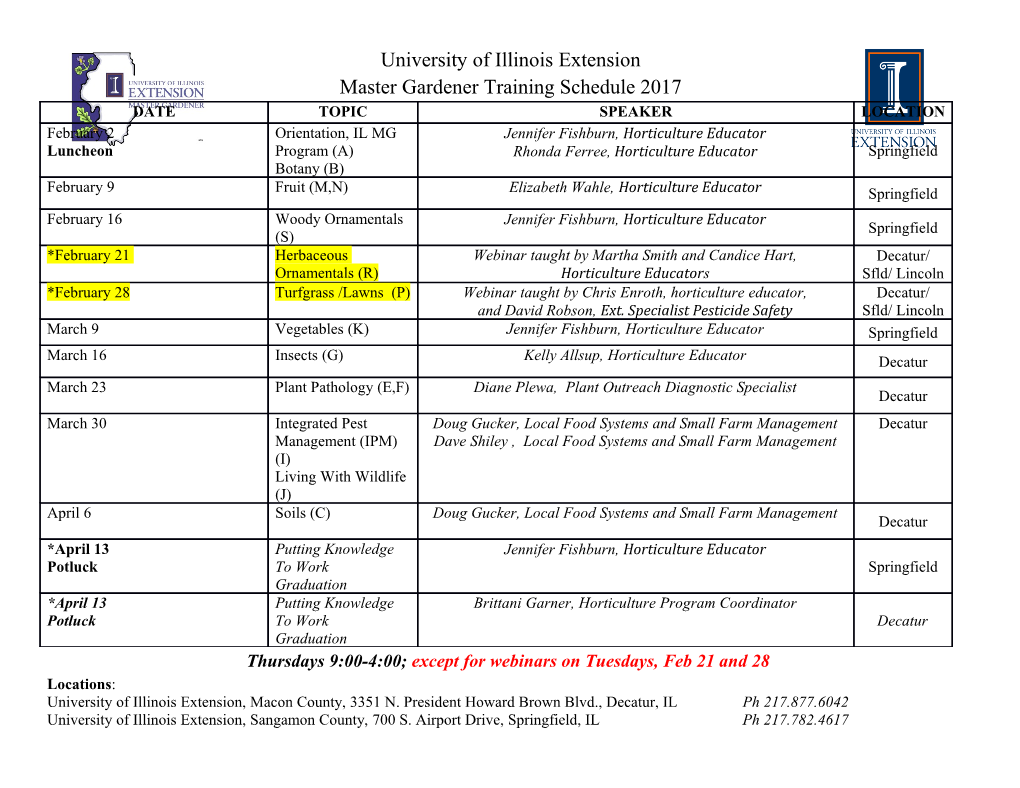
Dynamic Effects of Conceptual Combination on Semantic Network Structure Yoed N. Kenett ([email protected]) Sharon L. Thompson-Schill ([email protected]) Department of Psychology University of Pennsylvania, Philadelphia, PA 19104 USA Abstract where “mountain” is an object bearing a spatial relation with “lake”). An attributive based conceptual combination The generative capacity of language entails an ability to flexibly combine concepts with each other. Conceptual involves applying an attribute from the modifier noun to combination can occur either by using an attribute of one describe the head noun, such as zebra clam (“a clam that has concept to describe another (attributive combination) or by stripes”) A relational based conceptual combination, forming some relation between two concepts to create a new however, cannot be paraphrased this way - tennis ball is not one (relational combination). Prior research has addressed “a ball that is tennis”, but rather “a ball for playing tennis” whether common or distinct processes support these two (Downing, 1977; Gagné & Shoben, 1997). putatively different types of combinations. We turn the question around and ask whether the consequences of these An open theoretical issue is whether attributive and combination types on our conceptual system might differ, by relational mechanisms are similar or distinct; and if distinct, comparing semantic memory networks before and after how these mechanisms are applied (Estes, 2003; Gagné, participants perform either attributive or relational conceptual 2000; Gagné & Shoben, 1997; Rogers & McClelland, combinations. We find a general effect on the semantic 2004). We address this issue from a novel perspective: we networks: the structure of network decreases after participants apply a network science methodology to represent and conceptually combine some of the concepts in the network. compare semantic memory networks before and after However, the relational combination manipulation has a greater effect. Furthermore, only the relational combination participants conceptually combine some of the concepts in manipulation leads to an increase in the network’s the network with other concepts in either an attributive or a connectivity. relational manner. Such an approach allows us to examine, for the first time, in what way conceptual combinations Keywords: Conceptual combinations; Semantic Networks affect semantic memory structure, and how it differs based Introduction on attributive or relational mechanisms. We posit that such a conceptual combination manipulation will have Language generation involves the ability to combine restructuring effects on the semantic network, by changing concepts into novel combinations (Boylan, Trueswell, & or creating new connections between concepts in the Thompson-Schill, 2017). Investigating how individuals network. combine concepts can shed unique light on different aspects Recent studies have used computational network science of conceptual knowledge, including the cognitive to represent the structure of semantic memory (memory for mechanisms that enable the generative and flexible use of knowledge and facts, Jones, Willits, & Dennis, 2015), using language. For example, consider the noun-noun network science tools, as a semantic network and analyze its combination robin hawk: while some interpret this properties (for a review, see Borge-Holthoefer & Arenas, combination as “a red-breasted hawk”, applying the 2010). A semantic network comprises a set of nodes and attribute “red-breast” of the robin to the hawk; others edges, where nodes correspond to words or concepts and interpret this combination as “a hawk that preys on robins”, edges connect pairs of nodes and signify some sense of applying a thematic, relational role between robins and relations between the connected nodes. Of the various hawks (Wisniewski, 1996). While these two types of network models developed in network science theory, the conceptual combination mechanisms—attributive and network model that has been widely used to examine relational—are studied via behavioral and neurocognitive complex systems is the Small World Network (SWN) means (Boylan et al., 2017; Estes, 2003), whether these two model. A SWN is a network that is characterized by both mechanisms are similar or distinct remain an open question. high local connectivity and short global distances between Furthermore, the effect of these mechanisms on semantic nodes, allowing for efficient transfer of information. This memory structure has not yet been studied. In this paper, we network type is known as a small world network because apply a computational network science methodology to every node is relatively close to other nodes. Analyses of examine the effects of attributive and relational mechanisms different languages have consistently shown how different on semantic memory structure. Specifically, we will focus linguistic systems exhibit such SWN characteristics, on conceptual combinations in noun-noun compounds. characteristics which are now considered fundamental in Noun-noun compounds contain a modifier noun facilitating efficient and quick retrieval of linguistic followed by a head noun. The modifier noun can be either information (Borge-Holthoefer & Arenas, 2010). Common “attributive” (as in zebra clam, where zebra denotes the parameters of network structure include - the networks attribute “striped”) or “relational” (as in mountain lake, 657 clustering coefficient (CC), the average shortest path length Materials & Methods (ASPL), and the modularity index (Q). The CC measures the network’s connectivity. It refers to Participants the probability that two neighbors of a node will themselves Participants (N = 26) were recruited from the University of be neighbors (i.e., a neighbor is a node i that is connected Pennsylvania as part of a larger on-going research study on through an edge to node j). The ASPL and Q index measure conceptual combinations and semantic memory structure. the global structure of the network. The ASPL measure Participants were 55% female, average age of 22.6 years refers to the average shortest number of steps needed to be (SD = 3.9) and with an average 16.4 years of education (SD taken between any two pair of nodes. The Q measure = 3). Participants were randomly assigned to the attributive examines how a network breaks apart (or partitions) into combinations (AC) or relational combinations (RC) smaller sub-networks. The larger the modularity measure, conditions (N = 13 in each group). This study was approved the more the network comprised of sub-networks (Newman, by the University of Pennsylvania Institutional Review 2006). A SWN is characterized by having a high CC and a Board. short ASPL. To examine whether a specific network is a SWN, the statistical properties of empirical data are Design Overview compared to those of a random null network with the same number of nodes and edges. We characterized the semantic network of participants using Previous work has conducted such analysis to examine their free association responses obtained twice, before and cognitive phenomena such as language development, after completing a conceptual combination task that was bilingualism, memory search and retrieval, and creative biased (using both detailed instructions and a priming ability (Borge-Holthoefer & Arenas, 2010). For example, manipulation) to elicit either attributive or relational Kenett et al. (2014) found that low and high creative interpretations. With this procedure, we were able to assess individuals show different semantic network structure. The the main effect, within subjects, of conceptual combination semantic network of high creative individuals exhibited (by comparing the structure of the semantic networks at lower ASPL and Q values, and higher CC values compared both time points) as well as the interaction, between to that of the low creative individuals. This was the case subjects, of the type of conceptual combination on network despite both networks having an equal number of nodes, change. We also collected a number of measures of edges and average number of edges per node. Thus, cognitive ability that will be used in planned analyses of semantic networks analysis can be applied to examine individual differences in these effects. We will first describe differences in semantic memory structure related to different the conceptual combination task we used to manipulate the conditions such as attributive or relational combinations. type of combination process (attributive or relational). We Some current theories of semantic memory posit that will then describe the method we applied to represent the conceptual representations are not invariable across people semantic networks (before and after the conceptual or across time, but rather dynamically change contingent on combination task). context (e.g., task demand, stimulus modality) and individual differences (e.g., processing preferences), with Conceptual Combination Task short- and long-term effects on the structure of semantic Participants were presented with 25 noun-noun memory (Yee & Thompson-Schill, 2016). Such a dynamic combinations and were required to come up with an perspective describes an experienced-based, distributed, interpretation for each combination (Wisniewski & Love, semantic memory system that allows for flexible, generative 1998). They were also asked to indicate how familiar they language. We apply semantic network analysis to examine were with each combination and how
Details
-
File Typepdf
-
Upload Time-
-
Content LanguagesEnglish
-
Upload UserAnonymous/Not logged-in
-
File Pages6 Page
-
File Size-