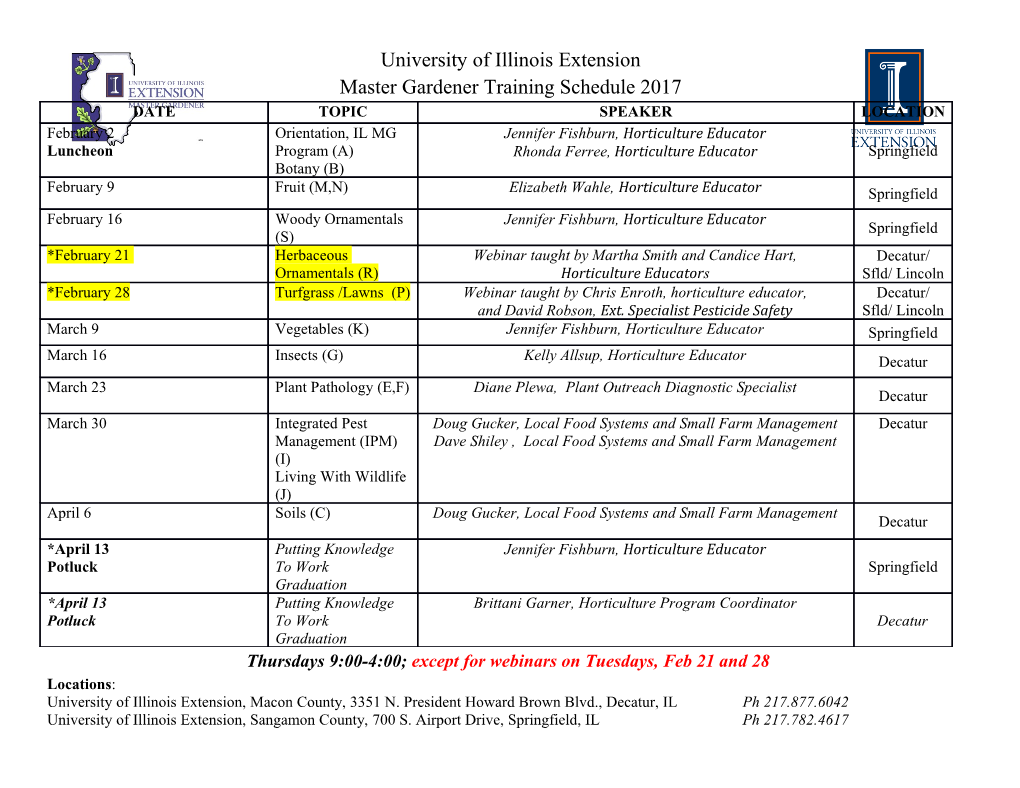
Hindawi Journal of Advanced Transportation Volume 2019, Article ID 6830450, 15 pages https://doi.org/10.1155/2019/6830450 Research Article Data-Driven Approaches to Mining Passenger Travel Patterns: (Left-Behinds) in a Congested Urban Rail Transit Network Xing Chen,1 Leishan Zhou ,1 Zixi Bai ,1 Yixiang Yue,1 Bin Guo ,2 and Hanxiao Zhou1 1 Department of Transportation Management Engineering, School of Trafc and Transportation, Beijing Jiaotong University, China 2National Research Center of Rail Transit and Transportation Training and Accreditation, Beijing Jiaotong University, China Correspondence should be addressed to Zixi Bai; [email protected] Received 20 November 2018; Accepted 6 March 2019; Published 1 April 2019 Academic Editor: Eneko Osaba Copyright © 2019 Xing Chen et al. Tis is an open access article distributed under the Creative Commons Attribution License, which permits unrestricted use, distribution, and reproduction in any medium, provided the original work is properly cited. Te “lef-behind” phenomenon occurs frequently in Urban Rail Transit (URT) networks with booming travel demand, especially during peak hours in a complex URT network, which makes passenger travel patterns more complicated. Tis paper proposes a methodology to mine passenger travel patterns based on fare transaction records from automatic fare collection (AFC) systems and Automatic Vehicle Location (AVL) data from Communication Based Train Control (CBTC) Systems or tracking systems. By introducing the concept of a sequence, a space-time-sequence trajectory model is proposed to simulate a passenger’s travel activities, including when they are lef-behind. Te paper analyzes passenger travel trajectory links and estimates the weight of each feasible trajectory under tap-in/tap-out constraints. Te station time parameters, including access/egress and transfer walking- time parameters, are important inputs for the model. Te paper also presents a maximum-likelihood approach to estimate these parameters from AFC transaction data and AVL data. Te methodology is applied to a case study using AFC and AVL data from the Beijing URT network during peak hours to test the proposed model and algorithm. Te estimation results are consistent with the results obtained from the authorities, and this fnding verifes the feasibility of our approach. 1. Introduction that we term “lef-behind”; some passengers fail to board the frst departing train afer their arrival at a platform and During the last decade, Urban Rail Transit (URT) in Main- must wait for a later one. Tis occurs mainly because the land China has developed from a total system length of only travel demand exceeds the network supply during a given 763 kilometers ten years ago to 5033 kilometers by the end operational time interval due to train vehicle capacity. of 2017 [1]. With the rapid development of the URT network, To address the above problems, numerous methods travel demand has also experienced a booming increase. In have been proposed and adopted from both the operator’s the past 10 years, the average daily passenger trafc of the perspective and the passenger’s perspective. Guan (2013) [4] Beijing URT system has increased from 1.92 million in 2007 and Yu et al. (2015) [5] developed a model for network design to 10.35 million in 2017, an increase of 439% [2]. Te Mass with the objective of minimizing the number of transfers. Transit Railway system (MTR) in Hong Kong has increased Niu and Zhou [6] presented a methodology to optimize the approximately 131.6% in patronage since 2006 [3]. timetable to minimize waiting time under time-dependent Te signifcant increase in travel demand has resulted travel demands and oversaturation. Tey analyzed the char- in congestion and overcrowding both in stations and in acteristics of passenger fow and formulated a model to train vehicles; this has become a serious problem for URT minimize passengers’ waiting times or minimize the number operators to address, particularly during peak hours. On one of transfers. To better grasp the distribution of URT network hand, congestion brings security risks. On the other hand, passenger fow, methodologies to study passengers’ travel congestion and overcrowding reduce the attractiveness of the patterns have been developed. Tese facilitate a number of URT network, and some passengers will choose other modes applications, including (i) analysis of passengers’ path-choice of transportation. Additionally, a new phenomenon appears preferences, such as minimum time and minimum number 2 Journal of Advanced Transportation of transfers, (ii) prediction of individual passengers’ locations followed by the introduction of the solution algorithm in Sec- and the future distribution of URT network passenger fow, tion 5. Section 6 presents a numerical experiment on a real- (iii) optimizing train scheduling both from the subway line worldnetwork.Tefnalsectionprovidesourconclusions level by identifying the most congested stations and sections and suggestions for future research directions. and from the network level by identifying the transfer hot-spots, and (iv) guiding passengers to avoid congested 2. Literature Review sections as much as possible by informing route suggestions, congestion levels, etc. Many scholars and researchers have studied URT network Tus, this paper attempts to mine passenger travel pat- passenger travel patterns during the last decades. At the terns based on automatic fare collection (AFC) transaction beginning of these studies, it was generally not possible to data and Automatic Vehicle Location (AVL) data. It builds obtain bulk data, including passengers’ tap-in/tap-out infor- on our prior work on the problem [7] and reconstructs a pas- mationandactualtrainmovementdata.Becauseofthelackof senger’s trajectory by introducing the concept of a sequence data, numerous methods were proposed at macroscale level. to describe lef-behind. Te prior work ignored train vehicle Tese methodologies mainly analyzed passengers’ travel pat- capacity constraints and assumed that all passengers lef a terns from a network-fow perspective. Tey generated sets of platform for another subway station by the frst departing feasible paths for each origin-destination (OD) and assigned train afer their arrival at the platform. We propose the passenger fows to each path following specifc principles. concept of sequence to describe the relationships between Te three most well-known principles are the all-or-nothing passenger’s arrival and departure of trains. By separating time principle, the stochastic-assignment principle [8–12], and the periods into segments according to stations, train directions, user-equilibrium-assignment principle [13–18]. and the departure times of trains passing stations, this paper With the wide adoption of AFC systems and rapid reconstructs a passenger’s trajectory and proposes a space- development of train tracking systems such as the Com- time-sequence trajectory model. Te model generates a set munication Based Train Control (CBTC) System, massive of feasible space-time-sequence trajectories that indicate a detaileddatawascollectedandsavedtodatabases.Most passenger’s precise travel patterns and the expected number AFC systems record passenger tap-in/tap-out information of times a passenger is lef-behind on a platform. Ten, a accurately except for some cases such as the New York methodology is presented to estimate the number of lef- City Transit Authority (NYCT) system, in which exit swipe behinds and the probability of each trajectory based on information is not recorded. Te Automatic Vehicle Location the distribution of station access/egress walking time and (AVL) system records train arrival and departure times at sta- transfer walking time. Tese distributions can be obtained tions accurately and in detail. With bulk data collected daily from manual surveys. However, these require substantial automatically and continuously, some novel methodologies labor. Te paper presents a maximum-likelihood estimation for transit performance [19–21] and management [6, 22, 23] methodology based on passengers’ trajectories. have been developed. Te main contributions of this study include the follow- Dai (2015) [22] presented a multimodal evacuation model ing: for metro disruptions based on AFC data in Shanghai, China. (i) A space-time-sequence trajectory model to simulate a Using AFC data of stations in urban areas of Hong Kong, passenger’s travel itinerary in a congested URT system. Te Wang (2015) [21] developed a methodology to analyze metro model introduces the concept of sequence and provides a trip patterns at an aggregate level. Kusakabe and Asakura means to indicate the lef-behind phenomenon. (2014) [24] estimated passengers’ behavioral attributes of (ii) A maximum-likelihood estimation methodology trips with a data fusion methodology using smart cards. based on AFC and AVL data that estimates passenger’s Tey observed and compared continuous long-term changes travel patterns. More automatic data instead of empirical in passengers’ trips as well as personal trip survey data data or manual survey data is used in the methodology; this and constructed a Bayes probabilistic model to estimate minimizes the occasional deviation caused by human factors. the purposes of passengers’ trips. Jin (2015) [19] evaluated Additionally, it reduces the difculty of obtaining data. transit service performance by developing a data-mining (iii) A data-driven method to estimate station walking- logic methodology based on transit smart-card data.
Details
-
File Typepdf
-
Upload Time-
-
Content LanguagesEnglish
-
Upload UserAnonymous/Not logged-in
-
File Pages16 Page
-
File Size-