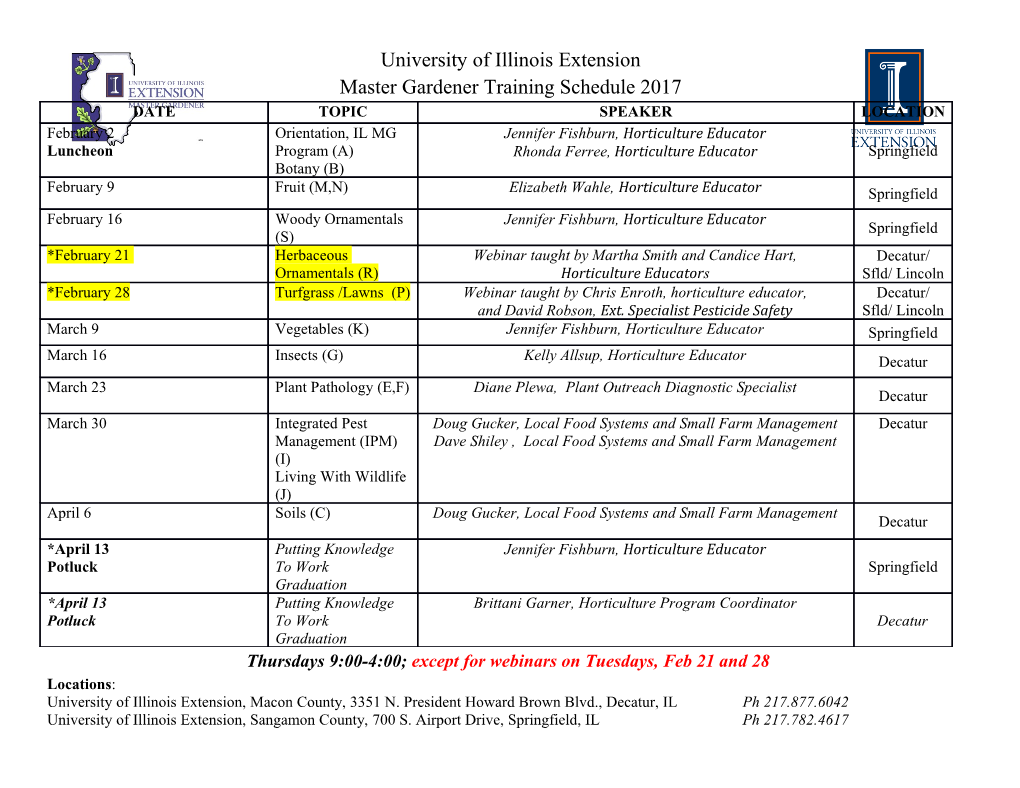
An automatic authorship attribution technique for Android applications by Hugo F. Gonzalez Robledo M.Sc. in Computer Science, Technological Institute of San Luis Potosi, Mexico, 2005. Bachelor of Software Engineering, Technological Institute of San Luis Potosi, Mexico, 2000. A THESIS SUBMITTED IN PARTIAL FULFILLMENT OF THE REQUIREMENTS FOR THE DEGREE OF Doctor of Philosophy In the Graduate Academic Unit of Faculty of Computer Science Supervisor(s): Ali A. Ghorbani, Ph.D., Faculty of Computer Science, UNB Natalia Stakhanova, Ph.D., Faculty of Computer Science, UNB Examining Board: Rodney Cooper, Professor, Faculty of Computer Science, UNB, Chair Ronxing Lu, Ph.D., Faculty of Computer Science, UNB Brent Petersen, Ph.D., Department of Electrical and Computer Engineering, UNB External Examiner: Nur Zincir-Heywood, Ph.D., Faculty of Computer Science, Dalhousie University This thesis is accepted Dean of Graduate Studies THE UNIVERSITY OF NEW BRUNSWICK September, 2017 c Hugo F. Gonzalez Robledo, 2017 Abstract In recent years, mobile devices have overcome desktop computers in usage. The most popular system for mobile devices is Android; hence, it is the most targeted by malicious applications. Typical signature detection systems had been proved to be ineffective for targeted attacks; therefore, other approaches should be explored. It is a common believe that only a handful of skilled au- thors exists to produce new malware, the overwhelming amount of current samples are adaptations, evolution, and modifications of an original strain. It is possible to associate a piece of malware with the adversary behind it by employing authorship attribution techniques. In other words, we believe that personal preferences of mobile software developers (including malware developers) leave traces in the produced software, i.e., apps. These prefer- ences are unique to each developer. Discovering these preferences would allow us to attribute an unknown app to an author. In this thesis, we proposed, developed, and evaluated a framework to automatically discover and create new author profiles of unknown Android apps on an open world problem. To accomplish this goal, we prepare and release two new Android datasets, one ii for legitimate and the other for malicious authors. Also, we define a set of stylistic features extracted from Android apk files to be used by the frame- work. The heart of the framework is an algorithm to discover and create new author profiles from a stream of apps; it uses machine learning boosting techniques to calculate prediction probability of new samples, then group them and measure their cosine similarity, and finally discard outliers. We employ a 5-fold cross-validation over a fixed set of apps with over a 99% of accuracy, precision, and recall. For unknown apps, we measure how many were attributed and how many were attributed to suspicious profiles. In our extensive experiments we discover five apps in the official market related to ransomware profile that were not detected by VirusTotal service as malicious immediately, but a couple of weeks later. We also present a use case where our solution is employed to evaluate the threat landscape in the official Android market. iii Dedication To God, the Almighty Creator. To Nadia, the love of my life, for all the support, love, care and sharing dur- ing this journey. To Jimena and Daniela, two little big reasons to keep going, for all the love, lessons and those enjoyable moments. To my parents Rosario and Jorge, for all the love, care and encouraging dur- ing my hole life. To my ancestors, because without them, I won't be here being who I am. iv Acknowledgements Foremost, I would like to thank my PhD advisers, Dr. Ali A. Ghorbani and Dr. Natalia Stakhanova, for the continuous support and guidance during my study and research these past four years. Thank you for your patience, motivation, enthusiasm and support; even when you were very busy with your appointments, you always found time to answer e-mails, have quick walking talks or discuss about new ideas and projects. I also would like to thank the rest of my thesis committee: Professor Cooper, Professor DeDourek, Dr. Lu, Dr. Petersen and Dr. Zincir-Heywood for their time, encouragement, insightful comments, and interesting questions. I thank my research colleagues at former Information Security Centre of Ex- cellence (ISCX) (now Canadian Institute for Cybersecurity (CIC)) for the stimulating discussions and exciting projects we worked on together. Espe- cially to Abdulla, Amirhosseing, Andi Fitriah for Android related discussions, ideas, projects and experiments. Thanks to Ratinder, Alina and Yan for the discussions, ideas and hard work in other several projects. Thanks to all the students and staff that converged in the \lab", with more or less interaction, v I'm sure that I learned something from you, and I hope you learned some- thing from me. Also my gratitude to the fellas that helped me to rediscover my passion for the volleyball, organizing the team to play regularly at UNB and outside of the University. I should express my gratitude to my previous mentors, friends, colleagues and family members who encourage me to continue my studies abroad. Thanks to \The Honeynet Project" and its members, who opened my view and helped me to view the world from a different perspective. I need to thank my family for all the support during these years: my parents, my wife and my kids. Last but not the least, I would like to thank Mexico's Government for my PROMEP scholarship during the first three years of my PhD studies. And of course all the support from Universidad Politecnica de San Luis Potosi. vi Table of Contents Abstract ii Dedication iv Acknowledgments v Table of Contents vii List of Tables ix List of Figures xi 1 Introduction 1 1.1 Problem Statement . 5 1.2 Research Motivations . 6 1.2.1 Challenges . 8 1.3 Contributions . 10 1.4 Thesis outline . 13 2 Background and related work 15 vii 2.1 Authorship attribution process . 16 2.1.1 Features . 17 2.1.2 Representations . 20 2.1.3 Authorship attribution methods . 25 2.1.3.1 Profile-based approach . 27 2.1.3.2 Instance-based approach . 28 2.1.3.3 Hybrid approach . 29 2.2 Basic introduction to Android platform . 30 2.3 Related work . 36 2.3.1 Source code authorship attribution . 37 2.3.2 Binary code authorship attribution . 41 2.3.3 Malware analysis . 43 2.3.4 Android malware analysis . 45 2.4 Concluding remarks . 48 3 An automatic authorship attribution technique for An- droid applications 51 3.1 Filter . 53 3.2 Feature extraction . 55 3.2.1 Features extracted. 58 3.3 Feature representation. 69 3.4 Attribution and Discovery approach . 73 3.5 Prototype implementation . 79 viii 3.5.1 Architectural view . 79 3.5.2 Implementation challenges . 87 3.5.3 Technical details of developed software components . 89 3.6 Summary . 93 4 Experimental results 95 4.1 Datasets . 96 4.2 Analysis of the authors' datasets . 102 4.3 Evaluation metrics . 106 4.4 Reference profiles validation. 108 4.5 Tuning thresholds . 114 4.6 Evaluation of emergent-profiles. 121 4.7 Experimenting with attribution only approach. 129 4.8 Concluding remarks . 137 5 Conclusions and future work 139 Bibliography 144 A Evolution of studies about Authorship Attribution 167 A.1 Bibliography . 167 Vita ix List of Tables 2.1 Summary of features and some examples. 21 3.1 Android packers detected. 55 3.2 Groups of features. 56 3.3 Directly extracted features. 58 3.4 Inferred features from string section. 59 3.5 Features composed by opcodes. 62 3.6 Features composed from a .dex file. 65 3.7 Features non related to a .dex file. 68 3.8 Features type and representations. 70 4.1 Datasets. 97 4.2 Relation between experiment stages and datasets. 102 4.3 Experimental results from filter step for legitimate and mali- cious authors. 104 4.4 Filters created with CommonCode tool. 105 4.5 Experimental results from created filters over legitimate and malicious authors. 105 x 4.6 Experimental results of signature distribution among legiti- mate and malicious authours. 106 4.7 Validation on legitimate authors dataset. 111 4.8 Validation on malicious authors dataset. 112 4.9 Validation over all authors. 113 4.10 Experimental results from discovery approach considering mal- ware families as coming from same author. 123 4.11 Experimental results for emergent-profiles considering mal- ware families as coming from same author. (First Part). 125 4.12 Experimental results for emergent-profiles considering mal- ware families as coming from same author. (Second Part). 126 4.13 Experimental results from discovery approach over legitimate apps. 129 4.14 Experimental results for authorship attribution over a large unknown suspicious stream of apps. 131 4.15 Experimental results for authorship attribution over a large unknown stream of apps. 132 4.16 Experimental results from attribution over new families' apps. 135 xi List of Figures 2.1 General steps to the process of software authorship attribution. 16 2.2 Android package (.apk) . 31 2.3 Android application project build process . 33 2.4 The structure of a typical plain .dex file. 35 2.5 Evolution of studies on software authorship attribution. 38 3.1 The flow of proposed approach to work on Android apps au- thorship attribution. 52 3.2 Overview of filter phase. 53 3.3 The process of building Bloom filters . 61 3.4 StructSignature technique description. 65 3.5 Creation of feature vector. 72 3.6 Attribution and Discovery approach. 74 3.7 Five categories holding different modules of our system. 81 3.8 External modules related each package of our system.
Details
-
File Typepdf
-
Upload Time-
-
Content LanguagesEnglish
-
Upload UserAnonymous/Not logged-in
-
File Pages188 Page
-
File Size-