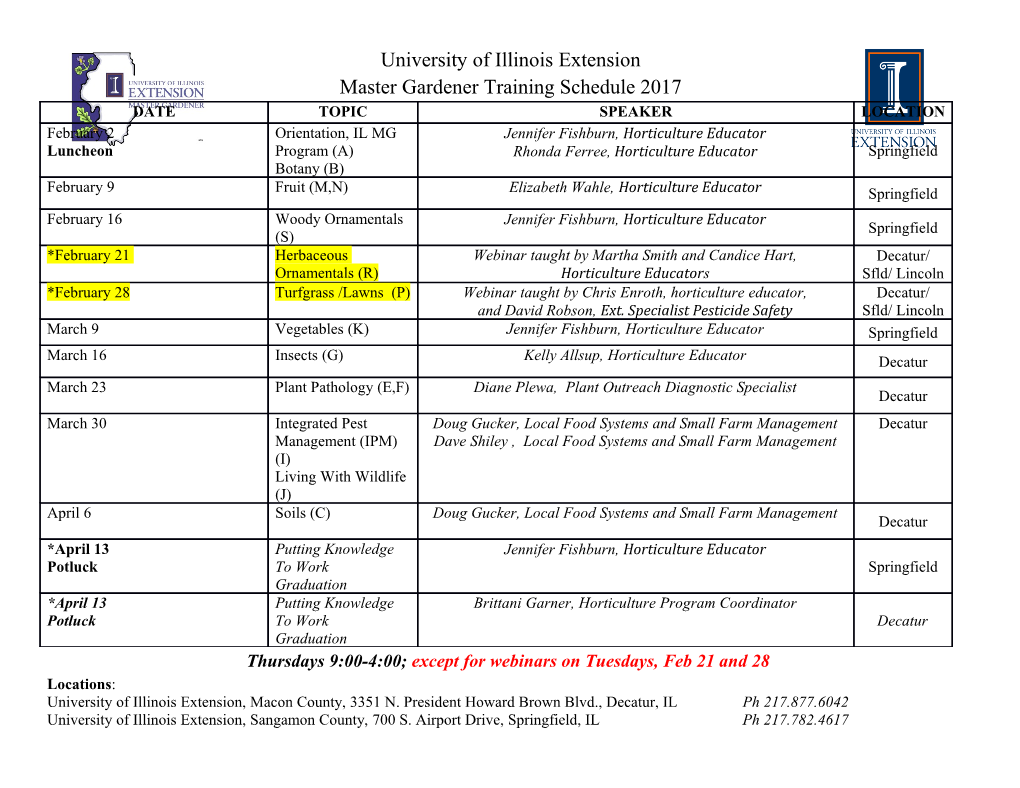
Page 1 of 32 Manuscripts submitted to Social Cognitive and Affective Neuroscience 1 2 3 4 5 6 7 8 9 10 11 12 13 14 15 Computational approaches to the neuroscience of social perception 16 17 18 19 For Peer Review 20 21 22 Jeffrey A. Brooks 23 24 New York University 25 26 27 28 29 Ryan M. Stolier 30 31 Columbia University 32 33 34 35 Jonathan B. Freeman 36 37 38 New York University 39 40 41 42 43 44 45 46 47 Corresponding author: 48 49 Jeffrey A. Brooks or Jonathan B. Freeman, Ph.D. 50 51 Department of Psychology 52 New York University 53 6 Washington Place 54 55 New York, NY 10003 56 Email: [email protected] or [email protected] 57 58 59 1 60 http://mc.manuscriptcentral.com/scan Manuscripts submitted to Social Cognitive and Affective Neuroscience Page 2 of 32 1 2 3 Abstract 4 5 Across multiple domains of social perception - including social categorization, emotion 6 7 perception, impression formation, and mentalizing - multivariate pattern analysis (MVPA) of 8 fMRI data has permitted a more detailed understanding of how social information is processed 9 10 and represented in the brain. As in other neuroimaging fields, the neuroscientific study of social 11 12 perception initially relied on broad structure-function associations derived from univariate fMRI 13 14 analysis to map neural regions involved in these processes. In this review, we trace the ways that 15 social neuroscience studies using MVPA have built on these neuroanatomical associations to 16 17 better characterize the computational relevance of different brain regions, and how MVPA 18 19 allows explicit tests of theFor correspondence Peer between Review psychological models and the neural 20 21 representation of social information. We also describe current and future advances in 22 methodological approaches to multivariate fMRI data and their theoretical value for the 23 24 neuroscience of social perception. 25 26 27 28 29 30 31 32 33 34 35 36 37 38 39 40 41 42 43 44 45 46 47 48 49 50 51 52 53 54 55 56 57 58 59 2 60 http://mc.manuscriptcentral.com/scan Page 3 of 32 Manuscripts submitted to Social Cognitive and Affective Neuroscience 1 2 3 Computational approaches to the neuroscience of social perception 4 5 Other people are tremendously complex, and humans must navigate their relationships 6 7 and interactions with others under conditions of high uncertainty. Whether meeting a stranger, 8 reading a description of someone, or trying to determine how a friend is feeling, we rely on a set 9 10 of perceptions and inferences about the person to determine our behavior. Understanding how we 11 12 form impressions about others has been a central focus in social psychology for decades, and 13 14 more recently the topic has proven to be well-suited to methods from computational 15 neuroscience, which can readily leverage the inherently high-dimensional nature of 16 17 neuroimaging data alongside behavioral measures of social perception. In this article, we review 18 19 recent advances in computationalFor approachesPeer to Reviewthe neuroscience of social perception. We focus 20 21 particularly on multivariate analyses of fMRI data, but computational analyses of behavioral data 22 used in conjunction with fMRI, such as using fMRI and behavioral responses to estimate 23 24 parameters of computational models, is an increasingly popular approach as well and is reviewed 25 26 elsewhere (e.g., Cheong et al., 2017; Gonzalez & Chang, 2019; Hackel & Amodio, 2018). 27 As in other areas of social neuroscience, early fMRI studies on social perception generally 28 29 focused on univariate activation-based analyses to associate relevant social cognitive processes 30 31 with particular brain regions, which continues to be a valuable mainstay. This research described 32 33 a number of regions important for social perception, such as the primacy of the fusiform gyrus 34 (FG) in face processing (Haxby et al., 2000; 2002; Kanwisher et al., 1997); superior temporal 35 36 sulcus (STS) in dynamic face and body perception (Grossman et al., 2000; Haxby et al., 2000; 37 38 Said et al., 2010); and regions such as the medial prefrontal cortex (MPFC; Amodio & Frith, 39 40 2006; Mitchell, 2008) and temporo-parietal junction (TPJ; Saxe & Kanwisher, 2003) in thinking 41 about others and representing their mental states (“mentalizing”). Multivariate pattern analysis 42 43 (MVPA) was first introduced to neuroimaging research by Haxby and colleagues (2001), 44 45 expanding the conceptual and methodological toolkit of neuroimaging researchers by providing a 46 different way to conceptualize the patterns of activation that emerge in fMRI data. In the present 47 48 review, we first briefly introduce MVPA techniques and discuss their theoretical basis as well as 49 50 some related advantages and limitations. We then review in turn three domains of social 51 52 perception research where these techniques have proven highly valuable: perceiving social group 53 memberships, perceiving identity and associated traits and person knowledge, and perceiving 54 55 others’ emotional states. 56 57 58 59 3 60 http://mc.manuscriptcentral.com/scan Manuscripts submitted to Social Cognitive and Affective Neuroscience Page 4 of 32 1 2 3 MVPA Approaches 4 5 Univariate approaches primarily seek to relate the overall level of activation in a region to 6 7 task conditions or experimental variables, which can provide neuroanatomical associations with 8 those conditions or variables and their related psychological processes (although with exceptions, 9 10 e.g., adaptation paradigms). However, neural regions assessed via fMRI do not only vary in their 11 12 mean level of activation, but also in the spatial patterns of activation distributed across voxels. 13 14 MVPA methods are sensitive to fine-grained differences in these spatial patterns of activation, 15 whereas mass univariate testing treats each voxel individually, almost always ignoring the level 16 17 of activation in contiguous voxels in statistical tests. This sensitivity enables MVPA to 18 19 differentiate experimentalFor conditions Peer even in cases Review where mere differences in mean activation of 20 21 a voxel cannot (Haxby et al., 2001; 2014). 22 A key assumption often made about MVPA and influencing how MVPA analyses are 23 24 generally interpreted is that neural response patterns inherently contain information about an 25 26 associated cognitive state (Davis et al., 2014; Haynes, 2015; Lewis-Peacock & Norman, 2014; 27 Popov et al., 2018). Researchers can thus probe condition response patterns to see how they may 28 29 differ between brain regions, revealing the involvement of different regions in processing 30 31 information or representing states relevant for a given task. Thus, multivariate analyses often 32 33 target regions already known to be involved in specific tasks to specify how that region is 34 representing information throughout the task and what computational processes that region may 35 36 support. Neuronal recordings in nonhuman primates have long shown that the aggregate activity 37 38 of an assembly of neurons can provide a ‘code’ for various kinds of sensory and abstract 39 40 cognitive information in the brain (i.e., a “population code”; Averbeck et al., 2006). Although 41 fMRI voxels are far too large to be sensitive to individual neurons, neurons belonging to 42 43 different neuronal assemblies (e.g., related to state 1 vs. state 2) may be distributed in different 44 45 ways such that the precise assembly of neurons related to each state may vary across voxels. This 46 could thereby give rise to distinct fMRI multi-voxel patterns associated with distinct states, 47 48 despite a lack of sensitivity to individual neurons (Logothetis, 2008; Chaimow et al., 2011). In 49 50 this way, MVPA provides a way to extend the population coding approach from systems 51 52 neuroscience to the macro-scale populations measured with fMRI (Haynes, 2015; Haxby et al., 53 2001; 2014; Lewis-Peacock & Norman, 2014; but see the Conclusions section for a discussion of 54 55 related limitations). 56 57 58 59 4 60 http://mc.manuscriptcentral.com/scan Page 5 of 32 Manuscripts submitted to Social Cognitive and Affective Neuroscience 1 2 3 In its original application, Haxby and colleagues (2001) used MVPA to demonstrate that 4 5 ventral temporal cortex shows spatially distributed response patterns to visual object categories 6 7 that can be discriminated from the region’s face response pattern using a classifier. Importantly, 8 this included the fusiform face area (FFA), a region of fusiform gyrus (FG) that reliably shows 9 10 higher mean activations for faces compared to other visual categories (Kanwisher, 1997). This 11 12 approach demonstrated that regions which show selectivity in univariate signal for one category 13 14 also can hold (perhaps even equivalent amounts of) information about other categories. Such a 15 classification (or “decoding”) analysis enables researchers to use neural response patterns to 16 17 predict an associated cognitive state or stimulus condition (Lewis-Peacock & Norman, 2014). A 18 19 classifier is typically trainedFor on one Peer set of the data Review and tested on at least one other held-out set. 20 21 By training a classifier to discriminate between any given experimental factors (i.e., conditions 22 or stimulus characteristics), testing the classifier on held-out data can reveal which regions are 23 24 involved in representing those conditions or characteristics of the stimuli. Another common way 25 26 to interpret the results of a classifier is that categorical boundaries between stimuli are 27 “computationally relevant” in a given brain region if that region shows high classification 28 29 accuracy for that category boundary. This interpretation assumes that if a brain region’s patterns 30 31 of activity discriminate between two stimulus categories, information about that category 32 33 boundary is retained in that region’s spatial response patterns because it is relevant for whatever 34 computation that region is performing in that particular cognitive context.
Details
-
File Typepdf
-
Upload Time-
-
Content LanguagesEnglish
-
Upload UserAnonymous/Not logged-in
-
File Pages33 Page
-
File Size-