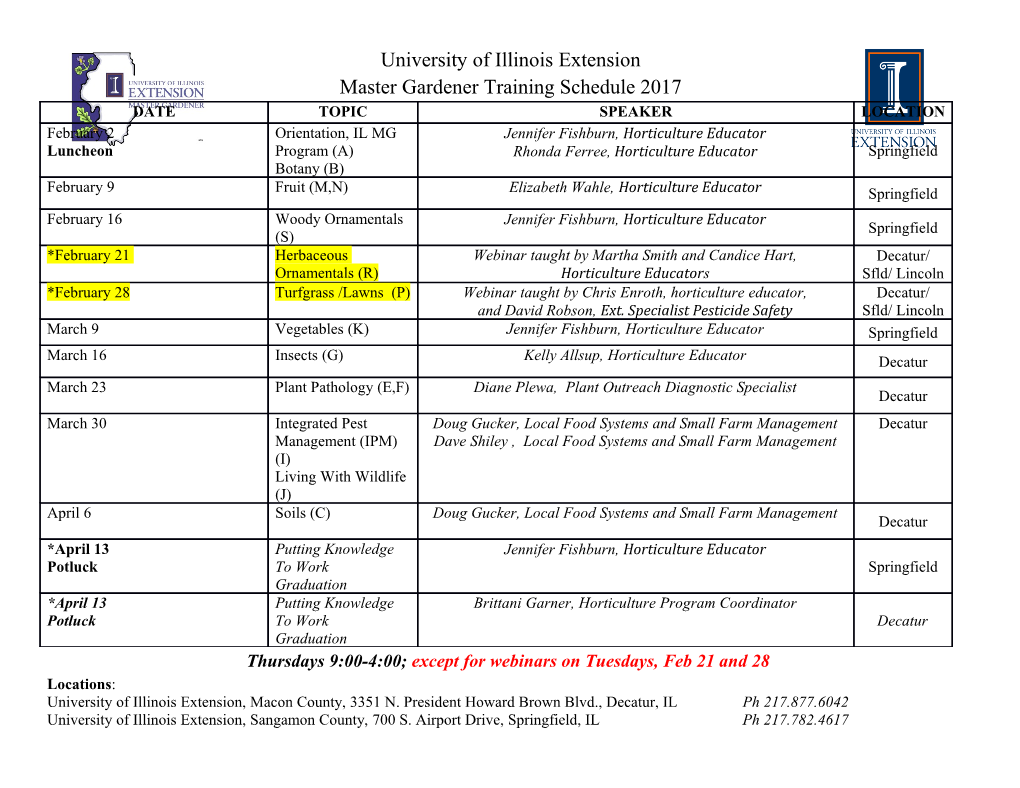
A Brief Introduction to Bayesian Statistics Bayes’ Theorem Priors Computation David Kaplan Bayesian Hypothesis Testing Department of Educational Psychology Bayesian Model Building and Evaluation The Bayesian Advantage GESIS, Mannheim, Germany, 8 November, 2017 1 / 37 The Reverend Thomas Bayes, 1701–1761 Bayes’ Theorem Priors Computation Bayesian Hypothesis Testing Bayesian Model Building and Evaluation The Bayesian Advantage 2 / 37 Pierre-Simon Laplace, 1749–1827 Bayes’ Theorem Priors Computation Bayesian Hypothesis Testing Bayesian Model Building and Evaluation The Bayesian Advantage 3 / 37 Bayes’ Theorem Priors Computation Bayesian Hypothesis Testing Bayesian Model Building and Evaluation . The Bayesian Advantage [I]t is clear that it is not possible to think about learning from experience and acting on it without coming to terms with Bayes’ theorem. - Jerome Cornfield 4 / 37 Bayesian statistics has long been overlooked in the quantitative methods training of social scientists. Bayes’ Theorem Typically, the only introduction that a student might have to Priors Bayesian ideas is a brief overview of Bayes’ theorem while Computation studying probability in an introductory statistics class. Bayesian Hypothesis Testing 1 Until recently, it was not feasible to conduct statistical modeling Bayesian Model from a Bayesian perspective owing to its complexity and lack of Building and Evaluation availability. The Bayesian 2 Advantage Bayesian statistics represents a powerful alternative to frequentist (classical) statistics, and is therefore, controversial. Bayesian statistical methods are now more popular owing to the development of powerful statistical software tools that make the estimation of complex models feasible from a Bayesian perspective. 5 / 37 Outline Major differences between the Bayesian and frequentist Bayes’ paradigms of statistics Theorem Priors Computation Bayes’ Theorem Bayesian Hypothesis Testing Priors Bayesian Model Building and Evaluation Computation The Bayesian Advantage Bayesian hypothesis testing Bayesian model building and evaluation Debates within the Bayesian school. 6 / 37 Paradigm Difference I: Conceptions of Probability For frequentists, the basic idea is that probability is represented Bayes’ Theorem by the model of long run frequency. Priors Computation Frequentist probability underlies the Fisher and Bayesian Hypothesis Neyman-Pearson schools of statistics – the conventional Testing methods of statistics we most often use. Bayesian Model Building and Evaluation The frequentist formulation rests on the idea of equally probable The Bayesian and stochastically independent events Advantage The physical representation is the coin toss, which relates to the idea of a very large (actually infinite) number of repeated experiments. 7 / 37 Bayes’ The entire structure of Neyman - Pearson hypothesis testing Theorem Priors and Fisherian statistics (together referred to as the frequentist Computation school) is based on frequentist probability. Bayesian Hypothesis Testing Our conclusions regarding null and alternative hypotheses Bayesian Model presuppose the idea that we could conduct the same Building and Evaluation experiment an infinite number of times. The Bayesian Advantage Our interpretation of confidence intervals also assumes a fixed parameter and CIs that vary over an infinitely large number of identical experiments. 8 / 37 But there is another view of probability as subjective belief. The physical model in this case is that of the “bet”. Bayes’ Theorem Priors Consider the situation of betting on who will win the the World Computation Cup (or the World Series). Bayesian Hypothesis Testing Here, probability is not based on an infinite number of Bayesian repeatable and stochastically independent events, but rather on Model Building and how much knowledge you have and how much you are willing Evaluation to bet. The Bayesian Advantage Subjective probability allows one to address questions such as “what is the probability that my team will win the World Cup?” Relative frequency supplies information, but it is not the same as probability and can be quite different. This notion of subjective probability underlies Bayesian statistics. 9 / 37 Bayes’ Theorem Bayes’ Consider the joint probability of two events, Y and X, for Theorem example observing lung cancer and smoking jointly. Priors Computation Bayesian The joint probability can be written as Hypothesis Testing . Bayesian Model Building and p(cancer; smoking) = p(cancerjsmoking)p(smoking) (1) Evaluation The Bayesian Similarly, Advantage . p(smoking; cancer) = p(smokingjcancer)p(cancer) (2) 10 / 37 Because these are symmetric, we can set them equal to each other to obtain the following Bayes’ Theorem . Priors Computation Bayesian p(cancerjsmoking)p(smoking) = p(smokingjcancer)p(cancer) (3) Hypothesis Testing Bayesian . Model Building and p(smokingjcancer)p(cancer) Evaluation p(cancerjsmoking) = (4) The p(smoking) Bayesian Advantage The inverse probability theorem (Bayes’ theorem) states . p(cancerjsmoking)p(smoking) p(smokingjcancer) = (5) p(cancer) 11 / 37 Statistical Elements of Bayes’ Theorem What is the role of Bayes’ theorem for statistical inference? Bayes’ Theorem Denote by Y a random variable that takes on a realized value y. Priors Computation Bayesian Hypothesis For example, a person’s socio-economic status could be Testing Bayesian considered a random variable taking on a very large set of Model Building and possible values. Evaluation The Bayesian Once the person identifies his/her socioeconomic status, the Advantage random variable Y is now realized as y. Because Y is unobserved and random, we need to specify a probability model to explain how we obtained the actual data values y. 12 / 37 Next, denote by θ a parameter that we believe characterizes the Bayes’ probability model of interest. Theorem Priors Computation The parameter θ can be a scalar, such as the mean or the Bayesian variance of a distribution, or it can be vector-valued, such as a Hypothesis Testing set of regression coefficients in regression analysis or factor Bayesian loadings in factor analysis. Model Building and Evaluation The We are concerned with determining the probability of observing Bayesian Advantage y given the unknown parameters θ, which we write as p(yjθ). In statistical inference, the goal is to obtain estimates of the unknown parameters given the data. 13 / 37 Paradigm difference II: Nature of parameters The key difference between Bayesian statistical inference and frequentist statistical inference concerns the nature of the Bayes’ unknown parameters θ. Theorem Priors θ Computation In the frequentist tradition, the assumption is that is unknown, Bayesian but that estimation of this parameter does not incorporate Hypothesis Testing uncertainty. Bayesian Model Building and In Bayesian statistical inference, θ is considered unknown and Evaluation random, possessing a probability distribution that reflects our The Bayesian uncertainty about the true value of θ. Advantage Because both the observed data y and the parameters θ are assumed random, we can model the joint probability of the parameters and the data as a function of the conditional distribution of the data given the parameters, and the prior distribution of the parameters. 14 / 37 More formally, Bayes’ . Theorem Priors p(θ; y) = p(yjθ)p(θ): (6) Computation Bayesian Hypothesis where p(θ; y) is the joint distribution of the parameters and the Testing data. Following Bayes’ theorem described earlier, we obtain Bayesian Model Building and Evaluation The Bayes’ Theorem Bayesian Advantage p(yjθ)p(θ) p(θjy) = / p(yjθ)p(θ); (7) p(y) where p(θjy) is referred to as the posterior distribution of the parameters θ given the observed data y. 15 / 37 Bayes’ Theorem Equation (7) represents the core of Bayesian statistical Priors Computation inference and is what separates Bayesian statistics from Bayesian frequentist statistics. Hypothesis Testing Bayesian Equation (7) states that our uncertainty regarding the Model Building and parameters of our model, as expressed by the prior distribution Evaluation The p(θ), is weighted by the actual data p(yjθ) yielding an updated Bayesian Advantage estimate of our uncertainty, as expressed in the posterior distribution p(θjy). 16 / 37 Priors Bayesian statistics requires that prior distributions be specified for ALL model parameters. Bayes’ Theorem Priors There are three classes of prior distributions: Computation Bayesian 1 Hypothesis Non-informative priors Testing Bayesian Model We use these to quantify actual or feigned ignorance. Lets the data Building and maximally speak. Evaluation The Bayesian 2 Weakly informative priors Advantage Allows some weak bounds to be placed on parameters. 3 Informative priors Allows cumulative information to be considered. 17 / 37 MCMC The increased popularity of Bayesian methods in the social and behavioral sciences has been the (re)-discovery of numerical Bayes’ Theorem algorithms for estimating the posterior distribution of the model Priors parameters given the data. Computation Bayesian It was virtually impossible to analytically derive summary Hypothesis Testing measures of the posterior distribution, particularly for complex Bayesian models with many parameters. Model Building and Evaluation Instead of analytically solving for estimates of a complex The Bayesian posterior distribution, we can instead draw samples from p(θjy) Advantage and summarize the distribution formed by those samples. This is referred to as Monte Carlo integration. The two most popular
Details
-
File Typepdf
-
Upload Time-
-
Content LanguagesEnglish
-
Upload UserAnonymous/Not logged-in
-
File Pages38 Page
-
File Size-