bring challenges to the network training. A learning of local feature. series of training strategies proposed to solve these problems, Our motivation stems from an phenomenon in the training including data augmentation [4, 34, 36], batch normaliza- of CNN: information loss will inevitably occur after going tion [14] and knowledge distillation [11]. Those approaches through the pooling layer, which leads to the lack of local fea- make the network training more robust from the perspective ture learning in network. We perform several visualization of input, feature space and output. experiments [38] to explore the difference between global arXiv:2107.08421v1 [cs.CV] 18 Jul 2021 In particular, some methods improve the generalizations feature and local feature, and results empirically suggest that by strengthening the learning of local feature. As a represen- the semantic between different parts of the feature is differ- tative regularization method, dropout [24] randomly discards ent, and local feature contain different semantic compared parts of internal feature in the network to improve the learn- with global feature, while the network will inevitably lose ing of the remaining feature. Be your own teacher(BYOT) [35] these information during feedforward propagation. Contain- improves the efficiency of network training the by squeezing ing more knowledge of feature is benefit to the training of the knowledge in the deeper portion of the networks into CNN, but how to make the local feature of images sufficiently the shallow ones. These approaches are effective to network mined by network is a big challenge. training because the network is not able to learn the feature Based on the above observation, we propose a parameter- sufficiently. In this paper, we focus on developing anew free training method: Feature Mining, which aims to make the network learn local feature more efficiently during train- 1 Institution:University of Electronic Science and Technology of China. ing. The Feature Mining approach requires two steps: feature * Ming Liu is the corresponding author. segmentation and feature reusing. During feature segmen- tation, we divide the feature before pooling layer into two complementary parts by a binary mask as shown in Figure 1. Feature reusing denotes that the two parts will pass through a global average pooling(GAP) layer and a fully connection layer respectively to generate two independent outputs, and the three cross-entropies will be added to form the final loss functions. In this way, different local feature can participate in the network training, so that the network is able to learn more abundant feature expression. Feature Mining can also be extended to any layers of the network. Specially, adding Feature Mining in the last two layers of the network not only further improves the performance of the network, but also strengthens the shallow learning ability of network. Due to its inherent simplicity, Feature Mining has a plug-and-play nature. Beside, we do not need to set any hyper parameter when using Feature Mining, which can reduce the operating cost and maintain the stability of the method for performance improvement. We demonstrate the effectiveness of our simple yet effec- Figure 2. Class activation maps (CAM) [38] for ResNet-50 tive training method using different CNNs, such as ResNet [10], model trained on CUB-200-2011. Each line represents the DenseNet [13], Wide ResNet [32] and PyramidNet [8] trained visualization results of the same network in different im- for image classification tasks on various datasets including ages, while the networks beginning from the third line were CIFAR-100 [16], TinyImageNet and ImageNet [23]. Great trained with a fixed binary masks on the the last layer, which accuracy boost is obtained by Feature Mining, higher than means only part of the features of the last layer of the net- other dropout [6, 24, 26] and self distillation methods [31, 35]. work could participate in the network training. The first In addition, our training method has strong compatibility column represents the composition of these binary masks, and can be superimposed with the mainstream data aug- the gray area represents the discarded area, and only the mentation [34] and self distillation method. Besides, we ver- features in the white area participate in the training ify the wide applicability of Feature Mining in fine-grained classification [27], object detection [5] and practical scenar- ios [2, 33]. In summary, we make the following principle contribu- tions in this paper: • We proposed a novel training strategy Feature Mining Class activation maps (CAM) [38] was chosen to visualize aims to make the network learn local feature more the trained model. Specifically, the ResNet50 [8] trained by efficiently. Our method enjoys a plug-and-play nature CUB-200-2011 [27] was used as the baseline model. All the and is parameter-free. models were trained from scratch for 200 epochs with batch • The prosed training approach can obviously improve size 32 and the learning rate is decayed by the factor of 0.1 the network’s performance with the increase of lit- at epochs 100, 150. tle training cost and can be superimposed with the As shown in Figure 2, the second line shows the visualiza- mainstream training methods. tion results of the complete feature in the last layer, while • Experiments for four kinds of convolutional neural next lines show the visualization results of differen local networks on three kinds of datasets are conducted to feature. We can see that the complete feature generally fo- prove the generalization of this technique. Tasks in cused on the global information of the target, while the local four different scenarios demonstrate the wide applica- feature focused more on the relevant local information ac- bility of our method. cording to their locations. Obviously, the image information concerned by local feature is different from that of global feature, and even the semantic information between different 2 observation local feature is different. However, Due to the feed-forward In order to explore the semantic difference among the local mechanism of CNN, the network only gets the output de- feature, we conducted series of experiments on the visualiza- pending on the global feature information, which may cause tion of CNN. During the network training, we multiplied the the loss of information contained in the local feature. How features of the last layer of the network by different fixed to make full use of feature information, especially the local binary masks, so that only part of the features of the last feature, in network training? We will see how to address this layer of the network can participate in the network training. issue next. Figure 3. This figure shows the details of a CNN training with proposed Feature Mining. There are two stages inFeature Mining: feature segmentation and feature reusing. The feature is divided into two parts and the final three cross-entropies are added as the final loss function. 3 Feature Mining set to one and other elements in " are set to zero. We define Feature Mining is designed to improve the learning of local the segmentation operation as feature by networks. Here we present some notations which -1 = - ⊙ " will be used in the following. For a given training sample (3) - = - ⊙ ¹ − "º ¹G,~º which G 2 R, ×퐻×퐶 denotes the training image and~ 2 2 1 . = f1, 2, 3, ...,퐶g denotes the training label, and a network where -1 and -2 are complementary and contain different with # classifiers. - denotes the network’s feature of the knowledge of -, which means -1 only contains the feature training sample. For the = classifier, its output is 0=. We use information within 퐵 while -2 only contains the feature in- softmax to compute the predicted probability: formation outside 퐵. We use block to segment feature based 4G?¹0=º on the characteristics of convolutional neural network: ad- ?= = 8 8 Í= 4G? 0= (1) jacent neurons have similar semantics, and block form seg- 9=1 ¹ 9 º mentation can ensure that the two parts of feature have great Next we will describe the proposed Feature Mining strategy differences. Note that this operating can also be applied in 8.4 in detail, which consists of two steps , feature segmenta- any layer to get different representative feature and is not tion and feature reusing, as depicted in Figure 2.
Details
-
File Typepdf
-
Upload Time-
-
Content LanguagesEnglish
-
Upload UserAnonymous/Not logged-in
-
File Pages10 Page
-
File Size-