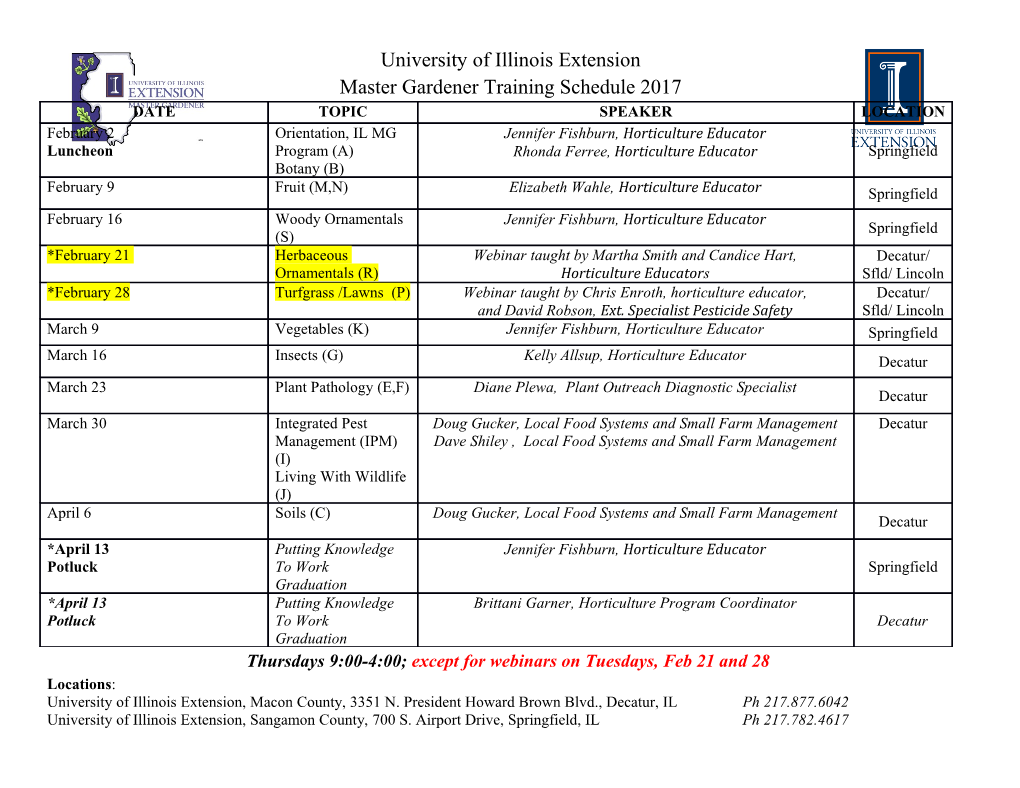
Iowa State University Capstones, Theses and Retrospective Theses and Dissertations Dissertations 2006 Applications of nonparametric regression in survey statistics Xiaoxi Li Iowa State University Follow this and additional works at: https://lib.dr.iastate.edu/rtd Part of the Statistics and Probability Commons Recommended Citation Li, Xiaoxi, "Applications of nonparametric regression in survey statistics " (2006). Retrospective Theses and Dissertations. 3055. https://lib.dr.iastate.edu/rtd/3055 This Dissertation is brought to you for free and open access by the Iowa State University Capstones, Theses and Dissertations at Iowa State University Digital Repository. It has been accepted for inclusion in Retrospective Theses and Dissertations by an authorized administrator of Iowa State University Digital Repository. For more information, please contact [email protected]. Applications of nonparametric regression in survey statistics by Xiaoxi Li A thesis submitted to the graduate faculty in partial fulfillment of the requirements for the degree of DOCTOR OF PHILOSOPHY Major: Statistics Program of Study Committee: Jean D. Opsomer, Major Professor Song X. Chen Barry L. Falk Wayne A. Fuller Michael D. Larsen Iowa State University Ames, Iowa 2006 Copyright c Xiaoxi Li, 2006. All rights reserved. UMI Number: 3243537 UMI Microform 3243537 Copyright 2007 by ProQuest Information and Learning Company. All rights reserved. This microform edition is protected against unauthorized copying under Title 17, United States Code. ProQuest Information and Learning Company 300 North Zeeb Road P.O. Box 1346 Ann Arbor, MI 48106-1346 ii To my family iii TABLE OF CONTENTS CHAPTER 1 Model-based variance estimation for systematic sampling 1 1.1 Systematic sampling . 1 1.2 Variance estimation for systematic sampling . 3 1.3 Variance estimators under linear regression models . 6 1.4 Variance estimators under nonparametric models . 16 1.5 Simulation Study . 32 1.6 Simulation conclusions . 37 CHAPTER 2 Applications of model-based nonparametric variance estimator in Forest Inventory and Analysis (FIA) . 49 2.1 Introduction . 49 2.2 Variance estimation . 50 2.3 Conclusions . 55 CHAPTER 3 Model averaging in survey estimation . 74 3.1 Regression estimator . 74 3.2 Model averaging . 77 3.3 Simulation setup . 82 3.4 Simulation results . 84 3.5 Simulation conclusions . 90 APPENDIX A Theorems, Corollaries and Lemmas for Chapter 1 . 116 APPENDIX B Using R to calculate αk’s in Chapter 3 . 121 iv BIBLIOGRAPHY . 124 v LIST OF TABLES Table 1.1 Systematic selection of n from population of N = nk elements. Same location b is selected from n rows. 2 Table 1.2 Relative biases when populations are sorted by x . 40 Table 1.3 Relative biases when populations are sorted by z1 . 41 Table 1.4 Relative biases when populations are sorted by z2 . 42 Table 1.5 Comparison of MSEs when populations are sorted by x . 43 Table 1.6 Comparison of MSEs when populations are sorted by z1 . 44 Table 1.7 Comparison of MSEs when populations are sorted by z2 . 45 Table 1.8 Comparison of MSPEs when populations are sorted by x . 46 Table 1.9 Comparison of MSPEs when populations are sorted by z1 . 47 Table 1.10 Comparison of MSPEs when populations are sorted by z2 . 48 Table 2.1 Mean and variance estimates under model (2.1) for FIA data . 52 Table 2.2 Mean and variance estimates under model (2.2) for FIA data . 53 Table 2.3 Mean and variance estimates under model (2.2) for FIA data. Different span for each predictor variable . 54 Table 3.1 List of population functions . 83 Table 3.2 List of case IDs and corresponding descriptions. 84 Table 3.3 Relative Bias, SRS design, R2 = 0.75 and n = 500 . 92 Table 3.4 Relative Bias, SRS design, R2 = 0.25 and n = 500 . 92 Table 3.5 Relative Bias, SRS design, R2 = 0.75 and n = 100 . 93 vi Table 3.6 Relative Bias, SRS design, R2 = 0.25 and n = 100 . 93 Table 3.7 Relative Bias, STSRS design, R2 = 0.75 and n = 500 . 94 Table 3.8 Relative Bias, STSRS design, R2 = 0.25 and n = 500 . 94 Table 3.9 Relative Bias, STSRS design, R2 = 0.75 and n = 100 . 95 Table 3.10 Relative Bias, STSRS design, R2 = 0.25 and n = 100 . 95 Table 3.11 Relative MSE, SRS design, R2 = 0.75 and n = 500 . 96 Table 3.12 Relative MSE, SRS design, R2 = 0.25 and n = 500 . 96 Table 3.13 Relative MSE, SRS design, R2 = 0.75 and n = 100 . 97 Table 3.14 Relative MSE, SRS design, R2 = 0.25 and n = 100 . 97 Table 3.15 Relative MSE, STSRS design, R2 = 0.75 and n = 500 . 98 Table 3.16 Relative MSE, STSRS design, R2 = 0.25 and n = 500 . 98 Table 3.17 Relative MSE, STSRS design, R2 = 0.75 and n = 100 . 99 Table 3.18 Relative MSE, STSRS design, R2 = 0.25 and n = 100 . 99 Table 3.19 Model Averaging coefficients and standard errors for population ’Linear’ and SRS design . 100 Table 3.20 Model Averaging coefficients and standard errors for population ’Quadratic’ and SRS design . 101 Table 3.21 Model Averaging coefficients and standard errors for population ’Bump’ and SRS design . 102 Table 3.22 Model Averaging coefficients and standard errors for population ’Jump’ and SRS design . 103 Table 3.23 Model Averaging coefficients and standard errors for population ’Normal CDF’ and SRS design . 104 Table 3.24 Model Averaging coefficients and standard errors for population ’Exponential’ and SRS design . 105 Table 3.25 Model Averaging coefficients and standard errors for population ’Slow sine’ and SRS design . 106 vii Table 3.26 Model Averaging coefficients and standard errors for population ’Fast sine’ and SRS design . 107 Table 3.27 Model Averaging coefficients and standard errors for population ’Linear’ and STSRS design . 108 Table 3.28 Model Averaging coefficients and standard errors for population ’Quadratic’ and STSRS design . 109 Table 3.29 Model Averaging coefficients and standard errors for population ’Bump’ and STSRS design . 110 Table 3.30 Model Averaging coefficients and standard errors for population ’Jump’ and STSRS design . 111 Table 3.31 Model Averaging coefficients and standard errors for population ’Normal CDF’ and STSRS design . 112 Table 3.32 Model Averaging coefficients and standard errors for population ’Exponential’ and STSRS design . 113 Table 3.33 Model Averaging coefficients and standard errors for population ’Slow sine’ and STSRS design . 114 Table 3.34 Model Averaging coefficients and standard errors for population ’Fast sine’ and STSRS design . 115 viii LIST OF FIGURES Figure 2.1 Map of study region in northern Utah . 56 Figure 2.2 Illustration of 4-per-stratum design . 57 Figure 2.3 Contour plot of fitted BIOMASS vs. location, span=0.5 . 58 Figure 2.4 Contour plot of fitted BIOMASS vs. location, span=0.2 . 59 Figure 2.5 Contour plot of fitted BIOMASS vs. location, span=0.1 . 60 Figure 2.6 Contour plot of fitted CRCOV vs. location, span=0.1 . 61 Figure 2.7 Contour plot of fitted BA vs. location, span=0.1 . 62 Figure 2.8 Contour plot of fitted NVOLTOT vs. location, span=0.1 . 63 Figure 2.9 Contour plot of fitted FOREST vs. location, span=0.1 . 64 Figure 2.10 Contour plot of fitted BIOMASS (with elevation) vs. location, span=0.1 . 65 Figure 2.11 Contour plot of elevation vs. location. 66 Figure 2.12 Gam component plot for BIOMASS (contributed by elevation) vs. elevation, span=0.1 . 67 Figure 2.13 Gam component plot for BIOMASS (contributed by location) vs. location, span=0.1 . 68 Figure 2.14 Gam component plots for BIOMASS, span=0.1 and 0.3 . 69 Figure 2.15 Gam component plots for CRCOV, span=0.1 and 0.3 . 70 Figure 2.16 Gam component plots for BA, span=0.1 and 0.3 . 71 Figure 2.17 Gam component plots for NVOLTOT, span=0.1 and 0.3 . 72 Figure 2.18 Gam component plots for FOREST, span=0.1 and 0.3 . 73 ix ACKNOWLEGEMENTS I would like to gratefully acknowledge my adviser Dr. Jean Opsomer’s enthusiastic supervision during this work. I greatly appreciate his help for suggesting the topic and guiding me through difficulties throughout the whole time. I also thank my PhD committee members, Dr. Wayne Fuller, Dr. Song Chen, Dr. Michael Larsen and Dr. Barry Falk for investing their time on my work and give me insightful advice. I am very thankful to other faculty and staff members at Center for Survey Statistics and Methodology (CSSM) as well for their support. I am also grateful to all my friends at Iowa State University for being the surrogate family during the five years I stayed there. Finally, I am forever indebted to my parents for their love and inspiration. Without them, I could not have imagine the completion of this work. 1 CHAPTER 1 Model-based variance estimation for systematic sampling 1.1 Systematic sampling Systematic sampling is widely used in surveys of finite populations due to its ap- pealing simplicity and efficiency. If applied properly, it can reflect stratifications in the population and thus can be more precise than random sampling. Suppose that the population size is N and that the study variable is Yj ∈ <, j = 1, 2, ··· ,N. Then the population mean is N 1 X Y¯ = Y . N N j j=1 Let n denote the sample size and k = N/n denote the sampling interval. For simplicity, p we assume that N is an integral multiple of n, i.e. k is an integer. Let Xj ∈ < (j = 1, 2, ··· ,N) be vectors of p auxiliary variables, where Yj is some continuous and finite function of Xj’s.
Details
-
File Typepdf
-
Upload Time-
-
Content LanguagesEnglish
-
Upload UserAnonymous/Not logged-in
-
File Pages137 Page
-
File Size-