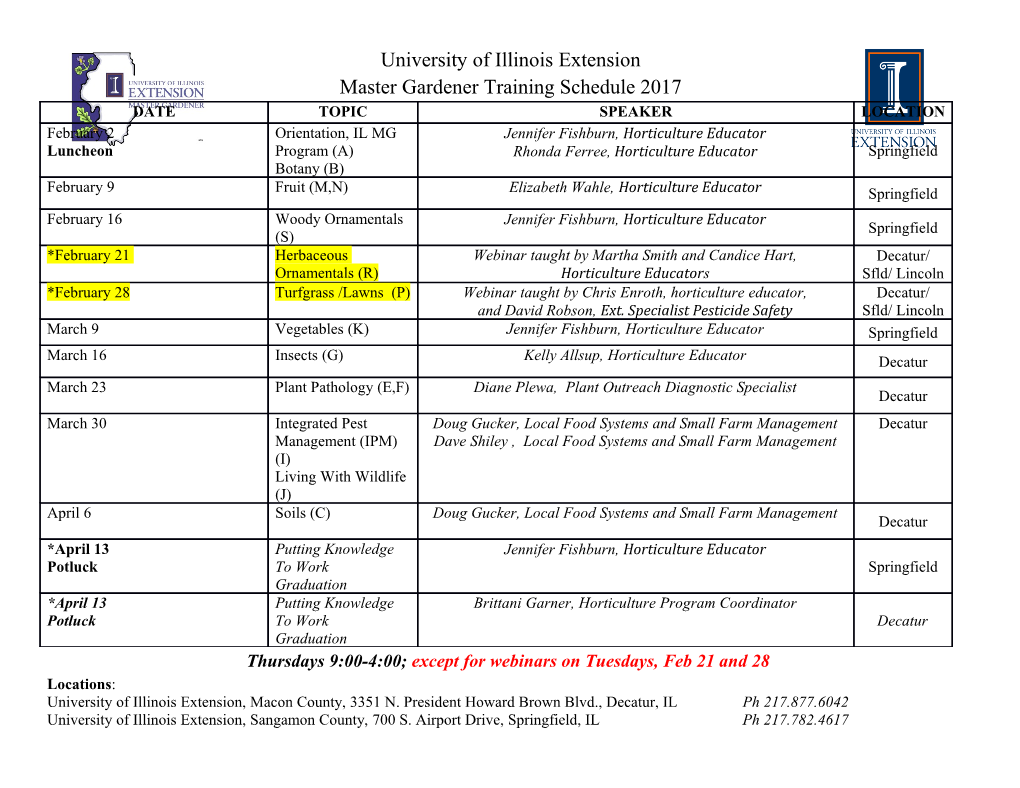
QR Decomposition on GPUs Andrew Kerr, Dan Campbell, Mark Richards Georgia Institute of Technology, Georgia Tech Research Institute {andrew.kerr, dan.campbell}@gtri.gatech.edu, [email protected] ABSTRACT LU, and QR decomposition, however, typically require ¯ne- QR decomposition is a computationally intensive linear al- grain synchronization between processors and contain short gebra operation that factors a matrix A into the product of serial routines as well as massively parallel operations. Achiev- a unitary matrix Q and upper triangular matrix R. Adap- ing good utilization on a GPU requires a careful implementa- tive systems commonly employ QR decomposition to solve tion informed by detailed understanding of the performance overdetermined least squares problems. Performance of QR characteristics of the underlying architecture. decomposition is typically the crucial factor limiting problem sizes. In this paper, we focus on QR decomposition in particular and discuss the suitability of several algorithms for imple- Graphics Processing Units (GPUs) are high-performance pro- mentation on GPUs. Then, we provide a detailed discussion cessors capable of executing hundreds of floating point oper- and analysis of how blocked Householder reflections may be ations in parallel. As commodity accelerators for 3D graph- used to implement QR on CUDA-compatible GPUs sup- ics, GPUs o®er tremendous computational performance at ported by performance measurements. Our real-valued QR relatively low costs. While GPUs are favorable to applica- implementation achieves more than 10x speedup over the tions with much inherent parallelism requiring coarse-grain native QR algorithm in MATLAB and over 4x speedup be- synchronization between processors, methods for e±ciently yond the Intel Math Kernel Library executing on a multi- utilizing GPUs for algorithms computing QR decomposition core CPU, all in single-precision floating-point. We present remain elusive. throughput for real-valued implementations of this QR im- plementation executing on several GPU architectures and In this paper1, we discuss the architectural characteristics believe this is the fastest strictly GPU implementation yet of GPUs and explain how a high-performance implementa- presented. Our implementation performs all processing on tion of QR decomposition may be implemented. We provide the selected GPU, enabling threads on the host processor detailed performance analysis of the resulting implementa- to perform other computations and to devote PCI-Express tion for real-valued matrices and o®er recommendations for bandwidth to other tasks. achieving high performance to future developers of dense lin- ear algebra procedures for GPUs. Our implementation sus- tains 143 GFLOP/s, and we believe this is the fastest an- 2. BACKGROUND nounced QR implementation executing entirely on the GPU. Commodity GPUs are inexpensive resources for delivering very high computing throughput for certain classes of ap- plications. GPUs are sold primarily as an integrated com- 1. INTRODUCTION ponent in display adapters for desktop personal computers. Graphics Processing Units are massively parallel processors High-throughput GPUs are primarily aimed for the video capable of completing hundreds of floating point operations game market. The primary application of GPUs has a very in parallel. Their large register ¯les and high-performance large degree of potential parallelism at the most computa- scratchpad memories are well-suited to streaming execution tionally intensive step: applying ¯nal shading e®ects to each models. Matrix factorization algorithms such as Cholesky, pixel in a polygon as it is rendered into the display bu®er. This fact has allowed GPU vendors to exploit microarchi- 1This work was supported in part by DARPA and AFRL under contracts FA8750-06-1-0012 and FA8650-07-C-7724. tecture parallelism for increased performance without con- The opinions expressed are those of the authors. straint by the application and without requiring much archi- tectural infrastructure to facilitate parallel execution. The volume of the GPU market provides tremendous competi- tive pressure to improve performance and keep prices low Permission to make digital or hard copies of all or part of this work for over successive product generations. personal or classroom use is granted without fee provided that copies are not made or distributed for profit or commercial advantage and that copies Simultaneously, GPU execution models have grown in flex- bear this notice and the full citation on the first page. To copy otherwise, to ibility in response to the needs of graphics programmers republish, to post on servers or to redistribute to lists, requires prior specific thereby enabling a wide range of computing tasks. GPU permission and/or a fee. GPGPU '09, March 8, 2009, Washington, D.C., USA. vendors have consequently developed graphics-agnostic pro- Copyright 2009 ACM 978-1-60558-517-8/09/03 ... $5.00 gramming models such as NVIDIA's Compute Uni¯ed De- vice Architecture (CUDA) [1] and Open Compute Layer · ¸ · ¸ · ¸ · ¸ (OpenCL) [2] to facilitate general purpose computing on f c s f r G(θ) = = GPUs. Nevertheless, fully exploiting the peak performance g ¡s¤ c g 0 capacity of GPUs has remained a challenge. Algorithms with very high arithmetic intensity, very little need to syn- chronize between execution paths, and very few scatter oper- The algorithm begins by choosing elements from the bottom ations typically perform well on GPUs without the need for two rows in A in the left-most column. These determine the careful optimization, but many computing tasks do not ¯t the Givens rotation Gm;1(θ) which may then be used to these idealized constraints. Several algorithms for fast QR multiply in-place the submatrix of A formed by the bottom H decomposition exhibit a high degree of parallelism, but have two rows. Then, Gm;1(θ) may be used to multiply a pair of low arithmetic intensity and are highly coupled between ex- columns Q(:; m¡1 : m) in place. The multiplication in A has ecution paths, requiring synchronization between elements the desired e®ect of overwriting the bottom left element with after small numbers of arithmetic operations. As a result, 0 while preserving the invariants in the algorithm. Next, the attempts to exploit GPUs to accelerate QR decomposition algorithm proceeds up one row in A and repeats, computing have been moderately successful achieving 4x speedup [3] Gm¡1;1(θ) and applying it to A(m ¡ 2 : m ¡ 1; :) and Q(: over a reference implementation distributed with Lincoln' ; m¡2 : m¡1). This proceeds throughout the entire column Laboratory's HPEC Challenge [4]. In this paper, we base stopping at the main diagonal at which point all of the left speedup numbers on the highly optimized Intel Math Ker- column in A except the ¯rst element is overwritten with nel Library which is among the fastest QR implementations zeros. The algorithm moves right one column and repeats available for multicore CPUs. from the bottom row, again stopping at the main diagonal. When all columns have been visited, A is in upper triangular form, and the QR decomposition is complete. 3. QR ALGORITHMS QR decomposition factors an m-by-n matrix A into the G(θ) may be computed from f and g without explicitly com- product A = QR, where Q is an m-by-m unitary matrix puting θ or evaluating any trigonometic functions [7]. More- and R is an m-by-n upper triangular matrix. Several one- over, Sameh and Kuck [8] demonstrate a pattern in which sided factorization methods compute the QR decomposition. Givens rotations may be computed in parallel. This method Two of these, Givens and Householder [5], apply a set of or- is adaptable to streaming architectures and systolic arrays thogonal transformations to the input matrix to bring it into such as MIT RAW [9]. In general, this approach achieves upper triangular form. By concatenating these orthogonal good performance on MIMD architectures that support low- transforms, the matrix Q is formed, all while preserving the latency communication and synchronization. GPUs, on the H invariants A = QR and Q Q = I. Modi¯ed Gram-Schmidt other hand, do not o®er on-chip mechanisms for synchroniz- computes the QR factorization via projection operations. ing the several SIMD processors and require either invoking Each of these methods has favorable numerical properties a sequence of kernels with implicit synchronization barriers and o®ers some parallelism. The suitability of each algo- between each invocation or assuming a consistency model rithm for GPU implementation depends on memory access for global memory and implementing barriers in the shared patterns, the frequency of inter-processor synchronization, global address space. Performance results for Givens QR and the scalability of parallelism within the algorithm. implemented on CUDA-compatible GPUs have been pre- sented for the HPEC Challenge benchmarks [3]. However, attempts to develop a high performance implementation of 3.1 Modified Gram-Schmidt this algorithm in CUDA were met with limited success and The modi¯ed Gram-Schmidt QR (MGS) method computes do not scale well to arbitrarily large matrix sizes. A = Q1R1 where A is m-by-n, Q1 is m-by-n with orthonor- mal columns, and R1 is n-by-n. This factorization di®ers from what we have de¯ned in that Q is not square. MGS of- 3.3 Householder QR fers fairly good numerical properties, but it consists of oper- Householder QR computes the upper triangular matrix R ations that are not favorable to high performance on GPUs: from an m-by-n matrix A by applying a sequence of House- computing each element of the output matrix R requires holder reflections to A in place [5]. A Householder reflection large vector inner products and many synchronizations [5]. is an orthogonal transform of the form Implementing MGS on a GPU would incur prohibitive over- head. A blocked method exists in which the matrix to be factored is ¯rst partitioned into a number of su±ciently 2 P = I ¡ vvH small submatrices which are independently factored.
Details
-
File Typepdf
-
Upload Time-
-
Content LanguagesEnglish
-
Upload UserAnonymous/Not logged-in
-
File Pages8 Page
-
File Size-