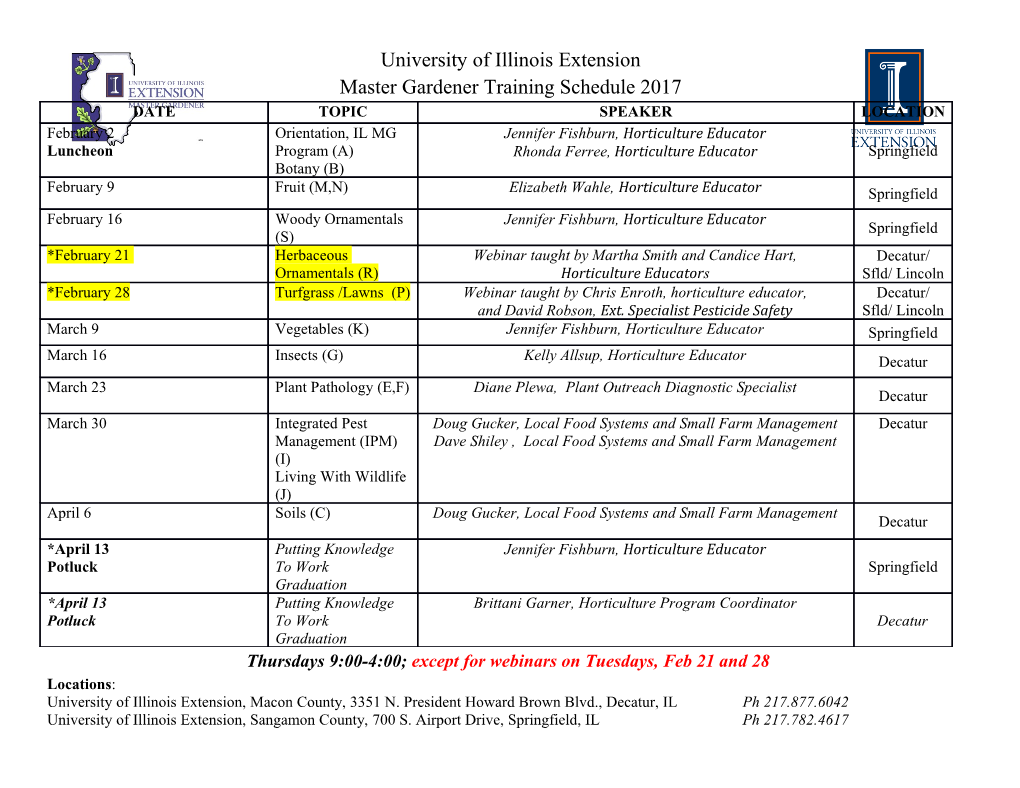
DIALECTAL ARABIC PROCESSING USING DEEP LEARNING Inaugural-Dissertation zur Erlangung des Doktorgrades der Philosophie (Dr. phil.) durch die Philosophische Fakultät der Heinrich-Heine-Universität Düsseldorf vorgelegt von Younes Samih aus essen Betreuerin: Prof. Dr. Laura Kallmeyer Düsseldorf, Oktober 2017 ABSTRACT In the last few years, the advent of the phenomena of social media and the ubiquity of the internet access have created an unprecedented deluge of in- formation and textual data on the world wide web. This data brings in its wake new opportunities and poses many challenges for machine learning and Natural Language Processing (NLP) in particular. The sheer size, non- standard spelling, the poor quality, the informality, and the noise of this data, presents new challenges to standard NLP tools developed for traditional data. To make sense out of such data and exploit its value, novel NLP methods, resources, and efficient algorithms beyond rule-based deductive reasoning and "traditional" system engineering need to be created. Given the ground breaking results of Deep Learning (DL) models in solving hard natural lan- guage processing tasks, I argue in this dissertation that these models are well suited for processing social Media textual data. A case in point is Dialectal Arabic (DA) which is emerging as the language of informal communication on the web, in emails, Social Media platforms, blogs, etc. To systematically investigate the ability of DL models to process the less controlled and more speech-like nature of DA in Social Media, I choose to address two concrete, challenging tasks, namely linguistic Code-Switching (CS) identification and DA morphological segmentation. ii ZUSAMMENFASSUNG In den letzten Jahren ist durch die sozialen Medien und allgegenwärtigen Zugang zum Internet eine noch nie dagewesene Flut von Information und Textdaten im Internet entstanden. Diese Daten bringen neue Chancen und vielfältige Herausforderungen im Bereich des machinellen Lernens, und in- besondere in der maschinellen Sprachverarbeitung NLP. Die schiere Menge, nicht den gewohnten Normen entsprechende Rechtschreibung, die schlechte Qualität und Informalität, und das Rauschen in den Daten stellen die NLP- Standardwerkzeuge, die auf traditionellen Daten entwickelt worden sind, vor neue Probleme. Um solche Daten interpretieren und ihren Wert nutzen zu können, müs- sen neue NLP-Methoden, Resourcen und effiziente Algorithmen jenseits von regelbasiertem dekutiven Herangehensweisen und "traditionellen"Methoden der Systementwicklung geschaffen werden. Im Licht der bahnbrechenden Er- gebnisse von Modellen des Deep Learning (DL) argumentiere ich in dieser Dissertation, dass solche Modelle gut zur Verarbeitung von Text aus sozia- len Medien geeignet sind. Als Beispielfall dient das Dialectal Arabic (DA), das sich zur Sprache der informellen Kommunikation im Web, in E-Mails, auf sozialen Medien, und in Blogs entwickelt hat. Um die Möglichkeiten von DL-Modellen zu zeigen, das weniger kontrollierte und der gesproche- nen Sprache ähnliche DA in sozialen Medien zu verarbeiten, bearbeite ich zwei konkrete, herausfordernde Probleme, und zwar die Identifikation von linguistischem Code-Switching (CS) und die morphologische Segmentierung von DA. iii AUTHOR STATEMENT I would like here to acknowledge that Chapter 4 “Data and Resource Cre- ation” includes work that was published as Samih and Maier (2016a) and Samih and Maier (2016b). I was primarily responsible for the design and creation of the corpus described in these two papers. I was also an active col- laborator throughout the creation of the multi-dialectal segmentation corpus presented in the following papers, Samih et al. (2017a), Samih et al. (2017b) Samih, Maier, and Kallmeyer (2016), and Eldesouki et al. (2017). Chapter 5 “Code-switching identification” also includes work that was pub- lished as Samih and Maier (2016a,b) and Samih, Maier, and Kallmeyer (2016). I was primarily responsible all the experiments and the design and creation of the corpus used in this chapter. The other authors contributed to these papers primarily in an advisory role. In addition, Section 5.5 in this chapter presents work that is excerpted from Samih et al. (2016), which also forms the basis for much of this chapter. I was responsible for the design of the neural models presented in that paper. Chapter 6 “Dialectal Arabic Segmentation” also includes work that was pub- lished as Eldesouki et al. (2017) and Samih et al. (2017a,b). I was responsi- ble for the design of the neural models presented in these three papers. I substantially contributed to the implementation and testing of these models and in the development of the segmentation algorithm. Eldesouki Mohamed was responsible for the implementation of the Support Vector Machine (SVM) models. The other authors contributed to these papers primarily in an advi- sory role. iv Appendix A “SAWT” is an updated version of my paper titled “SAWT: Se- quence Annotation Web Tool” presented in the following paper Samih, Maier, and Kallmeyer (2016). v ACKNOWLEDGMENTS I owe an enormous debt of gratitude to my supervisor, Laura Kallmeyer, for many reasons. First she put me in the right track since Day One saving me a lot of time and effort exploring uncountable paths. Second she kept motivating and supporting me along the way. She encouraged me to write papers, and attend meetings and conferences. She also provided the financial funds needed for travelling to these research events. Third, at times when I was about to go off rail or lose confidence, her advice helped put me back in the right track. My deepest gratitude goes to my affectionate mother and my dearest father, for everything they have provided, and to my family (my wife Ikram and my son Adam), to whom this dissertation is dedicated, for their love, patience and unfailing support. Mohammed Attia has been my post-doc mentor even before my dissertation. I am so thankful for all the detailed comments he has made during all these years. He has always been willing to discuss various aspect of my dissertation or read a chapter. I am really grateful for his support. I am grateful that I could finish my work at the University of Düsseldorf among nice and supportive colleagues. In particular I would like to thank Wolfgang Maier and Miriam Kaeshammer. I would also like to thank James Kilbury, Kareem Darwish, Ahmed Abde- lali, Hamdy Mubarak, Mohamed Eldesouki, Timm Lichte, Christian Wurm, Simon Petitjean, and Andreas van Cranenburgh for discussing various as- vi pects of my dissertation with me and for giving me insightful comments. All remaining errors are my own! vii CONTENTS i introduction 1 1 introduction 2 1.1 Overview and Motivation ...................... 2 1.2 Contributions of this Dissertation ................. 4 1.3 Dissertation Overview ........................ 6 ii background 7 2 arabic and its dialects 8 2.1 The Arabic Language Varieties ................... 8 2.2 Linguistic Characteristics of the Arabic Dialects ......... 12 2.2.1 Morphology .......................... 12 2.2.2 Syntax ............................. 14 2.3 Processing of Dialectal Arabic .................... 15 2.4 Challenges of Processing Dialectal Arabic ............. 17 2.5 Concluding Remarks ......................... 19 3 deep learning background 20 3.1 Introduction .............................. 20 3.2 Basics of Neural Networks ..................... 23 3.2.1 Mathematical Notation ................... 23 3.2.2 The Artificial Neuron .................... 23 3.2.3 Multi-Layer Neural Networks ............... 25 3.3 Recurrent Neural Networks ..................... 27 3.4 Deep Neural Networks for NLP .................. 30 3.5 Concluding Remarks ......................... 31 viii contents ix iii resource creation for dialctal arabic 32 4 data and resource creation 33 4.1 Introduction .............................. 33 4.2 The Moroccan Code-switching Corpus .............. 35 4.2.1 Data Collection ........................ 35 4.2.2 Annotation .......................... 36 4.2.3 Related Work ......................... 40 4.3 The Multi-Dialectal Segmentation Corpus ............ 41 4.3.1 Data Creation ......................... 41 4.3.2 Annotation .......................... 42 4.4 Concluding Remarks ......................... 46 iv applictaions 47 5 code-switching identification 48 5.1 Introduction .............................. 48 5.2 Code-Switching ............................ 50 5.2.1 A Linguistic Analysis of Code-Switching ......... 50 5.2.2 Code-Switching in Morocco ................ 52 5.3 Related Work ............................. 54 5.4 Dataset ................................. 56 5.5 Code-switching Detection Approaches .............. 57 5.5.1 CRF Approch (Baseline) ................... 58 5.5.2 A Deep Neural Network Model .............. 63 5.6 Results and Experiments ....................... 66 5.7 Analysis ................................ 68 5.7.1 What is Captured in Char-Word Representations? . 68 5.7.2 CRF Model .......................... 70 5.7.3 Error Analysis ........................ 71 contents x 5.8 Concluding Remarks ......................... 74 6 dialectal arabic segmentation 75 6.1 Introduction .............................. 75 6.2 related work .............................. 76 6.3 Segmentation Datasets ........................ 78 6.4 Arabic Dialects ............................ 78 6.4.1 Similarities Between Dialects................ 78 6.4.2 Differences Between Dialects ................ 81 6.5 Learning Algorithms ......................... 85 Rank 6.5.1 SVM Approach
Details
-
File Typepdf
-
Upload Time-
-
Content LanguagesEnglish
-
Upload UserAnonymous/Not logged-in
-
File Pages147 Page
-
File Size-