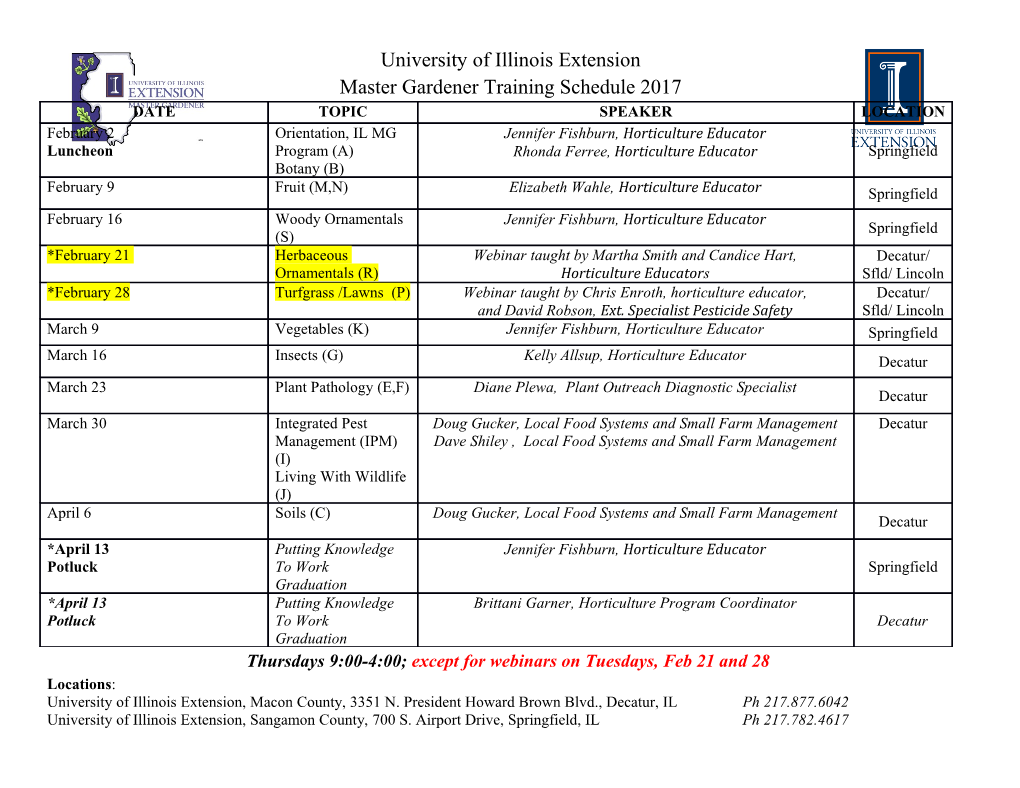
Real-Time Tracking of the Selective Auditory Attention from M/EEG via Bayesian Filtering Sina Miran1, Sahar Akram2, Jonathan Z. Simon1, Tao Zhang2, Behtash Babadi1 1 University of Maryland College Park (UMD) 2 Starkey Hearing Technologies Problem Overview Proposed Framework EEG Analysis (Decoding Model) MEG Analysis (Encoding Model) Cocktail Party Effect: the ability to identify and track a target speaker amid a our proposed framework for real-time attention decoding includes three modules: Experiment Specifications: • 6 subjects, dual-speaker setting, constant-attention and attention-switch experiments cacophony of acoustic interference [1] • 3 subjects, instructed constant attention on speaker 1, two speakers • estimation parameters similar to the EEG analysis humans can rapidly switch attention across multiple speakers • 64-channel EEG recording, 24 trials each 60s, downsampled to 푓푠 = 64퐻푧 • attention marker: real-time M100 magnitude estimates in the TRFs example TRF estimation results and state-space outputs: Attention Decoding Framework: Constant-Attention Attention-Switch • decoder estimation parameters: 푊 = 0.25푓 , considered EEG delays up to 푠 푊 0.25s, 훾 = 0.4, 휆 = 0.975 (effective data length of = 10푠) 1−휆 푓푠 (푖) 푖 • attention marker: ℓ1 norm of the decoder, i.e., 푚푘 = 휽푘 1 Dynamic Encoder/Decoder Estimation: rationale: detects significant decoder peaks Simplified Computational Problem: In a dual-speaker environment, can we decode • consider 퐾 consecutive non-overlapping windows of length 푊 samples • fixed-lag sliding window parameters: 퐾푊 = 15푓푠, 퐾퐹 = 1.5푓푠 the attentional state in real-time from the clean speech signals of the two speakers • 휽 • total attention decoding delay: 1.5s + 0.25s = 1.75s update the encoder/decoder estimates 푘 for each speaker in every window: 푘 and the multi-channel magnetoencephalography (MEG) or electroencephalography 푘−푗 2 Example Trial Outputs: 휽푘 = arg min 휆 풚푗 − 푿푗휽 + 훾 휽 1 , 푘 = 1,2, … , 퐾 (EEG) measurements of the listener’s brain? 휽 푗=1 2 • separating power of the attention marker decreasing from case 1 to 3 attention decoding in real-time from non-invasive neuroimaging data forgetting factor ℓ1 regularization penalty • second row shows inferred 푝푘’s in our real-time framework applications in Brain-Computer Interface (BCI) systems and smart hearing aids • third row shows inferred 푝푘’s in the batch-mode case, where the state-space speech envelopes (dec.) M/EEG covariates (dec.) (푖) processes all 푚 ’s at once neural response (enc.) envelope covariates (enc.) 푘 Existing methods: • 훾 chosen by cross-validation, 휆 chosen considering the inherent dynamics of data linear decoding models linearly map M/EEG data to stimulus linear encoding models linearly map stimulus to a neural response from M/EEG • estimation alg.: Forward-Backward Splitting (FBS) with real-time implementation Examples: Attention Marker: • reverse-correlation or stimulus reconstruction in decoding models (EEG) [2]: • compute a feature for each speaker from the set of measurements and estimated (푖) 1. train a decoder on the attended speech using training data encoder/decoder coefficients in every window 푘 푚푘 for 푖 = 1,2 2. use the attended decoder on the EEG data to reconstruct a stimulus • potential examples: 3. speech signal which has the highest correlation with the reconstructed reverse-correlation in decoding models: 푚(푖) = corr(풚 푖 , 푿 휽 (푖)) stimulus considered as the attended speech 푘 푘 푗 푘 (푖) • important stimulus time lags in encoding models (MEG) [3][4]: M100 peak magnitude in MEG encoding models: 휽푘 near the 100ms delay 1. estimate the encoding coefficients for each speaker, i.e., Temporal average classification accuracy in a trial for each subject: average classification accuracy in a trial for each subject: Response Function (TRF), in a test trial Dynamic State-Space Model: 2. the attended speaker has a larger M100 (the peak close to 100ms delay) • at 푘 = 푘0, consider a fixed-lag sliding window: backward-lag forward-lag Shortcomings for Real-Time Attention Decoding: • temporal resolution ~ tens of seconds (too slow given the dynamics of auditory processing) (푖) • operation in the batch-mode regime (requiring the entire data from one or multiple total number of 푚푘 ’s trials at once for processing) in the window • need large training datasets to estimate the attended encoder/decoder reliably for (푖) • dynamic state-space model: defined on the 푚푘 ’s in the sliding window use in test trials (not available in real-time applications) for an interpretable, probabilistic, and robust measure of attentional state State-Space Model Observation Equations References 1 푝 = 푃 푛 = 1 = 푖 푎 푎 푘 푘 푚 푛푘 = 푖 ~ LogNormal 휌 , 휇 [1] Cherry, E. Colin. "Some experiments on the recognition of speech, with one and with two ears." The 1 + exp −푧푘 푘 MSE of inferred 푝푘’s w.r.t. the batch-mode as a function of 퐾퐹: Summary Journal of the acoustical society of America 25.5 (1953): 975-979. 푧푘 = 푧푘−1 + 푤푘 푚 푖 푛 ≠ 푖 ~ LogNormal 휌 푢 , 휇 푢 rationale: batch-mode can serve as the ground truth for our framework [2] O'sullivan, James A., et al. "Attentional selection in a cocktail party environment can be decoded from 푘 푘 • a new framework for real-time attention decoding in competing speaker environments: 푤푘~푁 0, 휂푘 single-trial EEG." Cerebral Cortex 25.7 (2014): 1697-1706. real-time estimation of encoding or decoding coefficients [3] Ding, Nai, and Jonathan Z. Simon. "Emergence of neural encoding of auditory objects while listening to (푎) (푢) (푎) (푢) • model parameters: 푧1:퐾푊, 휂1:퐾푊, 휌 , 휌 , 휇 , 휇 computing a feature from the estimates and recorded data competing speakers." Proceedings of the National Academy of Sciences109.29 (2012): 11854-11859. ∗ [4] Akram, Sahar, Jonathan Z. Simon, and Behtash Babadi. "Dynamic Estimation of the Auditory Temporal • goal at 푘 = 푘0: estimate 푝푘∗ = logistic(푧푘∗) where 푘 = 푘0 − 퐾퐹 apply a state-space model on the features for a statistically interpretable and robust Response Function From MEG in Competing-Speaker Environments." IEEE Transactions on Biomedical • inference algorithm: apply the EM algorithm in the sliding window [5] measure of the attentional state Engineering 64.8 (2017): 1896-1905. • quality of the chosen feature in attention marker ∝ separation between the fitted • high temporal resolution and no need for large training datasets, unlike existing methods [5] Akram, Sahar, et al. "Robust decoding of selective auditory attention from MEG in a competing-speaker attended and unattended LogNormal distributions environment via state-space modeling." NeuroImage 124 (2016): 906-917. • serves as a step towards attention decoding for emerging real-time applications Supported in part by the National Science Foundation Award No. 1552946 and a Collaborative Research Grant from Starkey Hearing Technologies Poster PDF available at: http://ter.ps/simonpubs , Email: [email protected] .
Details
-
File Typepdf
-
Upload Time-
-
Content LanguagesEnglish
-
Upload UserAnonymous/Not logged-in
-
File Pages1 Page
-
File Size-