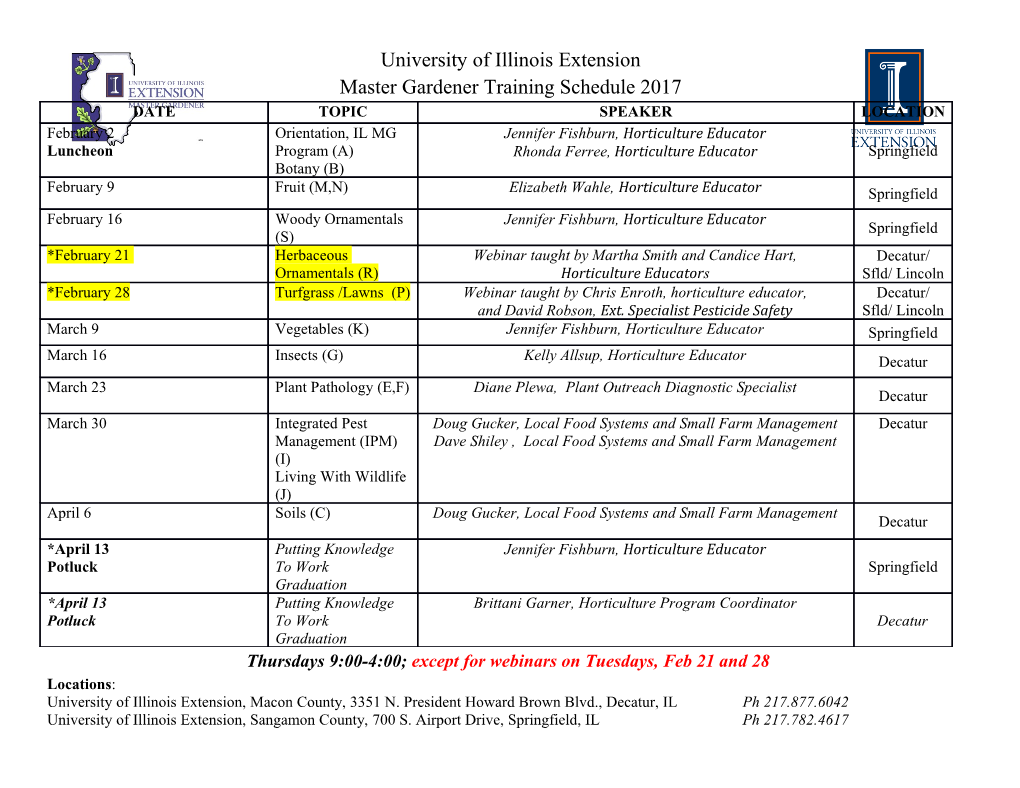
Dynamic causal modeling for fMRI Methods and Models for fMRI, HS 2016 Jakob Heinzle Structural, functional & effective connectivity Sporns 2007, Scholarpedia anatomical/structural functional connectivity effective connectivity connectivity - presence of physical - statistical dependency - causal (directed) connections between regional time influences between - DWI, tractography, series neuronal populations tracer studies (monkeys) - correlations, ICA - DCM Dynamic causal modelling (DCM) for fMRI • DCM framework was introduced in 2003 for fMRI by Karl Friston, Lee Harrison and Will Penny (NeuroImage 19:1273-1302) • part of the SPM software package • Allows to do an effective connectivity analysis D-ITET / IBT / TNU 3 From functional segregation to functional integration localizing brain activity: effective connectivity analysis: functional segregation functional integration A B A B ? u u1 u2 1 u2 u1 u1 X u2 « Where, in the brain, did « How did my experimental manipulation my experimental manipulation propagate through the network? » have an effect? » Introductory example: Attention to motion Assess site of attention modulation during visual processing in fMRI paradigm reported by Büchel and Friston. How can the classical results be explained mechanistically: Photic V1 + Motion V5 + Attention V5 + parietal cortex (SPC) Friston et al. (2003) NeuroImage Bayesian model selection m1 m2 m3 m4 Modulation Modulation Modulation Modulation By attention By attention By attention By attention PPC PPC PPC PPC stim V1 V5 stim V1 V5 stim V1 V5 stim V1 V5 ( | ) ( | ) ( | ) ( | ) 1 2 3 4 → → attention→ → models marginal likelihood 0.10 ln p(y m) PPC 0.39 0.26 1.25 0.26 stim V1 0.13 V5 0.46 estimated effective synaptic strengths for best model (m4) Stephan et al. 2008, NeuroImage Parameter inference attention MAP = 1.25 0.10 0.8 0.7 PPC 0.6 0.26 0.5 1.25 0.39 0.4 0.26 stim V1 0.13 0.3 V5 0.2 0.46 0.1 0.50 0 -2 -1 0 1 2 3 4 5 PPC p(DV 5,V 1 > 0 | y) = 99.1% motion We will run this example in the tutorial Stephan et al. 2008, NeuroImage Generative model py(|,)θ m⋅pm(|θ ) p(|,)θ ym 1. enforces mechanistic thinking: how could the data have been caused? 2. generate synthetic data (observations) by sampling from the prior – can model explain certain phenomena at all? 3. inference about model structure: formal approach to disambiguating mechanisms → p(m|y) 4. inference about parameters → p(θ|y) DCM approach to effective connectivity A simple model of a … described as a … causes the data neural network … dynamical system … (BOLD signal). ( ) = ( , , ) = ( , ) ( ) ( ) ̇ Let the system run with input ( ) and parameters ( , ), and you will get a BOLD signal time course that you can ( ) Neural node compare to the measured data. Input ( ) Connections ( ) Approximating ( , , ) = , , + + + + + ≈ ⋯ Bi-linear model Non-linear model Modulation Modulation By attention By attention PPC PPC stim V1 V5 stim V1 V5 Approximating ( , , ) = , , + + + + + ≈ ⋯ Bi-linear model Non-linear model Modulation Modulation By attention By attention PPC PPC stim V1 V5 stim V1 V5 The neural model – summary. Modulatory input Driving input u2(t) Parameter sets… t u1(t) t ( ) A - fixed connectivity ( ) B - modulation of ( ) connectivity C - weight of driving inputs ( ) Neural node D – weight of non-linear Driving input ( ) 1 terms Connections Modulatory input ( ) … determine dynamics! 2 The neural equations – bilinear model Modulatory input Driving input u2(t) t u1(t) t ( ) ( ) ( ) = + + ( ) � =1 Parameters A, B and C define connectivity! The neural equations – non-linear model Driving input u1(t) t ( ) ( ) ( ) ( ) = + + + ( ) � � =1 =1 Modulatory input u2(t) t Parameters A, B, C and D define connectivity! DCM parameters = rate constant a11 dx1 = a11x1 x1(t) = x1(0)exp(a11t) x1 dt Decay function 1 A 0.8 If AB is 0.10 s-1 this means that, 0.6 0.10 per unit time, the increase in 0.5x1(0) 0.4 activity in B corresponds to 10% of 0.2 the current activity in A 0 B -0.1 0 0.1 0.2 0.3 0.4 0.5 0.6 0.7 0.8 0.9 τ = ln 2 / a11 Bilinear neural state equation State External Current External changes modulatory state driving Modulatory input Driving input inputs inputs u2(t) t u1(t) t = + ( ) + ( ) ( ) ̇ � ( ) =1 = , , … , , Fixed Weights Weights for connectivity (strength) of direct inputs weights connectivity modulation The problem of the hemodynamic response ↑ neural activity oxy- Hb deoxy- Hb ↑ blood flow Rest Peak Brief ↑ oxyhemoglobin Stimulus Undershoot ↑ T2* Initial dip ↑ MR signal Activity Source, Huettel et al, 2004, fMRI (Book) From neural activity to the BOLD signal Local hemodynamic state equation = vasodilatory signal and flow = ̇ − − − Induction ( ) (rCBF) ̇ Balloon model = / / = ( , )/ / ̇ − ̇ Changes in− volume ( ) and dHb ( ) BOLD signal change equation cf. Simulations in = + + Lecture 1 ≈ − − − The hemodynamic u stimulus functions model in DCM t m dx ( j) = A + ∑u j B x + Cu dt neural state equation • 6 hemodynamic parameters: j=1 vasodilatory signal s = x −κs − γ( f −1) h s θ = {κ,γ ,τ ,α, ρ,ε} f s flow induction (rCBF) f = s important for model fitting, hemodynamic f but of no interest for state equations statistical inference Balloon model changes in volume v changes in dHb 1/α 1/α τv = f − v τq = f E( f,E0 ) qE0 − v q/v • Computed separately for each v q area (like the neural parameters) ∆S q → region-specific HRFs! λ(q,v) = ≈V0 k1(1− q)+ k2 1− + k3(1− v) S0 v k1 = 4.3ϑ0E0TE BOLD signal Friston et al. 2000, NeuroImage k2 = εr0E0TE change equation Stephan et al. 2007, NeuroImage k3 = 1− ε u stimulus functions The hemodynamic t model in DCM – role of ε dx m neural state = + ( j) + A ∑u j B x Cu equation dt j=1 0.4 0.2 vasodilatory signal 0 s = x −κs − γ( f −1) 0 2 4 6 8 10 12 14 RBM , ε = 0.5 s N CBM , ε = 0.5 f s N RBM , ε = 1 1 N CBM , ε = 1 flow induction (rCBF) N RBM , ε = 2 0.5 N = CBM , ε = 2 hemodynamic f s N state 0 f equations 0 2 4 6 8 10 12 14 Balloon model 0.2 0 changes in volume changes in dHb v -0.2 1/α 1/α τv = f − v τq = f E( f,E0 ) qE0 − v q/v -0.4 v q -0.6 0 2 4 6 8 10 12 14 ∆S q λ(q,v) = ≈V0 k1(1− q)+ k2 1− + k3(1− v) S0 v k1 = 4.3ϑ0E0TE BOLD signal k2 = εr0E0TE = − ε change equation k3 1 Stephan et al. 2007, NeuroImage Hemodynamic forward models are important for connectivity analyses of fMRI data Granger causality DCM David et al. 2008, PLoS Biol. Summary – the full model Input Inputs ( ) Neuronal states ( ) ( ) Hidden ( ) states Hemodynamic model ( ) and ( ) ( ) BOLD ( ) signal change equation ( ) ( ) BOLD signal y(t) t Summary – the full model Input Inputs ( ) Neuronal states ( ) ( ) Hidden ( ) states Hemodynamic model ( ) and ( ) ( ) BOLD ( ) signal change equation ( ) ( ) BOLD signal y(t) t Summary – parameters of interest Inputs Neuronal states Connection weights: A, B, C and D ( ) Hemodynamic model ( ) and ( ) Hemodynamic parameters: τ, κ, ε BOLD signal change equation ( ) BOLD signal y(t) t Example traces 1: Single node context u2 - ( ) ( ) - stimuli + u1 = + ( ) + 0 2 0 0 0 = 2 + 1 + ̇ 0 0 0 0 0 1 1 1 11 1 ̇ 2 � 2 � 2 � 2 ̇2 Example traces 2: Connected nodes context u2 - ( ) ( ) - stimuli + + u1 = + ( ) + 0 2 0 0 0 = 2 + 1 + ̇ 0 0 0 0 1 1 1 11 1 ̇ 2 � 2 � 2 � 2 ̇2 12 Example traces 3: Modulation of connection context u2 - ( ) - ( ) - stimuli + + u1 = + ( ) + 2 0 0 2 0 1 0 ̇ = + ( ) + 0 0 0 1 1 1 11 1 ̇ 2 2 � 2 � 2 � 2 ̇2 12 12 Example traces 4: Modulation of self-connection context u2 + - ( ) ( ) - stimuli + + u1 = + ( ) + 2 0 0 20 1 0 ̇ = + ( ) + 0 0 0 1 1 1 11 1 ̇ 2 2 � 2 � 2 � 2 ̇2 12 22 Bayesian inference – forward and inverse model forward problem py( ϑ, m) likelihood posterior distribution p(ϑ ym, ) inverse problem Bayesian inference Likelihood: Prior: θ Bayes rule: generative model m Dynamical systems in Bayes Assume data is normally distributed around the prediction from the dynamical model.v ( ) , = ( , ) Dynamical model defines the likelihood! Combining likelihood (data) and priors Likelihood = Probability of data Priors (constraints): Derived from dynamical system Hemodynamic parameters Gaussian noise empirical Neural parameters self connections: principled other parameters (inputs, connections): shrinkage , , = � The likelihood of the data , = ( ) , � Type role and impact of priors • Types of priors: Explicit priors on model parameters (e.g., connection strengths) Implicit priors on model functional form (e.g., system dynamics) Choice of “interesting” data features (e.g., regional timeseries vs ICA components) • Role of priors (on model parameters): Resolving the ill-posedness of the inverse problem Avoiding overfitting (cf. generalization error) • Impact of priors: On parameter posterior distributions (cf. “shrinkage to the mean” effect) On model evidence (cf. “Occam’s razor”) On free-energy landscape (cf. Laplace approximation) Model estimation: running the machinery Goal: Find posterior of parameters , that maximises model evidence given data and priors This is often not possible analytically approximate methods are used BMS and BMA Klaas Enno Stephan Variational Bayes (VB) Idea: find an approximate density ( ) that is maximally similar to the true posterior . This is often done by assuming a particular form for (fixed form VB) and then optimizing its sufficient statistics. true hypothesis posterior class divergence KL || best proxy Variational Bayes ln ln ln ( ) = KL[ | + , ∗ KL[ | divergence neg. free , 0 energy KL[ | (unknown) (easy to evaluate ≥ for a given ) , is a functional wrt.
Details
-
File Typepdf
-
Upload Time-
-
Content LanguagesEnglish
-
Upload UserAnonymous/Not logged-in
-
File Pages65 Page
-
File Size-