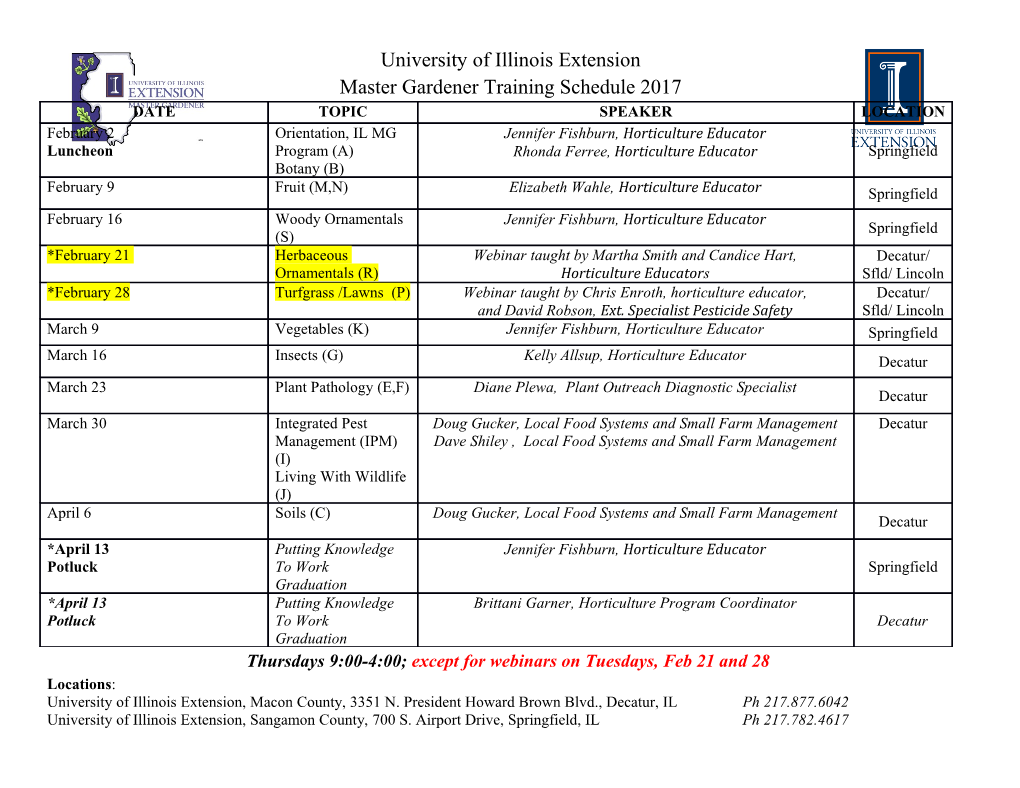
Truck Taxonomy and Classification Using Video and Weigh-In Motion (WIM) Technology Final Report Pan He, Aotian Wu, Anand Rangarajan, Sanjay Ranka <[email protected], [email protected], [email protected], [email protected]> Contract: BDV31-977-81 Date: May 2019 Truck Taxonomy and Classification Using Video and Weigh-In Motion (WIM) Technology Final Report DISCLAIMER The work was supported in part by the Florida Department of Transportation. The opinions, findings and conclusions expressed in this publication are those of the author(s) and not necessarily those of the Florida Department of Transportation or the U.S. Department of Transportation. ii Truck Taxonomy and Classification Using Video and Weigh-In Motion (WIM) Technology Final Report METRIC CONVERSION TABLE SYMBOL WHEN YOU KNOW MULTIPLY BY TO FIND SYMBOL in inches 25.4 millimeters mm ft. feet 0.305 meters m yd. yards 0.914 meters m mi miles 1.61 kilometers km Table 1: U.S. UNITS TO METRIC (SI) UNITS SYMBOL WHEN YOU KNOW MULTIPLY BY TO FIND SYMBOL mm millimeters 0.039 inches in m meters 3.28 feet ft. m meters 1.09 yards yd. km kilometers 0.621 miles mi Table 2: METRIC (SI) UNITS TO U.S. UNITS iii Truck Taxonomy and Classification Using Video and Weigh-In Motion (WIM) Technology Final Report DOCUMENTATION PAGE Technical Report Documentation Page 1. Report No. 2. Government Accession No. 3. Recipient’s Catalog No. 4. Title and Subtitle 5. Report Date Truck Taxonomy and Classification Using Video and Weigh-In Motion (WIM) Technology May 2019 Final Report 6. Performing Organization Code 7. Author(s) 8. Performing Organization Report No. Pan He, Aotian Wu, Anand Rangarajan, Sanjay Ranka 9. Performing Organization Name and Address, 10. Work Unit No. (TRAIS) Computer and Information Science and Engineering, University of Florida 432 Newell Dr, Gainesville, FL 32611 11. Contract or Grant No. BDV31-977-81 12. Sponsoring Agency Name and Address 13. Type of Report and Period Covered Florida Department of Transportation Final Report 605 Suwannee Street, MS 30 8/21/2017 - 7/22/2019 Tallahassee, FL 32399 14. Sponsoring Agency Code 15. Supplementary Notes 16. Abstract The primary objective of the present work was to develop video processing and machine learning methods to automatically detect and classify trucks traveling on Florida highways. The assembly of an automated system to detect, classify, and recognize various truck types from video and to derive their attributes is presented in this report. These extracted attributes were then used to determine commodity types, which can be used downstream for tracking commodity movements. To accomplish this, a set of high resolution videos (made available by the Florida Department of Transportation, FDOT) using freeway roadside passive cameras was utilized to create benchmark datasets. These videos were captured at different times of day, mainly at two freeway locations, and on various days during the past two years. The set of images derived from the videos was processed by our developed system to train and evaluate different approaches. The approaches drew upon recent work in deep convolutional neural networks for object detection and classification, semantic segmentation, and feature extraction, as well as drawing from traditional methods such as decision trees and geometric features (like edges and corners). We developed deep learning algorithms that leveraged transfer learning to determine whether an image frame has a truck and, if the answer is affirmative, localize the area from the image frame where the truck is most likely to be present. In particular, (1) we developed deep learning algorithms for detecting the location of a truck in a video frame followed by determining whether the image corresponds to a truck or a non-truck, (2) we developed a hybrid truck classification approach that integrates deep learning models and geometric truck features for classifying trucks into one of the nine FHWA classes (FHWA classes 5 through 13), (3) we developed algorithms for recognizing and classifying various truck attributes such as tractor type, trailer type, and refrigeration units that are useful in commodity prediction, and (4) we developed techniques for extracting vendor information corresponding to a truck, using logo and text detection. 17. Key Word 18. Distribution Statement Machine learning; Deep neural networks; Truck classification; Trailer classification; Logo detection; Commodity identification No restrictions. 19. Security Classif. (of this report) 20. Security Classif. (of this page) 21. No. of Pages 22. Price. Unclassified. Unclassified. 76 Form DOT F 1700.7 Reproduction of completed page authorized iv Truck Taxonomy and Classification Using Video and Weigh-In Motion (WIM) Technology Final Report ACKNOWLEDGMENTS The authors would like to thank the Florida Department of Transportation (FDOT) for provid- ing real truck video data and for insightful discussions during the course of the project. v Truck Taxonomy and Classification Using Video and Weigh-In Motion (WIM) Technology Final Report EXECUTIVE SUMMARY Freight analysis is an established approach in many states and major metropolitan areas. It is aimed at integrating various data sources, thus creating a comprehensive picture of freight movement. The main goal of the present work was to develop video processing and machine learning methods to automatically detect and classify trucks traveling on Florida highways. We used the Federal Highway Administration (FHWA) truck taxonomy for truck classification. Next, we automatically performed logo detection and recognition because this allowed for precise determination of commodity types in the detected truck. The assembly of an automated system for detection, classification, and recognition of var- ious truck types from video and deriving their attributes is addressed in this report. These extracted attributes were used to determine commodity types, which can be used down- stream for tracking commodity movements. To accomplish this, a set of high resolution videos (made available by FDOT) using freeway roadside passive cameras was utilized to create benchmark datasets. These videos were captured at different times of day, mainly at two freeway locations, and on various days during the past two years. The set of images derived from the videos was processed by our developed system to train and evaluate different approaches. The approaches drew upon recent work in deep neural networks for object detection and classification, semantic segmentation, feature extraction, as well as drawing from traditional methods such as decision trees and geometric features (like edges and corners). We developed deep learning algorithms that used transfer learning to determine whether an image frame has a truck and, if the answer is affirmative, localize the area from the image frame where the truck is most likely to be present. In particular, 1. We developed deep learning algorithms for detecting the location of a truck in a video frame followed by whether the image corresponds to a truck or a non-truck. 2. We developed a hybrid truck classification approach that integrates deep learning models and geometric truck features for classifying trucks into one of the nine FHWA classes (FHWA classes 5 through 13). 3. We developed algorithms for recognizing and classifying various truck attributes, such as tractor types, trailer types and refrigeration units, that are useful in commodity pre- vi Truck Taxonomy and Classification Using Video and Weigh-In Motion (WIM) Technology Final Report diction. 4. We developed techniques for extracting vendor information corresponding to a truck using logo and text detection. This consisted of two steps: determining the location of the text or logo and detecting company information using text- or logo-based identifi- cation. For enclosed trailer types (which correspond to a large fraction of trailer types), this is an effective way of determining the commodity. Results obtained from our datasets show that our scheme for truck classification has > 90% accuracy for classifying trucks into one of the nine classes and is relatively independent of the actual camera angle. Additionally, our algorithms for trailer detection achieved > 85% accuracy for classifying a tractor and trailer and a 95% accuracy for detecting a refrigerator unit. Additionally, we were able to demonstrate determination of vendor information using text- or logo-based detection and classification, which in turn is associated with commodities using the NAICS database. vii Truck Taxonomy and Classification Using Video and Weigh-In Motion (WIM) Technology Final Report CONTENTS Contents DISCLAIMER ii METRIC CONVERSION TABLE iii DOCUMENTATION PAGE iv ACKNOWLEDGMENTS v EXECUTIVE SUMMARY vi LIST OF FIGURES x LIST OF TABLES xiv 1 Introduction 1 2 Literature Review 3 3 Methodology 6 3.1 Overview . 6 3.2 Truck Model . 8 3.2.1 Truck Detection Component . 8 3.2.2 Truck Classification Component . 10 3.2.3 Results for Truck Classification . 19 3.2.4 Tractor and Trailer Classification Component . 21 3.2.5 Results for Tractor and Trailer Classification . 24 3.2.6 Refrigerator Unit Detection Component . 27 3.3 Commodity Classification . 28 3.3.1 Text-based Logo Detection and Recognition . 29 3.3.2 Image-based Logo Detection and Recognition . 32 4 Conclusions 44 5 References 47 Appendices 51 viii Truck Taxonomy and Classification Using Video and Weigh-In Motion (WIM) Technology Final Report CONTENTS A Machine Learning Terminology and Acronyms 51 B Visualization and Annotation Tool Development 55 C FHWA Vehicle Classification 56 D Refrigerator Unit Model 57 E Wheel Model 58 F FDOT Image Library 60 G Universal Logo Detector 61 ix Truck Taxonomy and Classification Using Video and Weigh-In Motion (WIM) Technology Final Report LIST OF FIGURES List of Figures 1 A pipeline of all the developed techniques. 6 2 The YOLO detector divides the image into an S × S grid and, using shared computation, passes the whole image through the model to obtain image fea- tures for each cell. Predictions are encoded as a 3D tensor of size S × S × (B × 5 + C).
Details
-
File Typepdf
-
Upload Time-
-
Content LanguagesEnglish
-
Upload UserAnonymous/Not logged-in
-
File Pages76 Page
-
File Size-