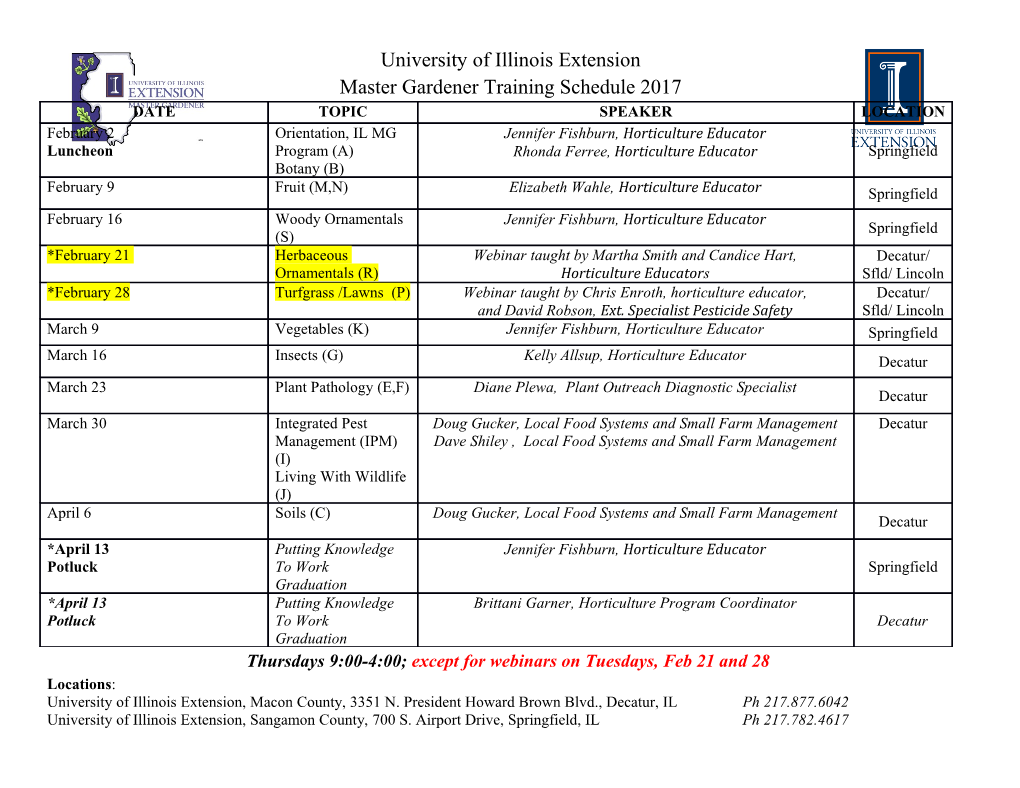
ARTICLE Received 9 Sep 2016 | Accepted 12 Dec 2016 | Published 31 Jan 2017 DOI: 10.1038/ncomms14249 OPEN Pancancer modelling predicts the context-specific impact of somatic mutations on transcriptional programs Hatice U. Osmanbeyoglu1, Eneda Toska2, Carmen Chan2, Jose´ Baselga2 & Christina S. Leslie1 Pancancer studies have identified many genes that are frequently somatically altered across multiple tumour types, suggesting that pathway-targeted therapies can be deployed across diverse cancers. However, the same ‘actionable mutation’ impacts distinct context-specific gene regulatory programs and signalling networks—and interacts with different genetic backgrounds of co-occurring alterations—in different cancers. Here we apply a computational strategy for integrating parallel (phospho)proteomic and mRNA sequencing data across 12 TCGA tumour data sets to interpret the context-specific impact of somatic alterations in terms of functional signatures such as (phospho)protein and transcription factor (TF) activities. Our analysis predicts distinct dysregulated transcriptional regulators downstream of somatic alterations in different cancers, and we validate the context-specific differential activity of TFs associated to mutant PIK3CA in isogenic cancer cell line models. These results have implications for the pancancer use of targeted drugs and potentially for the design of combination therapies. 1 Computational and Systems Biology Program, Memorial Sloan Kettering Cancer Center, 1275 York Avenue, Box No. 460, New York, New York 10065, USA. 2 Human Oncogenesis and Pathogenesis Program, Memorial Sloan Kettering Cancer Center, New York, New York 10065, USA. Correspondence and requests for materials should be addressed to C.S.L. (email: [email protected]). NATURE COMMUNICATIONS | 8:14249 | DOI: 10.1038/ncomms14249 | www.nature.com/naturecommunications 1 ARTICLE NATURE COMMUNICATIONS | DOI: 10.1038/ncomms14249 ancer cells evolve to acquire hallmark capabilities to therapeutics—and potentially for the development of context- sustain chronic proliferation, evade growth suppressors specific combination therapies—in a pancancer setting. Cand avoid cell death1 largely through the accumulation of somatic alterations that disrupt key signalling pathways. Large-scale cancer genomics projects such as The Cancer Results Genome Atlas (TCGA) have generated a comprehensive Pancancer analysis models dysregulated TFs and signalling. catalogue of somatic mutations and copy number aberrations We used a computational strategy for exploiting parallel across many tumour types. These alterations have been (phospho)proteomic and transcriptomic data to learn a model mapped to known pathways with the hope of deploying that links alterations in signalling (from RPPA data) pathway-targeted therapeutics—drugs targeting mutant with downstream changes transcriptional response (measured by oncoproteins or highly overexpressed wild-type (WT) receptors mRNA data) via predicted TF binding sites15 (Fig. 1a–c). or signal-transduction proteins—for personalized medicine2–11. We used a regularized bilinear regression algorithm called However, while the same ‘actionable mutation’ may occur in affinity regression (AR)16 to learn an interaction matrix multiple cancers, it interacts with context-specific regulatory between upstream signal-transduction proteins and downstream and signalling networks as well as the genetic background TFs that predicts target gene expression (Fig. 1a, bottom). More of other somatic alterations, suggesting that its impact—and the intuitively, the model learns weighted edges between signalling effectiveness of the targeted therapy—may strongly depend proteins and TFs to describe the flow of information from both on the cancer type and additional molecular features of change in (phospho)protein level to altered activity of TF to the individual tumour. Moreover, the role of many frequent transcriptional changes in target genes (Fig. 1a, top). In somatic alterations remains obscure, and it is unclear whether a pancancer context, an AR model is trained independently for and how they interact with targetable pathways. In fact, each cancer type and explains the variation in gene expression computational studies of drug sensitivity across cancer cell lines across tumours in terms of (phospho)protein variation and have found that gene expression features are more informative presence of TF binding sites (see Methods section). than mutations for predicting response to targeted therapies12. We can further use the trained AR interaction matrix for each Meanwhile, early ‘basket’ clinical trials that enroll patients for cancer type to obtain different views of each tumour data set targeted therapies based on mutation status alone—regardless of via mappings (Fig. 1b): given a tumour sample’s (phospho)protein cancer type—have demonstrated efficacy only in a subset of expression levels, we can multiply through the model to infer cancers13,14. These findings point to the need for better sample-specific TF activities; conversely, given the gene expres- integrative computational methods that leverage additional sion profile, we can multiply through the motif hit matrix and molecular readouts to model the context-specific impact of the model to infer ‘(phospho)protein activities’ that are somatic alterations on gene expression programs. more informative than the original noisy RPPA data (Fig. 1b, To this end, we applied a computational strategy for exploiting bottom). Intuitively, information flows down from observed parallel (phospho)proteomic and mRNA sequencing data for RPPA levels through the learned interaction matrix to infer large tumour sets by linking the dysregulation of upstream TF activities, and observed mRNA expression levels propagate up signalling pathways with altered transcriptional response through through the TF-target edges and interaction network to infer the transcriptional circuitry15,16. We then developed a statistical (phospho)protein activities (Fig. 1b, top). framework to interpret the impact of mutations and copy Importantly, by associating the presence of somatic alterations number events in terms of altered (phospho)protein and with altered regulator activities, we can gain mechanistic insight transcription factor (TF) activity. We used this strategy to train into the role of specific mutations or copy number events (phospho)protein–TF interaction models across 12 human (Fig. 1c). We perform the association analysis by using cancers in TCGA. First, we identified shared and cancer-specific regularized regression to predict each inferred TF activity roles of TF/signalling regulators across cancer types. In bladder (resp. (phospho)protein activity) individually from the full set urothelial carcinoma, renal cell clear carcinoma and uterus of frequent mutation and copy number features (Fig. 1c, bottom; endometrial carcinoma, many of the identified TF regulators were see Methods section). We then evaluate the significance of the significantly associated with survival outcome. By stratifying effect size (coefficient) for each alteration in the regression model tumours by inferred TF activities rather than gene expression by a permutation approach (see Methods section). After false patterns, we identified known and previously unlinked TFs that discovery rate (FDR) correction across TFs/(phospho)proteins, are differentially active in HPV( þ ) versus HPV( À ) head and we can identify a significant set of regulators whose altered neck squamous cancer, and we uncovered a subtype activities are associated with each mutation/copy number event of endometrioid uterine cancer harbouring mutant b-catenin while controlling for the genetic background of other alterations. with altered TF activities. We trained AR models on tumours from 12 different We next performed a systematic regularized regression analysis TCGA cancer studies using samples for which mRNA, RPPA, to associate frequent somatic aberrations with changes in inferred somatic mutation and copy number variation data were available: TF and (phospho)protein activities in each cancer type. bladder urothelial carcinoma (BLCA, n ¼ 115), breast cancer This analysis identified key regulators associated with the (BRCA, n ¼ 368), colorectal adenocarcinoma (COADREAD, major mutations in renal clear-cell carcinoma. More generally, n ¼ 150), glioblastoma multiforme (GBM, n ¼ 58), head and we observed that specific molecular aberrations have cancer- neck squamous carcinoma (HNSC, n ¼ 194), kidney renal cell- specific functional consequences. In particular, we associated clear carcinoma (KIRC, n ¼ 376), lung adenocarcinoma (LUAD, PIK3CA activating mutations with altered activities of distinct n ¼ 216), lung squamous cell carcinoma (LUSC, n ¼ 106), ovarian sets of TFs in different cancers. Notably, in isogenic cell carcinoma (OV, n ¼ 164), prostate cancer (PRAD, n ¼ 159), line models of breast cancer and head and neck cancer, we uterine corpus endometrial carcinoma (UCEC, n ¼ 183), and validated the altered activity of several TFs in the presence uterine carcinosarcoma (UCS, n ¼ 47). of mutant PIK3CA by measuring promoter occupancy and For statistical evaluation, we computed the mean Spearman expression of target genes, confirming the context-specific correlation between predicted and measured gene expression predictions of our model. These proof-of-principle results suggest profiles on held-out samples using 10-fold cross-validation for a computational strategy for personalized deployment of targeted each cancer model. We obtained
Details
-
File Typepdf
-
Upload Time-
-
Content LanguagesEnglish
-
Upload UserAnonymous/Not logged-in
-
File Pages14 Page
-
File Size-